ChartReader: A Unified Framework for Chart Derendering and Comprehension without Heuristic Rules
2023 IEEE/CVF International Conference on Computer Vision (ICCV)(2023)
摘要
Charts are a powerful tool for visually conveying complex data, but their comprehension poses a challenge due to the diverse chart types and intricate components. Existing chart comprehension methods suffer from either heuristic rules or an over-reliance on OCR systems, resulting in suboptimal performance. To address these issues, we present ChartReader, a unified framework that seamlessly integrates chart derendering and comprehension tasks. Our approach includes a transformer-based chart component detection module and an extended pre-trained vision-language model for chart-to-X tasks. By learning the rules of charts automatically from annotated datasets, our approach eliminates the need for manual rule-making, reducing effort and enhancing accuracy.~We also introduce a data variable replacement technique and extend the input and position embeddings of the pre-trained model for cross-task training. We evaluate ChartReader on Chart-to-Table, ChartQA, and Chart-to-Text tasks, demonstrating its superiority over existing methods. Our proposed framework can significantly reduce the manual effort involved in chart analysis, providing a step towards a universal chart understanding model. Moreover, our approach offers opportunities for plug-and-play integration with mainstream LLMs such as T5 and TaPas, extending their capability to chart comprehension tasks. The code is available at https://github.com/zhiqic/ChartReader.
更多查看译文
关键词
chartreader derendering,comprehension
AI 理解论文
溯源树
样例
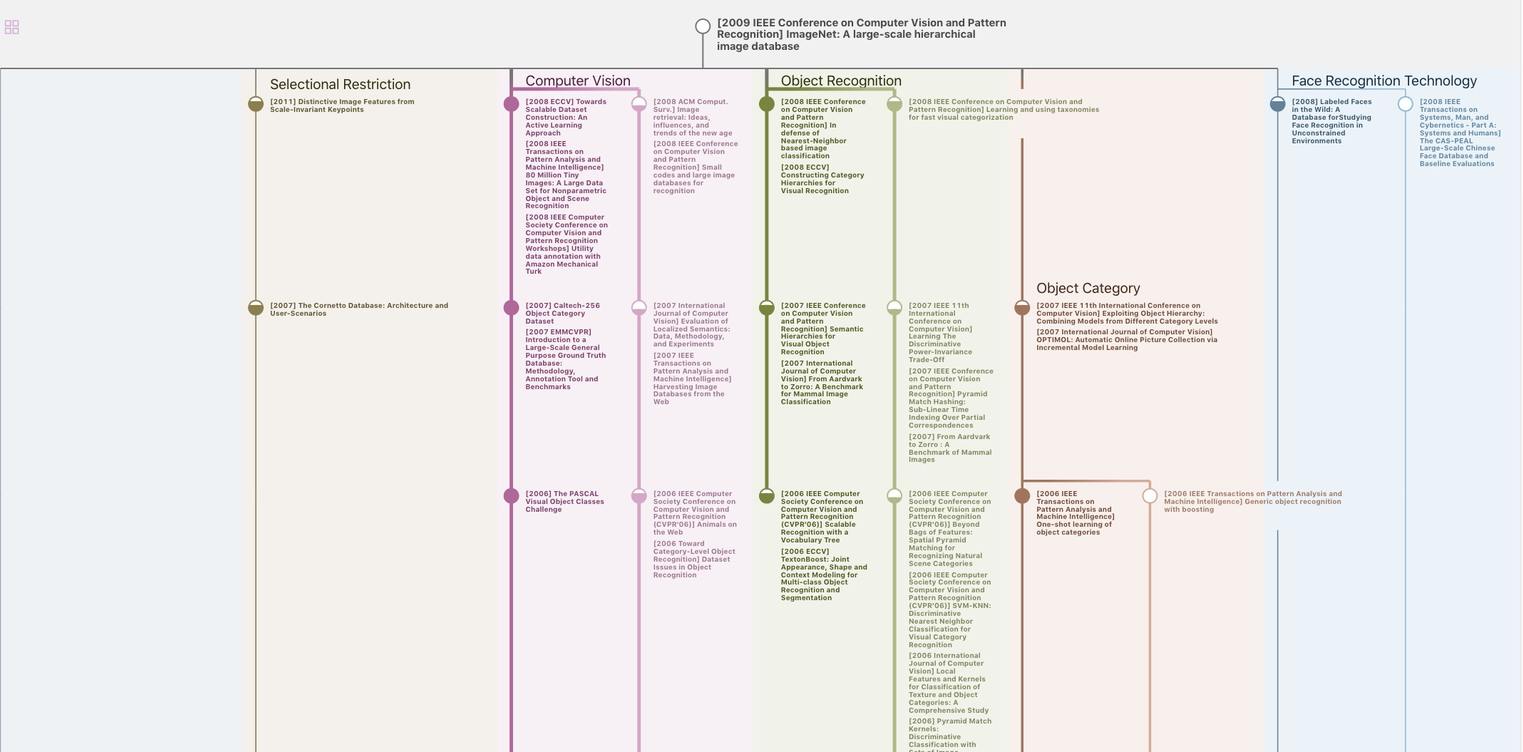
生成溯源树,研究论文发展脉络
Chat Paper
正在生成论文摘要