An adaptive image enhancement approach for safety monitoring robot under insufficient illumination condition
Comput. Ind.(2023)
摘要
The safety monitoring robots have been a potential solution to the task of patrol and inspection under uncontrolled complex environments such as tunnels. However, unclear semantic information and noises in low-light images will cause disturbance to the robot vision system. The deep learning-based enhancement is proven to advance the vision under poor illuminating conditions. However, the difficulties of excessive manual cost in collecting image pairs and image noise amplification are still challenging. In this work, we introduce a new adaptive image enhancement pipeline, which enables robots to self-adaptive to complex illumination conditions. With the pipeline, the IE-Module is proposed to learn image enhancement information from multi-scale feature blocks, and the SEnhance Module is used to eliminate the damage of denoising to normal pixels of the image. To make the algorithm better coupled with the safety monitoring robot, the algorithm is further enhanced with a filter threshold for adaptive to varied illumination conditions. The effectiveness of our method on both standard datasets and real-world scenarios was verified with experiments and real applications. The experiments on a low-light image benchmark as well as SLAM and 6D pose estimation experiments demonstrated that our method is superior to state-of-the-art methods qualitatively and quantitatively. Moreover, the proposed algorithm has been deployed in a real safety monitoring robot, which successfully demonstrates its capacity to perform patrol and inspection tasks in a low-light environment.
更多查看译文
关键词
Safety monitoring robot,Insufficient illumination condition,Image enhancement,Image denoise,Deep learning
AI 理解论文
溯源树
样例
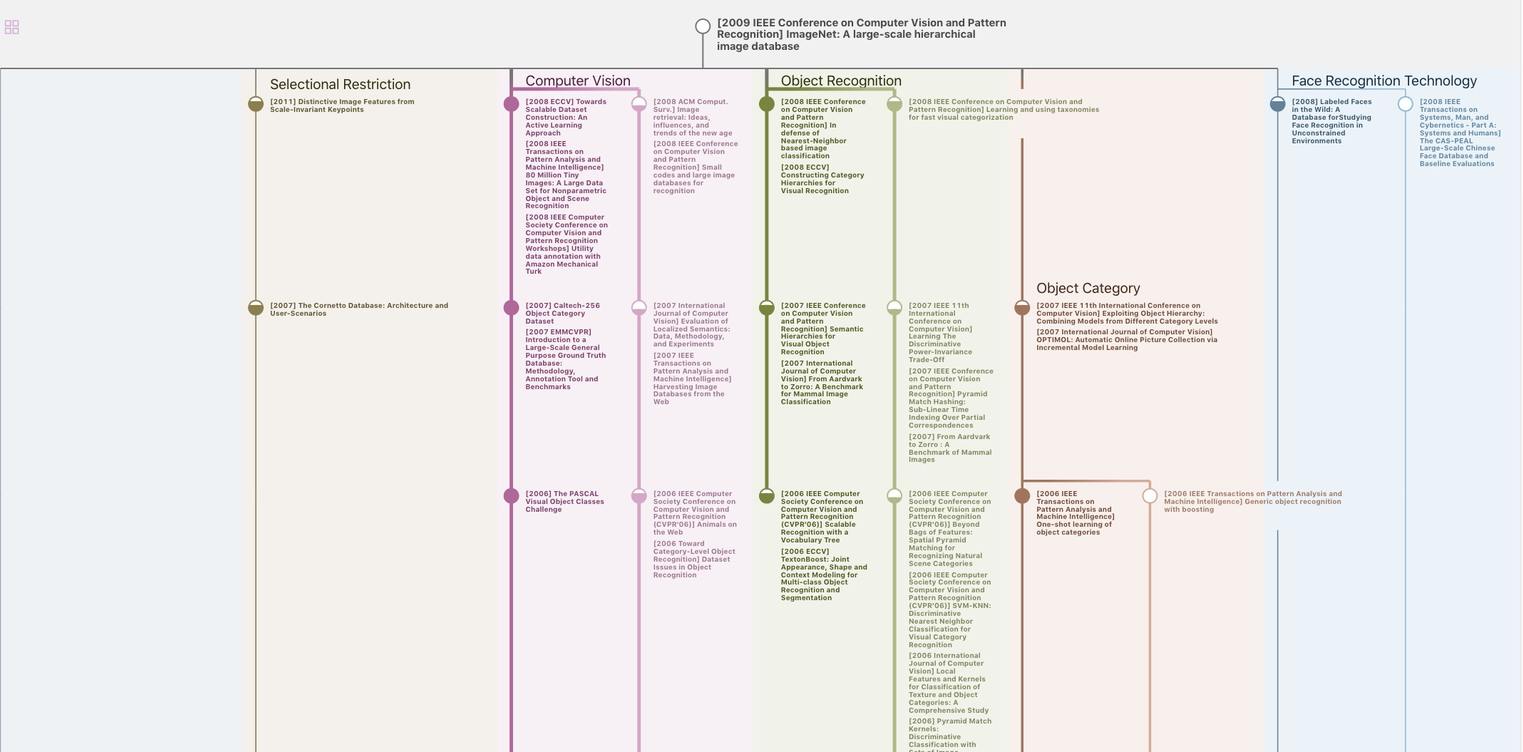
生成溯源树,研究论文发展脉络
Chat Paper
正在生成论文摘要