Designs to Improve Capability of Neural Networks to Make Structural Predictions
CHINESE JOURNAL OF POLYMER SCIENCE(2023)
摘要
A deep neural network model generally consists of different modules that play essential roles in performing a task. The optimal design of a module for use in modeling a physical problem is directly related to the success of the model. In this work, the effectiveness of a number of special modules, the self-attention mechanism for recognizing the importance of molecular sequence information in a polymer, as well as the big-stride representation and conditional random field for enhancing the network ability to produce desired local configurations, is numerically studied. Network models containing these modules are trained by using the well documented data of the native structures of the HP model and assessed according to their capability in making structural predictions of unseen data. The specific network design of self-attention mechanism adopted here is modified from a similar idea in natural language recognition. The big-stride representation module introduced in this work is shown to drastically improve network’s capability to model polymer segments of strong lattice position correlations.
更多查看译文
关键词
Deep neural network,Self-attention mechanism,Big-stride representation,Conditional random methods
AI 理解论文
溯源树
样例
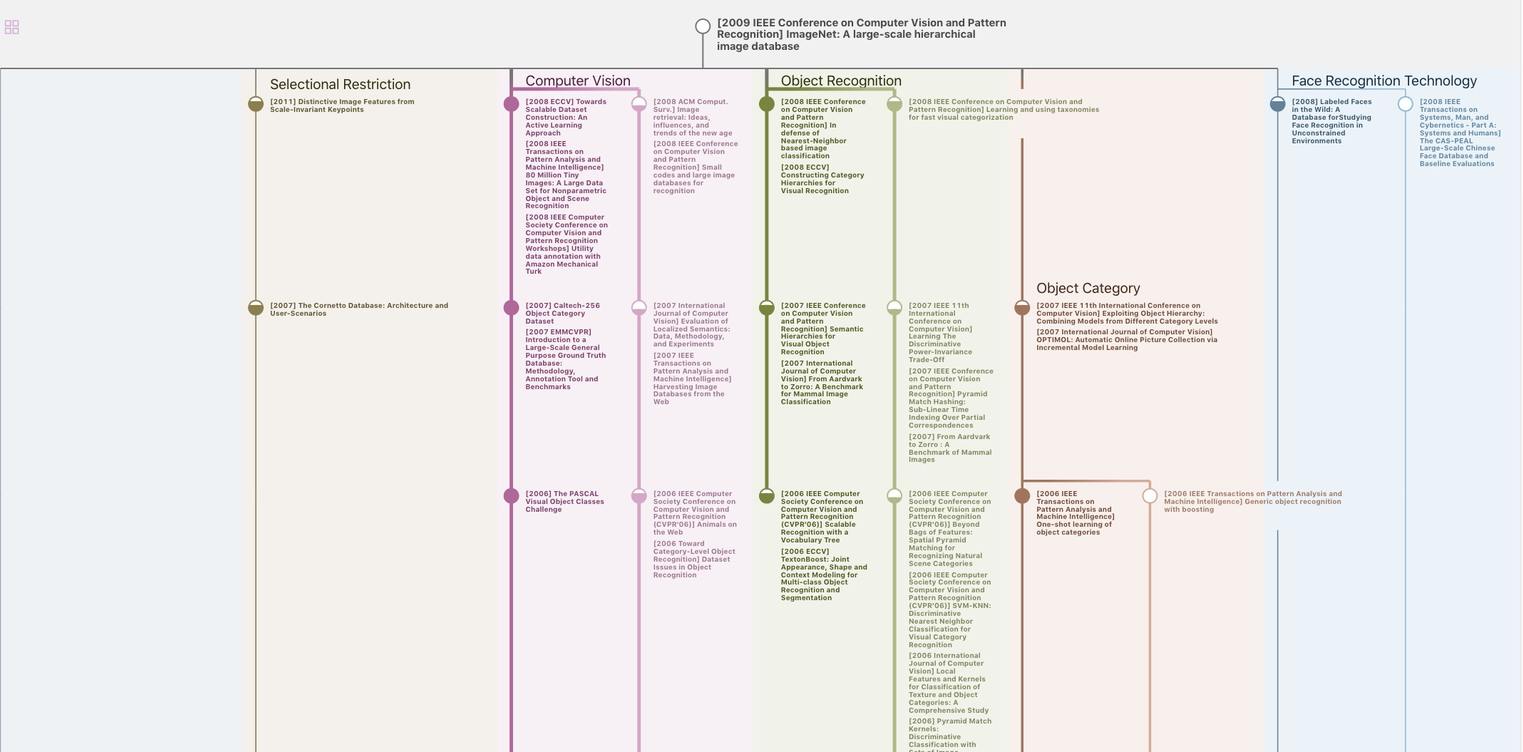
生成溯源树,研究论文发展脉络
Chat Paper
正在生成论文摘要