In Vivo Performance of a Machine Learning Model Predicting Pediatric Sepsis at Triage
PEDIATRIC QUALITY & SAFETY(2023)
摘要
Introduction: Sepsis is a leading cause of pediatric mortality across the globe. Early recognition reduces mortality; and improve outcomes however, rapid detection remains difficult. Although physiologic mechanisms contribute to the complexity the epidemiology of pediatric Emergency Department (ED) visits also contributes. A variety of sepsis screening methods have been designed, from alerts based on triage temperature and heart rate to more complex algorithms. Evaluation of such tools has suggested improved identification, yet there is a lack of definitive data.Objective: The objective of this study was to evaluate the implementation and performance of the (ST2)-T-2 (Sepsis Screening Triage Tool) in a pediatric ED.Methods: The (ST2)-T-2 is a multiclass stochastic gradient boosting machine learning model recently developed at the study institution. It uses ED triage data and historical data mined from electronic health record to predict sepsis potential at triage. (ST2)-T-2 was operationalized on 2/1/21; performance data for this study were collected over the first 7 months of implementation (to 8/31/21). Sensitivity and specificity were assessed by comparison with final International Classification of Disease-10 diagnoses codes. The pediatric ED at which this model was developed is a level-2 pediatric trauma center with an annual census of approximately 96,000.Results: In total, 43,337 patients were included, 41 with severe sepsis and 121 with sepsis. (ST2)-T-2 demonstrated a sensitivity of 41.5% and specificity of 99.2% for severe sepsis, and a sensitivity of 25.6% and specificity of 99.2% for sepsis.Conclusion: (ST2)-T-2 may aide in the identification of pediatric patients at risk for sepsis, potentially allowing for accelerated diagnosis, intervention, improved care, and better outcomes.
更多查看译文
关键词
machine learning,learning model
AI 理解论文
溯源树
样例
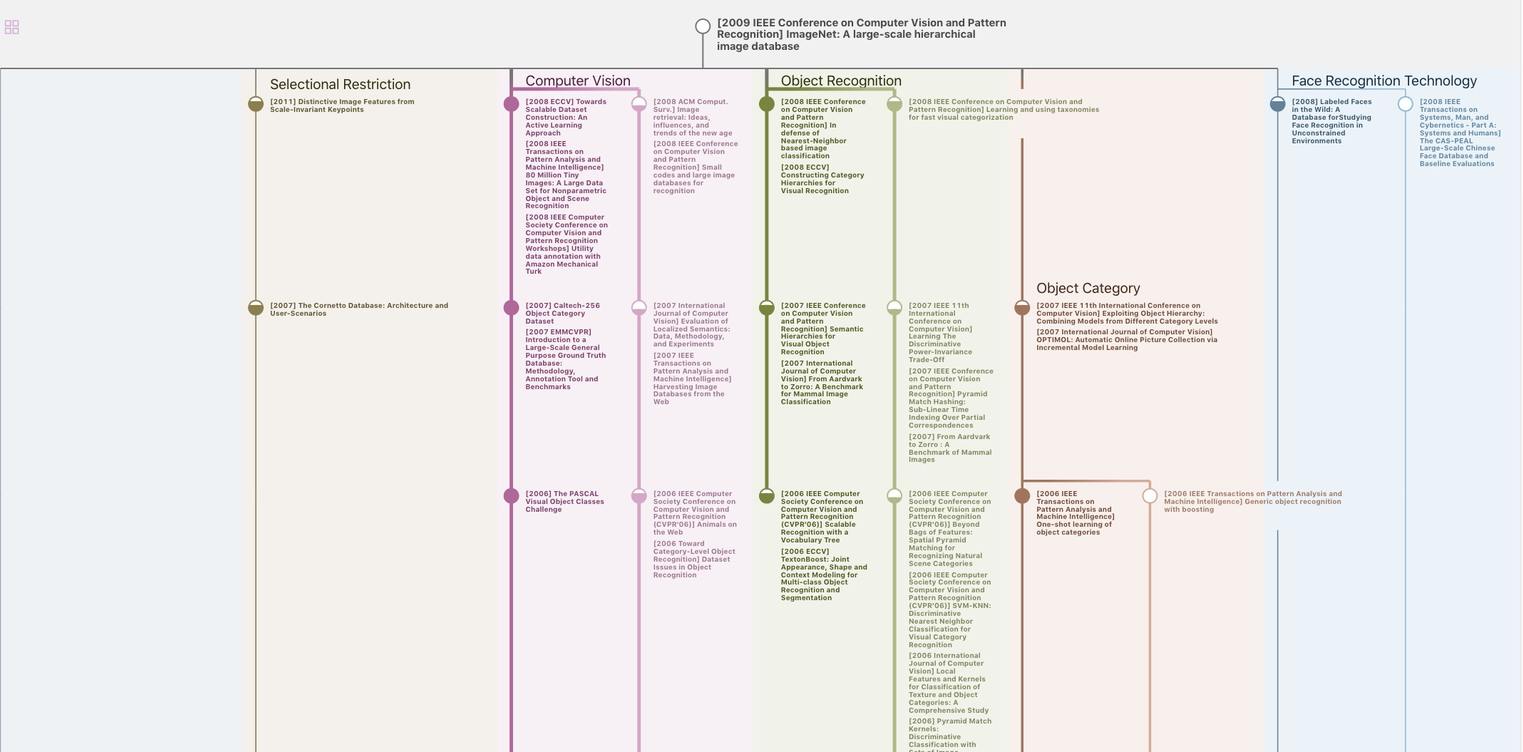
生成溯源树,研究论文发展脉络
Chat Paper
正在生成论文摘要