Segmentation and Analysis Emphasizing Neonatal MRI Brain Images Using Machine Learning Techniques
MATHEMATICS(2023)
摘要
MRI scanning has shown significant growth in the detection of brain tumors in the recent decade among various methods such as MRA, X-ray, CT, PET, SPECT, etc. Brain tumor identification requires high exactness because a minor error can be life-threatening. Brain tumor disclosure remains a challenging job in medical image processing. This paper targets to explicate a method that is more precise and accurate in brain tumor detection and focuses on tumors in neonatal brains. The infant brain varies from the adult brain in some aspects, and proper preprocessing technique proves to be fruitful to avoid miscues in results. This paper is divided into two parts: In the first half, preprocessing was accomplished using HE, CLAHE, and BPDFHE enhancement techniques. An analysis is the sequel to the above methods to check for the best method based on performance metrics, i.e., MSE, PSNR, RMSE, and AMBE. The second half deals with the segmentation process. We propose a novel ARKFCM to use for segmentation. Finally, the trends in the performance metrics (dice similarity and Jaccard similarity) as well as the segmentation results are discussed in comparison with the conventional FCM method.
更多查看译文
关键词
MRI segmentation,histogram equalization,CLAHE,BPDFHE,neonatal brain,ARKFCM,FCM
AI 理解论文
溯源树
样例
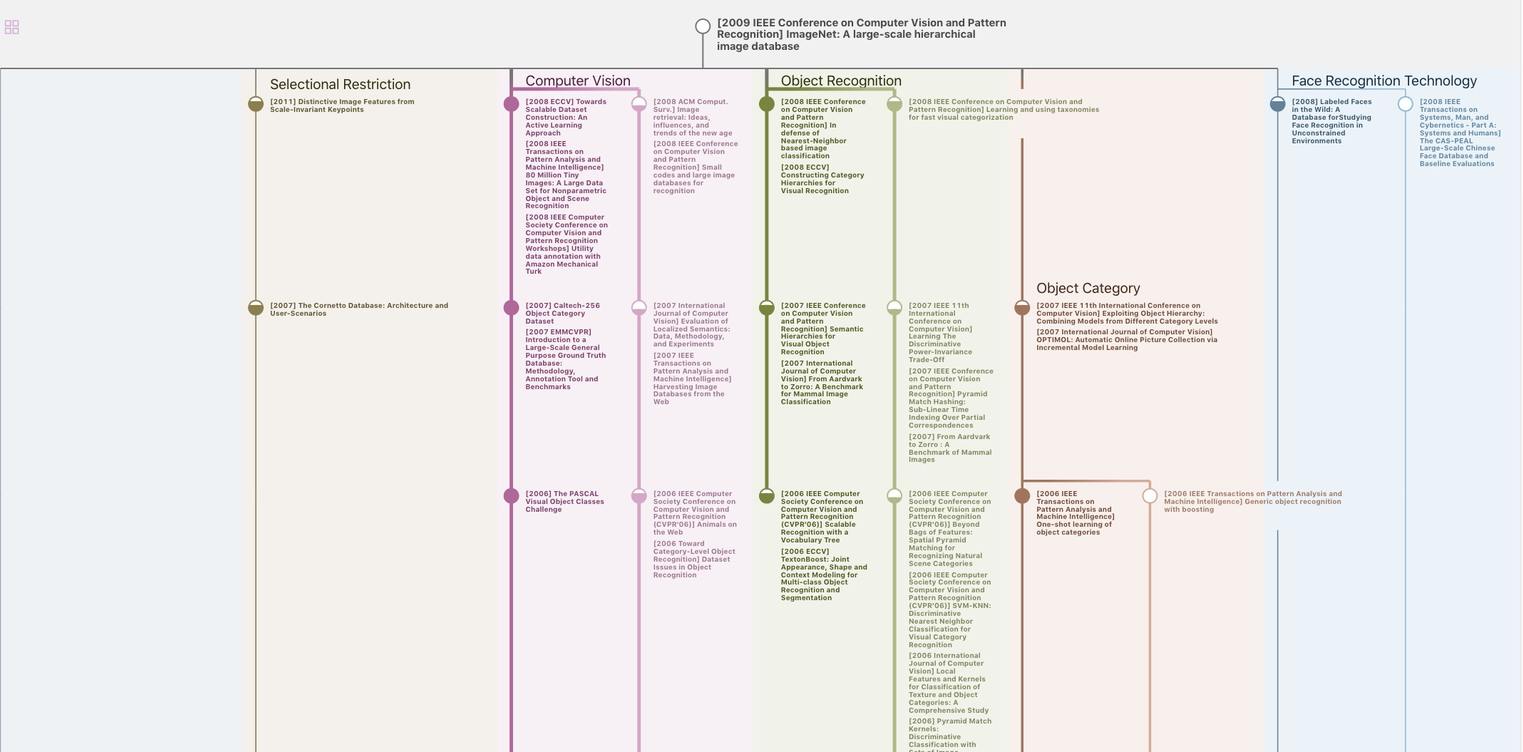
生成溯源树,研究论文发展脉络
Chat Paper
正在生成论文摘要