Automated diagnosis of flatfoot using cascaded convolutional neural network for angle measurements in weight-bearing lateral radiographs
European radiology(2023)
摘要
Objectives Diagnosis of flatfoot using a radiograph is subject to intra- and inter-observer variabilities. Here, we developed a cascade convolutional neural network (CNN)–based deep learning model (DLM) for an automated angle measurement for flatfoot diagnosis using landmark detection. Methods We used 1200 weight-bearing lateral foot radiographs from young adult Korean males for the model development. An experienced orthopedic surgeon identified 22 radiographic landmarks and measured three angles for flatfoot diagnosis that served as the ground truth (GT). Another orthopedic surgeon (OS) and a general physician (GP) independently identified the landmarks of the test dataset and measured the angles using the same method. External validation was performed using 100 and 17 radiographs acquired from a tertiary referral center and a public database, respectively. Results The DLM showed smaller absolute average errors from the GT for the three angle measurements for flatfoot diagnosis compared with both human observers. Under the guidance of the DLM, the average errors of observers OS and GP decreased from 2.35° ± 3.01° to 1.55° ± 2.09° and from 1.99° ± 2.76° to 1.56° ± 2.19°, respectively (both p < 0.001). The total measurement time decreased from 195 to 135 min in observer OS and from 205 to 155 min in observer GP. The absolute average errors of the DLM in the external validation sets were similar or superior to those of human observers in the original test dataset. Conclusions Our CNN model had significantly better accuracy and reliability than human observers in diagnosing flatfoot, and notably improved the accuracy and reliability of human observers. Key Points • Development of deep learning model (DLM) that allows automated angle measurements for landmark detection based on 1200 weight-bearing lateral radiographs for diagnosing flatfoot. • Our DLM showed smaller absolute average errors for flatfoot diagnosis compared with two human observers. • Under the guidance of the model, the average errors of two human observers decreased and total measurement time also decreased from 195 to 135 min and from 205 to 155 min.
更多查看译文
关键词
Computer-assisted diagnosis,Deep Learning,Flatfoot,Observer variation,X-rays
AI 理解论文
溯源树
样例
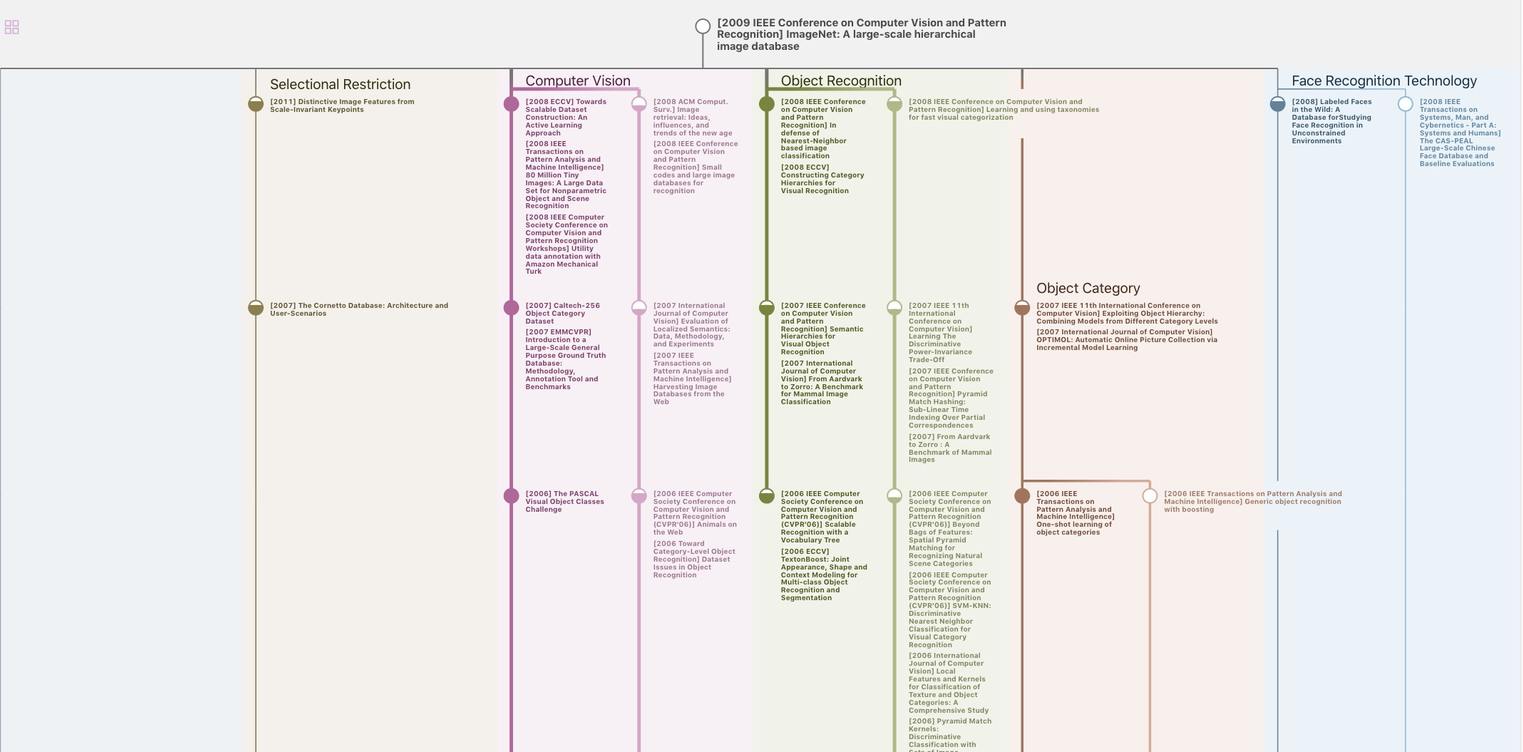
生成溯源树,研究论文发展脉络
Chat Paper
正在生成论文摘要