Digital Twin for Transportation Big Data: A Reinforcement Learning-Based Network Traffic Prediction Approach
IEEE TRANSACTIONS ON INTELLIGENT TRANSPORTATION SYSTEMS(2024)
摘要
Vehicular Ad-Hoc Networks (VANETs), as the crucial support of Intelligent Transportation Systems (ITS), have received great attention in recent years. With the rapid development of VANETs, various services have generated a great deal of data that can be used for transportation planning and safe driving. Especially, with the advent of Coronavirus Disease 2019 (COVID-19), the transportation system has been impacted, thus novel modes of transportation planning and intelligent applications are necessary. Digital twins can provide powerful support for artificial intelligence applications in Transportation Big Data (TBD). The features of VANETs are varying, which arises the main challenge of digital twins applying in TBD. Network traffic prediction, as part of digital twins, is useful for network management and security in VANETs, such as network planning and anomaly detection. This paper proposes a network traffic prediction algorithm aiming at time-varying traffic flows with a large number of fluctuations. This algorithm combines Deep Q-Learning (DQN) and Generative Adversarial Networks (GAN) for network traffic feature extraction. DQN is leveraged to carry out network traffic prediction, in which GAN is involved to represent Q-network. Meanwhile, the generative network can increase the number of samples to improve the prediction error. We evaluate the performance of our method by implementing it on three real network traffic data sets. Finally, we compare the two state-of-the-art competing methods with our method.
更多查看译文
关键词
COVID-19,Predictive models,Neural networks,Feature extraction,Transportation,Generative adversarial networks,Digital twins,Digital twin,transportation big data,deep reinforcement learning,generative adversarial networks
AI 理解论文
溯源树
样例
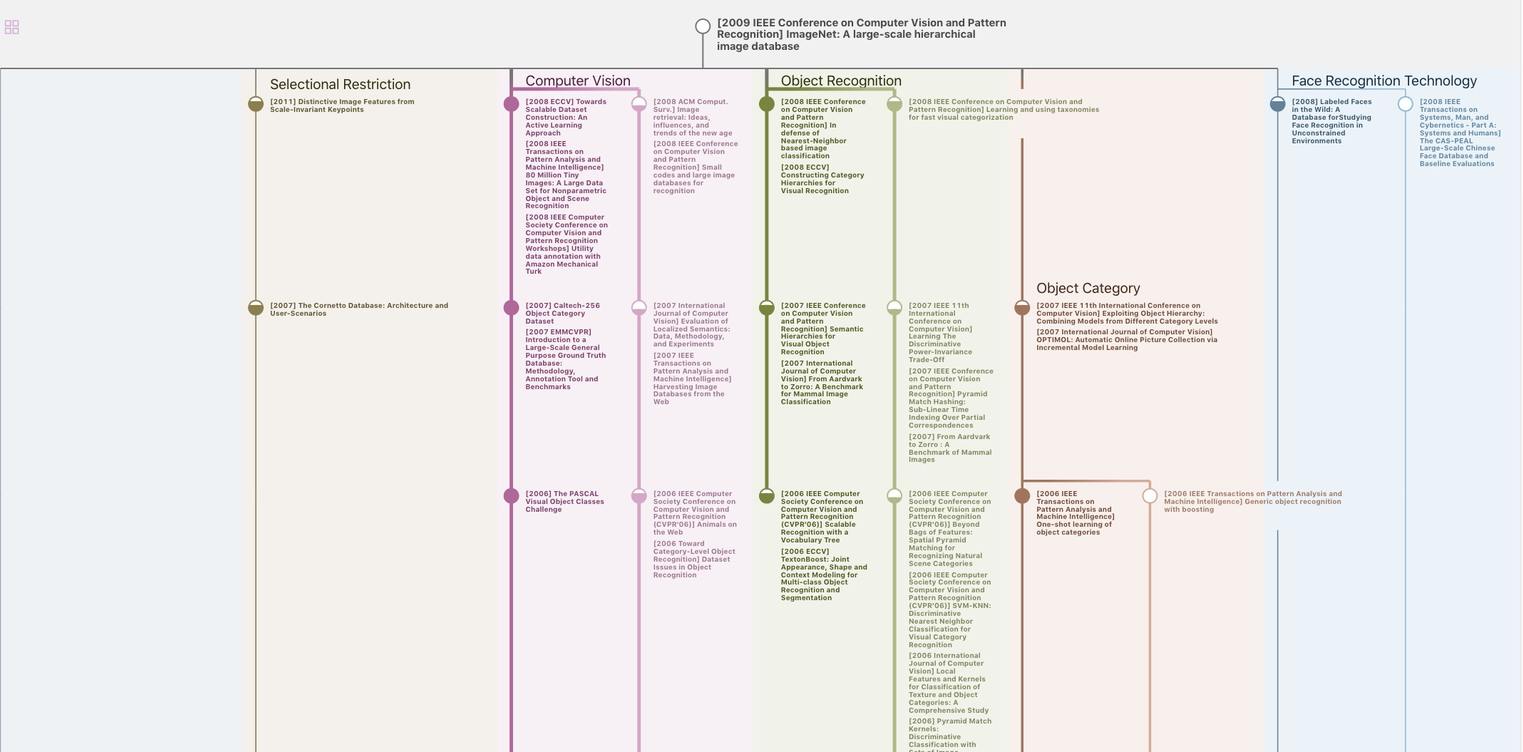
生成溯源树,研究论文发展脉络
Chat Paper
正在生成论文摘要