Efficient representations of binarized health deficit data: the frailty index and beyond
GEROSCIENCE(2023)
摘要
We investigated efficient representations of binarized health deficit data using the 2001–2002 National Health and Nutrition Examination Survey (NHANES). We compared the abilities of features to compress health deficit data and to predict adverse outcomes. We used principal component analysis (PCA) and several other dimensionality reduction techniques, together with several varieties of the frailty index (FI). We observed that the FI approximates the first — primary — component obtained by PCA and other compression techniques. Most adverse outcomes were well predicted using only the FI. While the FI is therefore a useful technique for compressing binary deficits into a single variable, additional dimensions were needed for high-fidelity compression of health deficit data. Moreover, some outcomes — including inflammation and metabolic dysfunction — showed high-dimensional behaviour. We generally found that clinical data were easier to compress than lab data. Our results help to explain the success of the FI as a simple dimensionality reduction technique for binary health data. We demonstrate how PCA extends the FI, providing additional health information, and allows us to explore system dimensionality and complexity. PCA is a promising tool for determining and exploring collective health features from collections of binarized biomarkers.
更多查看译文
关键词
Frailty index,Principal component analysis,Logistic principal component analysis,Dimensionality reduction,Biological age,Aging
AI 理解论文
溯源树
样例
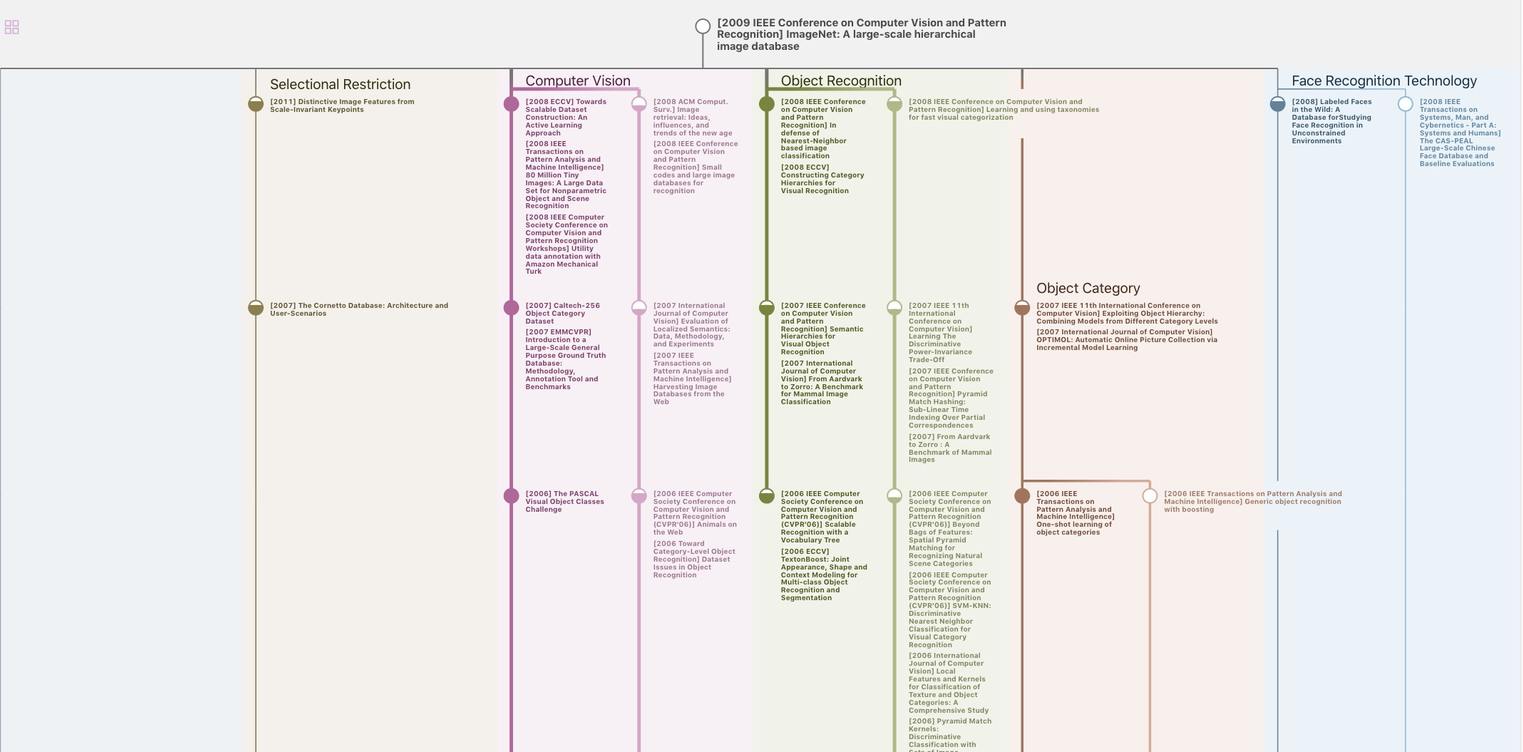
生成溯源树,研究论文发展脉络
Chat Paper
正在生成论文摘要