Machine learning model performances for the Z boson mass
EUROPEAN PHYSICAL JOURNAL PLUS(2023)
摘要
Z bosons, one of the electroweak gauge bosons, are produced in proton–proton (pp) collisions mainly by Drell-Yan (DY) process. Having short lifetime results in study of the vector boson production via reconstruction of its decay products. Because of its remarkable success in various disciplines, in this study machine learning (ML) models were applied to identify the invariant mass spectrum of Z boson produced in pp collisions at √(s) = 7 TeV at the Large Hadron Collider (LHC) by reconstruction of two oppositely charged same-flavor leptons, muons or electrons, in its peak region (60–120 GeV/c ^2 ). To introduce an alternative method for classic vector boson analysis, ensemble models such as Random Forest (RF), Weighted Random Forest (WRF), Balanced Random Forest (BRF), a gradient boosting framework as Light Gradient Boosted Machine (LightGBM), and Deep Neural Networks (DNNs) were preferred for identification of Z boson spectrum from its dielectron and dimuon decay channels, separately. Each model's performances were assessed by ML metrics such as area under receiver operating characteristic curve (AUC ROC), sensitivity, precision, and F-1 score. It is revealed that LightGBM algorithm has 99.311
更多查看译文
关键词
model performances,machine learning,mass
AI 理解论文
溯源树
样例
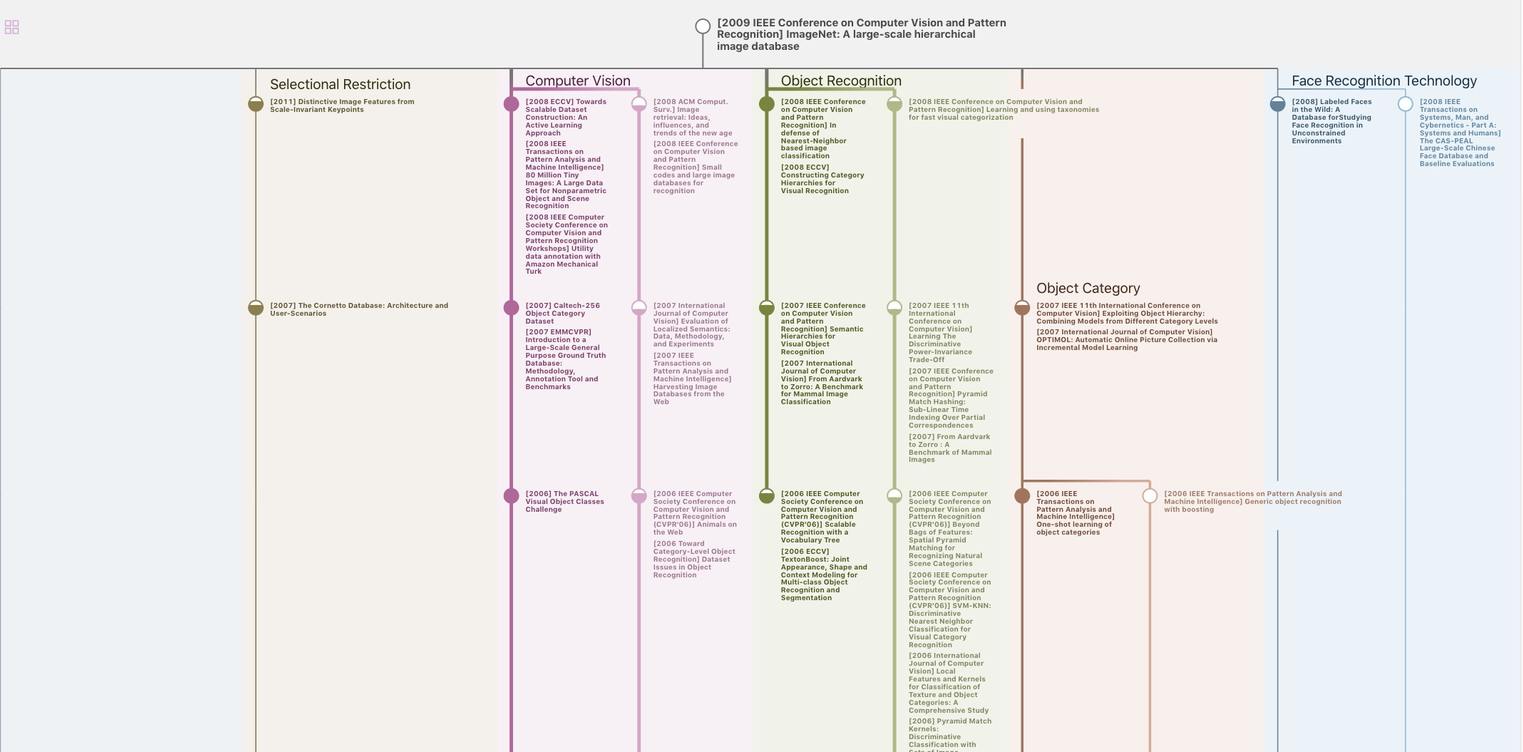
生成溯源树,研究论文发展脉络
Chat Paper
正在生成论文摘要