Identification of Supersonic Inlet Unstarted State and Optimal Sensor Configuration Based on EI-SPEA2
JOURNAL OF AEROSPACE ENGINEERING(2023)
摘要
As the key starting component of supersonic flight, identification of the unstarted state of the supersonic inlet is the premise of restarting the inlet and an important link of engine protection control. In order to explore the characteristics of the dynamic pressure inside the inlet, the selection of a pressure sensor configuration scheme, that is, how to obtain more abundant pressure information with fewer sensors, is also an urgent problem to be solved. This paper combines time-frequency analysis and deep learning for flow pattern recognition of a supersonic inlet and proposes a strength-based Pareto evolutionary algorithm combined with improved extinction and migration strategies (EI-SPEA2). The signals of all sensors were preliminarily processed using continuous wavelet transform, and the dynamic signals of all sensors were transformed into two-dimensional (2D) time-frequency spectrograms, which were then used as inputs by convolutional neural network to determine the inlet flow patterns from the dynamic sensor signals. A multiobjective optimization algorithm, EI-SPEA2, was adopted, which takes the minimum classification error, minimum number of selected sensors, and optimal sensor distribution as the optimization objectives. Through the continuous iteration of the population, identification of the unstarted state and optimal configuration of sensors was carried out simultaneously. By introducing the improved EI strategy at the end of iteration, SPEA2 can get rid of the local optimum and achieve convergence faster. A distance index for measuring sensor distribution and two indexes for measuring sensor information differentiation are proposed to improve sensor configuration scheme. The experimental results demonstrated that compared with competitive methods, EI-SPEA2 has better performance on both dual-objective optimization problems and three-objective optimization problems. Moreover, three indexes proposed to measure the distribution of the sensor are beneficial to improving the sensor configuration scheme, and the distance-based index is more suitable.
更多查看译文
关键词
Identification of unstarted state,Time-frequency analysis,Convolutional neural network,Multiobjective evolutionary algorithm,Optimal sensor configuration
AI 理解论文
溯源树
样例
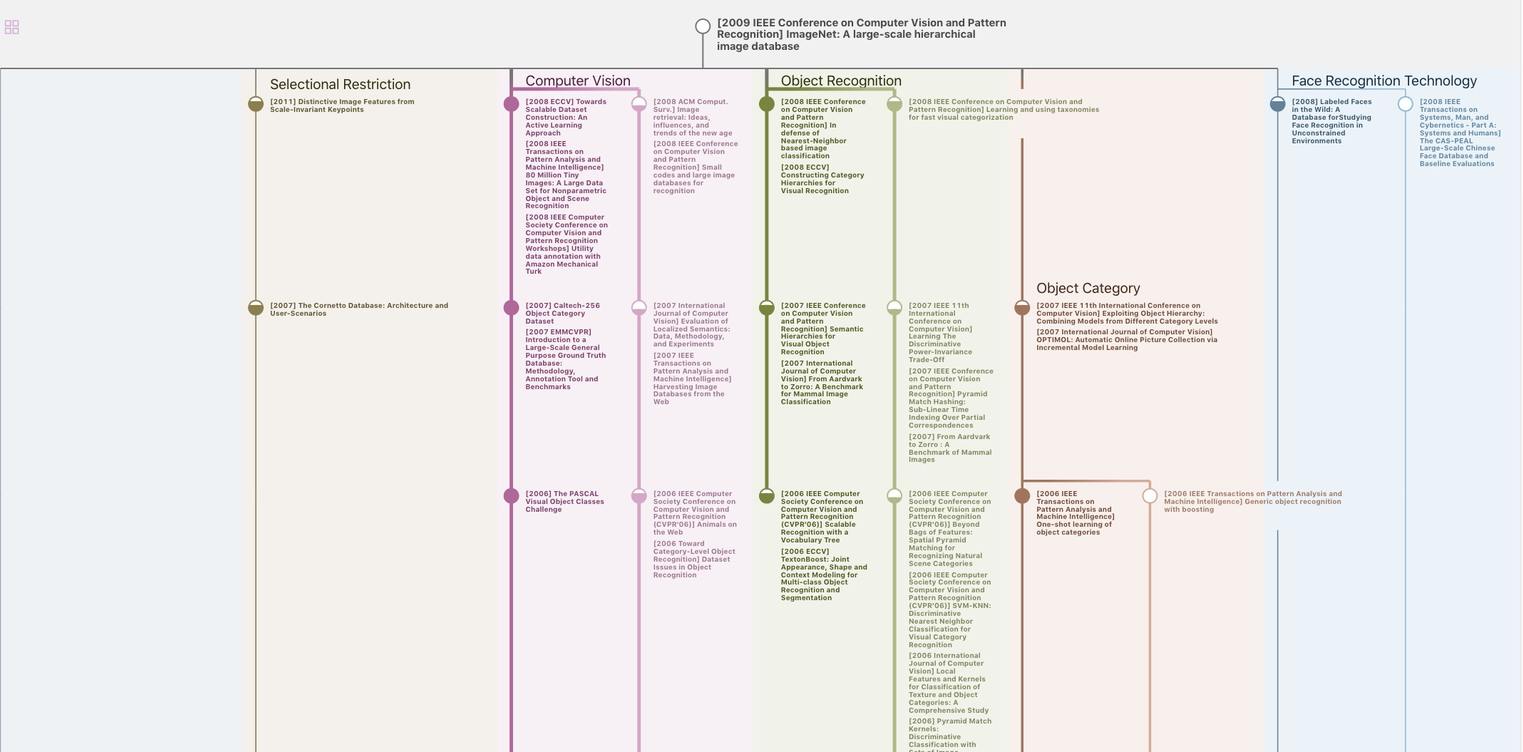
生成溯源树,研究论文发展脉络
Chat Paper
正在生成论文摘要