A deep learning approach using temporal-spatial data of computational fluid dynamics for fast property prediction of gas-solid fluidized bed
KOREAN JOURNAL OF CHEMICAL ENGINEERING(2023)
摘要
To deal with the critical issue of long computational time in practical application of computational fluid dynamics (CFD), this paper presents a new approach of deep learning for voidage prediction (DeepVP) that couples short time CFD simulations (limited CFD iterations) with the deep learning method to accelerate the 2D voidage distribution prediction for a gas-solid fluidized bed at steady state. Short time CFD simulations are first performed to obtain a sequence of voidage distribution images containing the temporal-spatial property of a gas-solid fluidized bed of the early period. A deep learning model is built to predict the voidage distribution at steady state, which is achieved by implementing multi-scale convolutional neural networks based on the sequence of voidage images. The case study results for a bubbling bed show that the voidage distribution at steady state for the bubbling bed can be predicted with comparable accuracy of conventional CFD simulations at about 1/30th computational cost. Moreover, the DeepVP method exhibits better extrapolation capability than the deep learning approach merely based on CFD condition parameters.
更多查看译文
关键词
Gas-solid Fluidized Bed,CFD Surrogate Model,Deep Learning,Multi-scale Convolutional Neural Network,Voidage Distribution Prediction
AI 理解论文
溯源树
样例
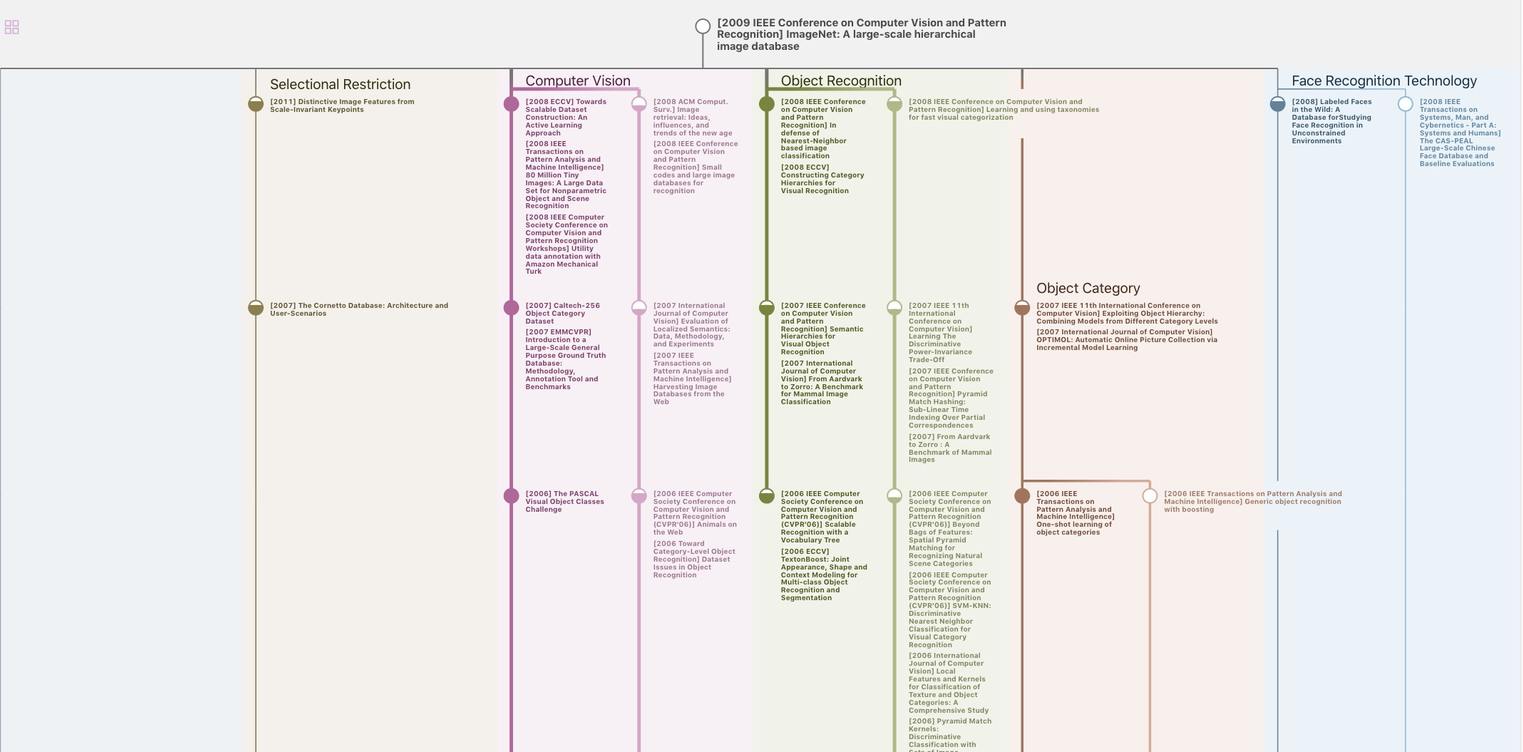
生成溯源树,研究论文发展脉络
Chat Paper
正在生成论文摘要