Physics-Informed Neural Networks for 1-D Steady-State Diffusion-Advection-Reaction Equations
NUCLEAR SCIENCE AND ENGINEERING(2023)
摘要
This work aims to solve six problems with four different physics-informed machine learning frameworks and compare the results in terms of accuracy and computational cost. First, we considered the diffusion-advection-reaction equations, which are second-order linear differential equations with two boundary conditions. The first algorithm is the classic Physics-Informed Neural Networks. The second one is Physics-Informed Extreme Learning Machine. The third framework is Deep Theory of Functional Connections, a multilayer neural network based on the solution approximation via a constrained expression that always analytically satisfies the boundary conditions. The last algorithm is the Extreme Theory of Functional Connections (X-TFC), which combines Theory of Functional Connections and shallow neural network with random features [e.g., Extreme Learning Machine (ELM)]. The results show that for these kinds of problems, ELM-based frameworks, especially X-TFC, overcome those using deep neural networks both in terms of accuracy and computational time.Keywords: Extreme Learning Machinefunctional interpolationPhysics-Informed Neural Networks Disclosure StatementNo potential conflict of interest was reported by the authors.
更多查看译文
关键词
Model Reduction,Data-Driven Modeling,Deep Learning,Nonlinear Systems
AI 理解论文
溯源树
样例
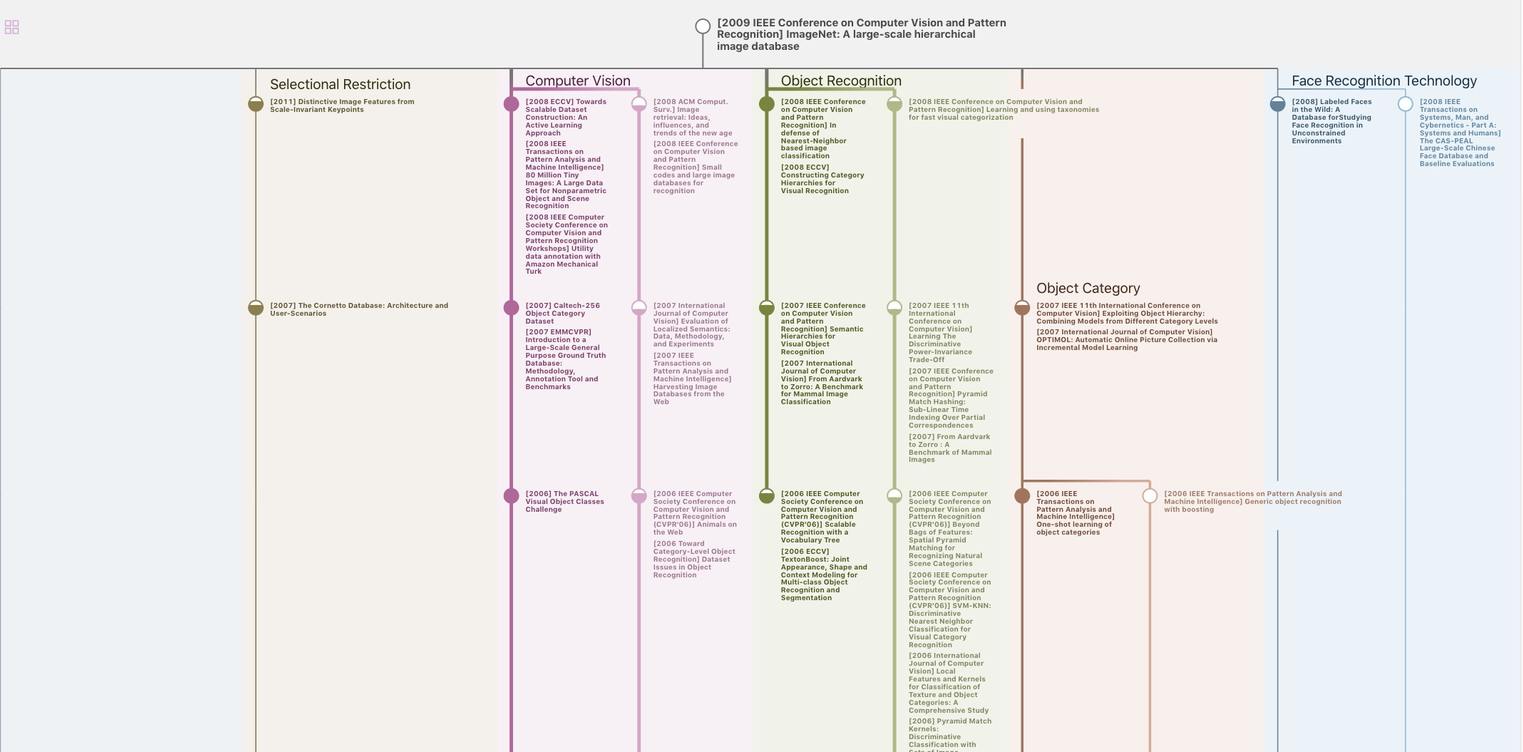
生成溯源树,研究论文发展脉络
Chat Paper
正在生成论文摘要