ECG signal generation based on conditional generative models
BIOMEDICAL SIGNAL PROCESSING AND CONTROL(2023)
摘要
Due to the high cost of labeling medical data such as electrocardiogram (ECG) signals, the performance of classifiers suffers significantly from the lack of annotated data. In recent years, generative models have achieved great success in image and natural language synthesis. However, for ECG synthesis, the method is still in its infancy. So far, researchers in this field mainly focus on Generative Adversarial Networks (GAN) or its variants. Besides, the model generally produces data separately for different classes. This strategy leads to a cumbersome generative process and bias to classes with few instances. We propose two unique ECG generators to address the above problems: Conditional Variational Auto-Encoder (CVAE) and Conditional Wasserstein Generative Adver-sarial Networks (CWGAN), which haven't been covered in previous works. We build simple networks for the encoder and decoder to demonstrate the strong potential of VAE for ECG synthesis. Additionally, we extend GAN-based ECG generators to a more practical version by conditioning the generative process. We use the MIT-BIH arrhythmia database for performance evaluation. The results show that the conditional generative framework can shorten the training time and simplify the generation process without significant performance loss for ECG generators. In particular, the VAE-based ECG generator shows similar potential to the GAN-based model.
更多查看译文
关键词
Conditional generative model,Variational Auto-Encoder,Generative adversarial networks,ECG synthesis
AI 理解论文
溯源树
样例
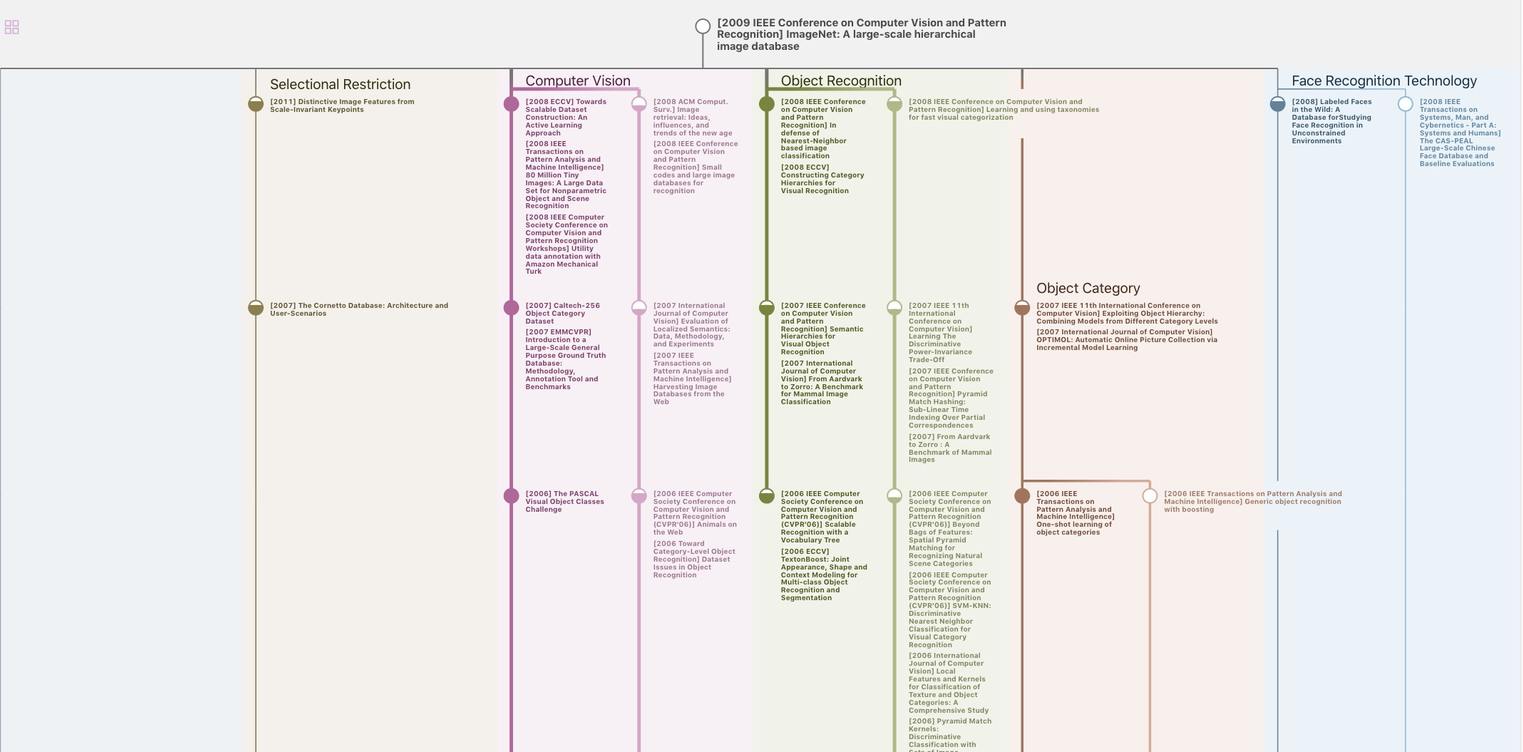
生成溯源树,研究论文发展脉络
Chat Paper
正在生成论文摘要