Deep Learning Reduction to the Pole Method Constrained by Initial Model for Low Latitude Magnetic Anomalies
CHINESE JOURNAL OF GEOPHYSICS-CHINESE EDITION(2023)
关键词
Low-latitude magnetic anomaly,Initial model,Deep learning,Reduction-to-the-pole,Joint drive
AI 理解论文
溯源树
样例
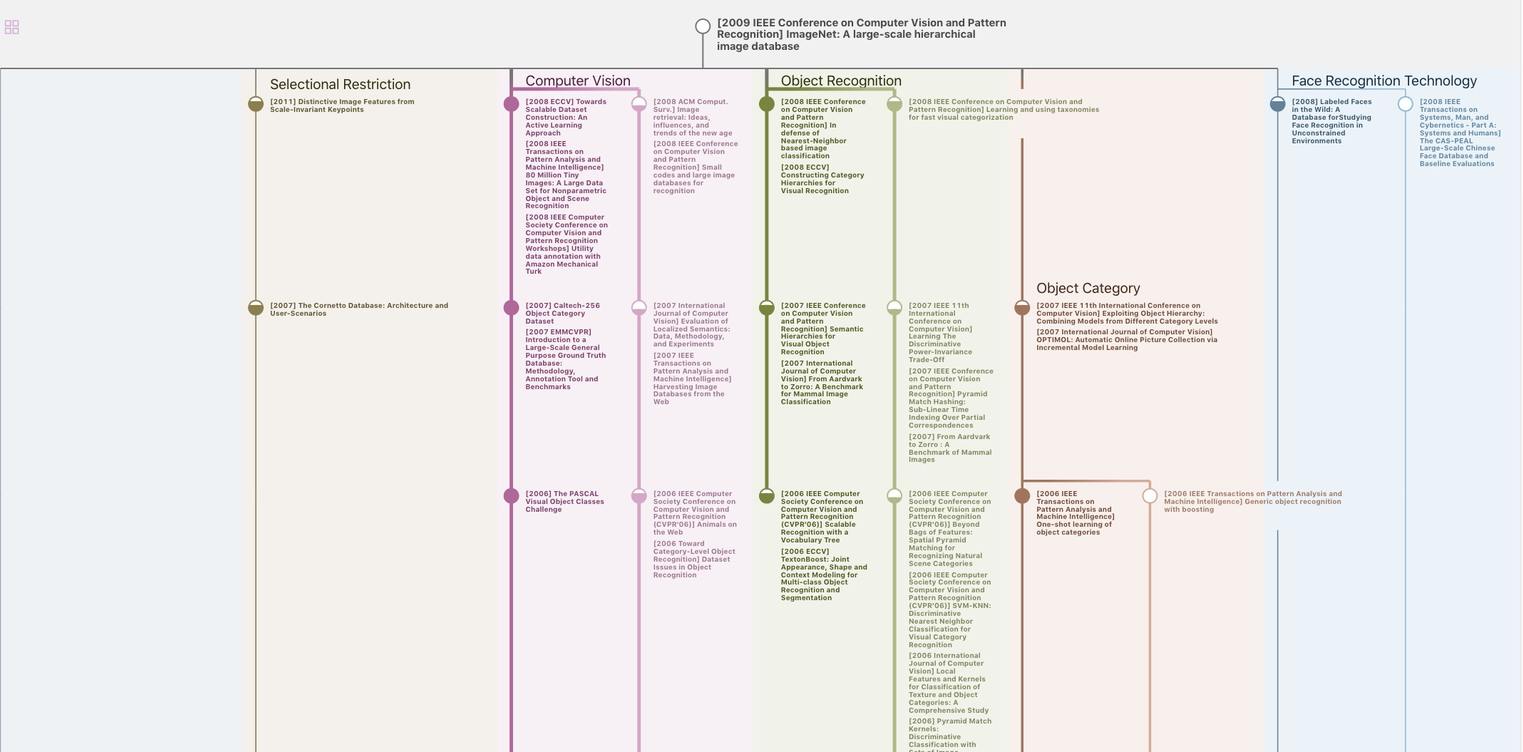
生成溯源树,研究论文发展脉络
Chat Paper
正在生成论文摘要