Toward DMC Accuracy Across Chemical Space with Scalable ?-QML
Journal of chemical theory and computation(2023)
摘要
In the past decade, quantum diffusion Monte Carlo (DMC) has been demonstrated to successfully predict the energetics and properties of a wide range of molecules and solids by numerically solving the electronic many-body Schro''dinger equation. With O(N3) scaling with the number of electrons N, DMC has the potential to be a reference method for larger systems that are not accessible to more traditional methods such as CCSD(T). Assessing the accuracy of DMC for smaller molecules becomes the stepping stone in making the method a reference for larger systems. We show that when coupled with quantum machine learning (QML)-based surrogate methods, the computational burden can be alleviated such that quantum Monte Carlo (QMC) shows clear potential to undergird the formation of high-quality descriptions across chemical space. We discuss three crucial approximations necessary to accomplish this: the fixed-node approximation, universal and accurate references for chemical bond dissociation energies, and scalable minimal amons-set-based QML (AQML) models. Numerical evidence presented includes converged DMC results for over 1000 small organic molecules with up to five heavy atoms used as amons and 50 medium-sized organic molecules with nine heavy atoms to validate the AQML predictions. Numerical evidence collected for Delta-AQML models suggests that already modestly sized QMC training data sets of amons suffice to predict total energies with near chemical accuracy throughout chemical space.
更多查看译文
AI 理解论文
溯源树
样例
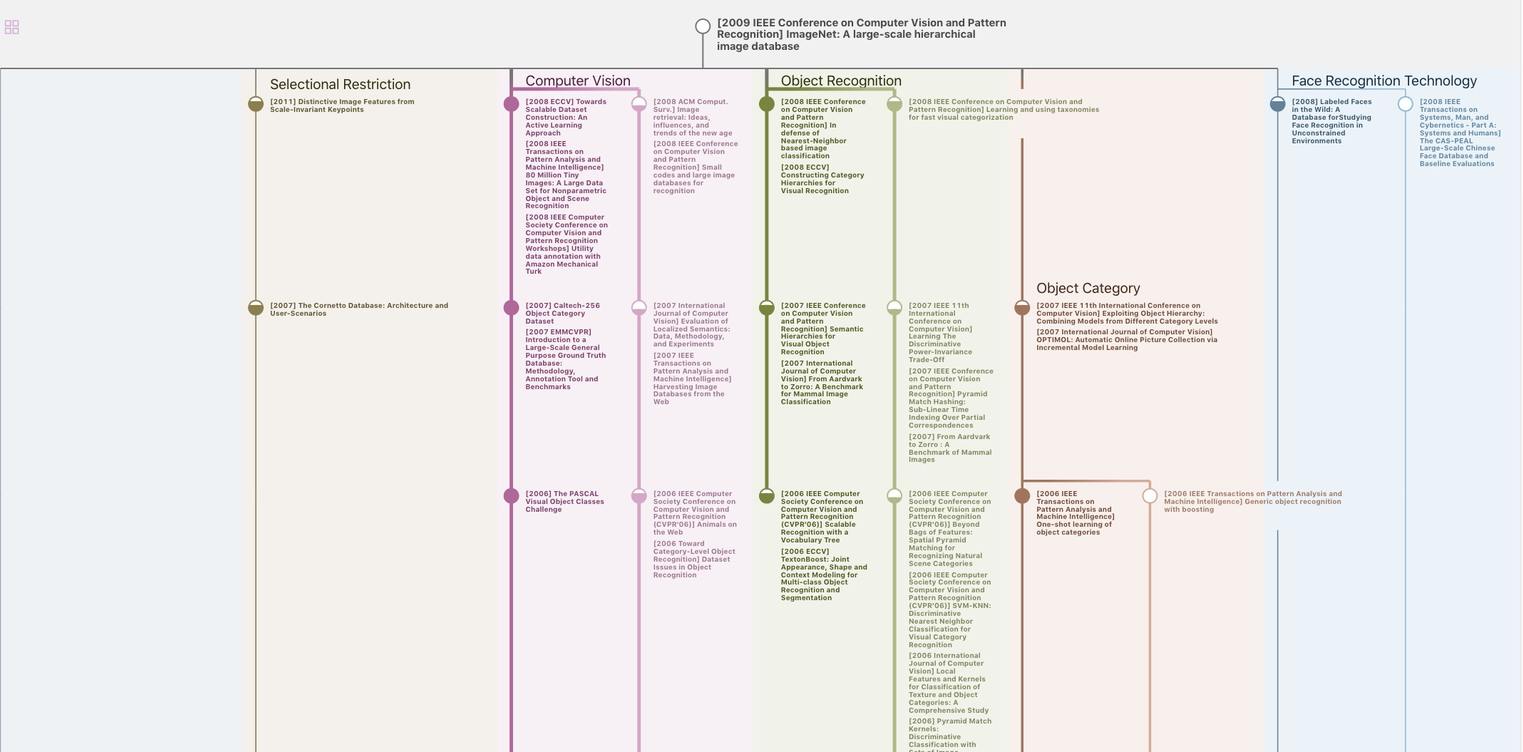
生成溯源树,研究论文发展脉络
Chat Paper
正在生成论文摘要