Beyond Homophily and Homogeneity Assumption: Relation-Based Frequency Adaptive Graph Neural Networks
IEEE TRANSACTIONS ON NEURAL NETWORKS AND LEARNING SYSTEMS(2023)
摘要
Graph neural networks (GNNs) have been playing important roles in various graph-related tasks. However, most existing GNNs are based on the assumption of homophily, so they cannot be directly generalized to heterophily settings where connected nodes may have different features and class labels. Moreover, real-world graphs often arise from highly entangled latent factors, but the existing GNNs tend to ignore this and simply denote the heterogeneous relations between nodes as binary-valued homogeneous edges. In this article, we propose a novel relation-based frequency adaptive GNN (RFA-GNN) to handle both heterophily and heterogeneity in a unified framework. RFA-GNN first decomposes an input graph into multiple relation graphs, each representing a latent relation. More importantly, we provide detailed theoretical analysis from the perspective of spectral signal processing. Based on this, we propose a relation-based frequency adaptive mechanism that adaptively picks up signals of different frequencies in each corresponding relation space in the message-passing process. Extensive experiments on synthetic and real-world datasets show qualitatively and quantitatively that RFA-GNN yields truly encouraging results for both the heterophily and heterogeneity settings. Codes are publicly available at: https://github.com/LirongWu/RFA-GNN.
更多查看译文
关键词
Task analysis,Graph neural networks,Feature extraction,Adaptation models,Transforms,Kernel,Electronic mail,Graph neural networks (GNNs),heterogeneous graph,heterophily graph,relation learning
AI 理解论文
溯源树
样例
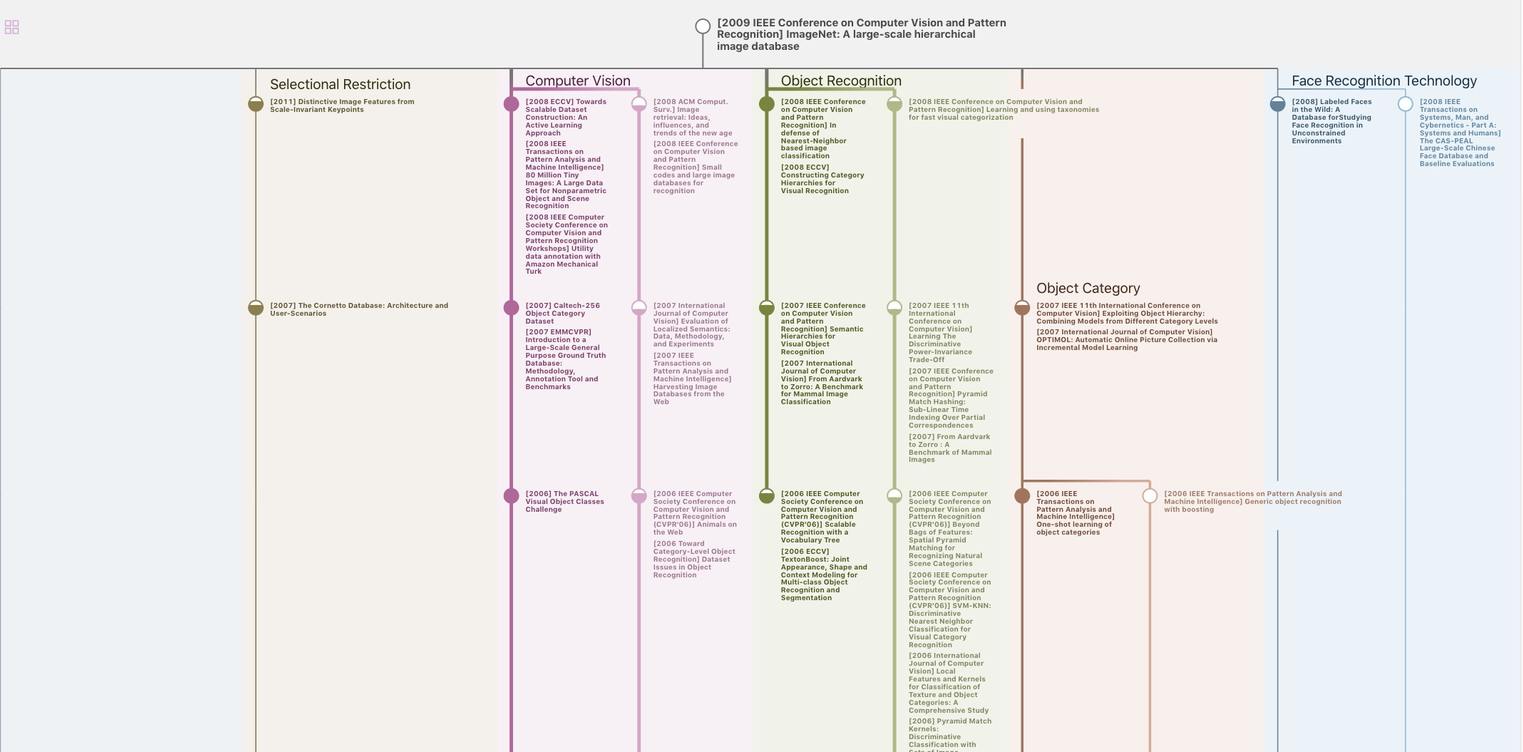
生成溯源树,研究论文发展脉络
Chat Paper
正在生成论文摘要