Loss Function Dynamics and Landscape for Deep Neural Networks Trained with Quadratic Loss
DOKLADY MATHEMATICS(2023)
摘要
Knowledge of the loss landscape geometry makes it possible to successfully explain the behavior of neural networks, the dynamics of their training, and the relationship between resulting solutions and hyperparameters, such as the regularization method, neural network architecture, or learning rate schedule. In this paper, the dynamics of learning and the surface of the standard cross-entropy loss function and the currently popular mean squared error (MSE) loss function for scale-invariant networks with normalization are studied. Symmetries are eliminated via the transition to optimization on a sphere. As a result, three learning phases with fundamentally different properties are revealed depending on the learning step on the sphere, namely, convergence phase, phase of chaotic equilibrium, and phase of destabilized learning. These phases are observed for both loss functions, but larger networks and longer learning for the transition to the convergence phase are required in the case of MSE loss.
更多查看译文
关键词
scale invariance,batch normalization,training of neural networks,optimization,MSE loss function
AI 理解论文
溯源树
样例
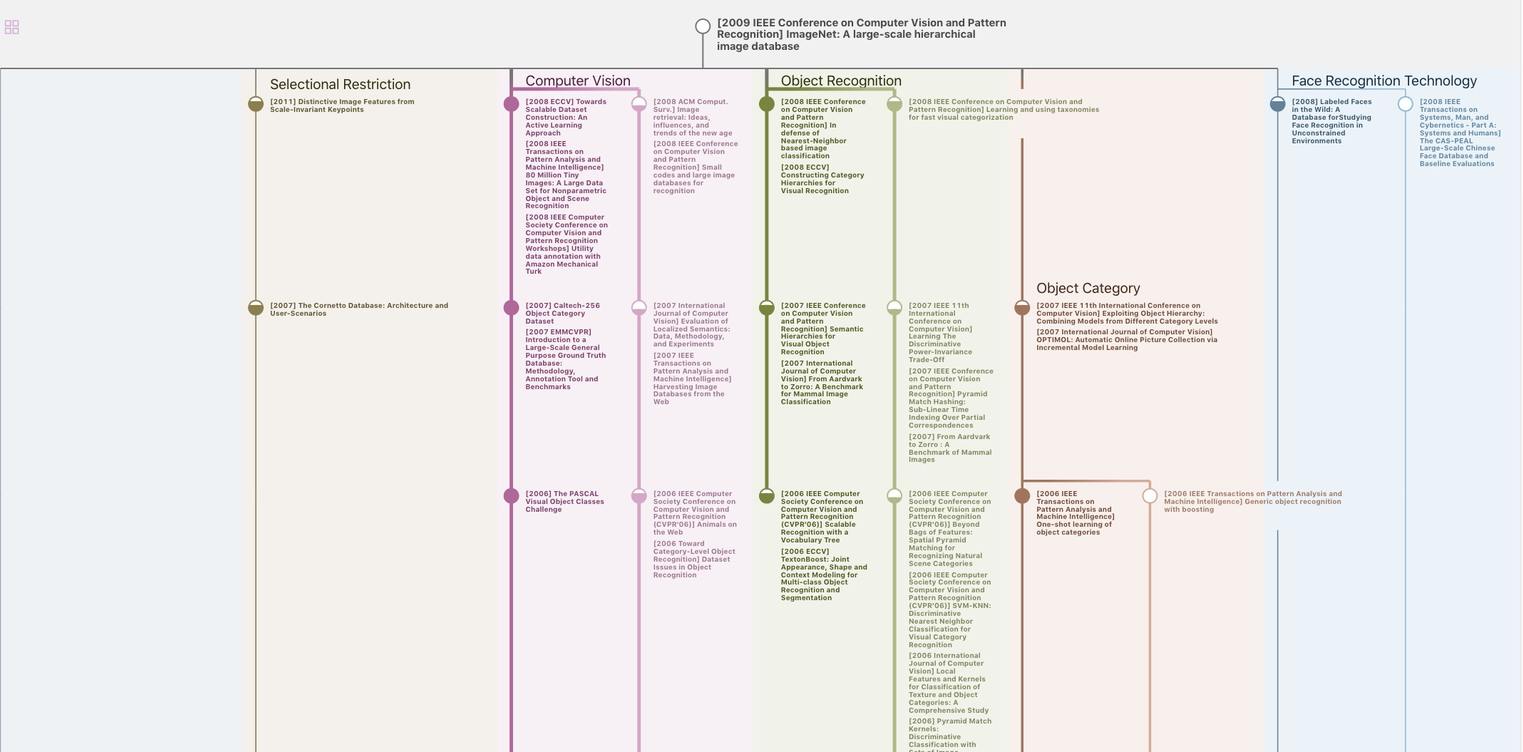
生成溯源树,研究论文发展脉络
Chat Paper
正在生成论文摘要