Convex Bounds on the Softmax Function with Applications to Robustness Verification
arxiv(2023)
摘要
The softmax function is a ubiquitous component at the output of neural networks and increasingly in intermediate layers as well. This paper provides convex lower bounds and concave upper bounds on the softmax function, which are compatible with convex optimization formulations for characterizing neural networks and other ML models. We derive bounds using both a natural exponential-reciprocal decomposition of the softmax as well as an alternative decomposition in terms of the log-sum-exp function. The new bounds are provably and/or numerically tighter than linear bounds obtained in previous work on robustness verification of transformers. As illustrations of the utility of the bounds, we apply them to verification of transformers as well as of the robustness of predictive uncertainty estimates of deep ensembles.
更多查看译文
关键词
softmax function,robustness
AI 理解论文
溯源树
样例
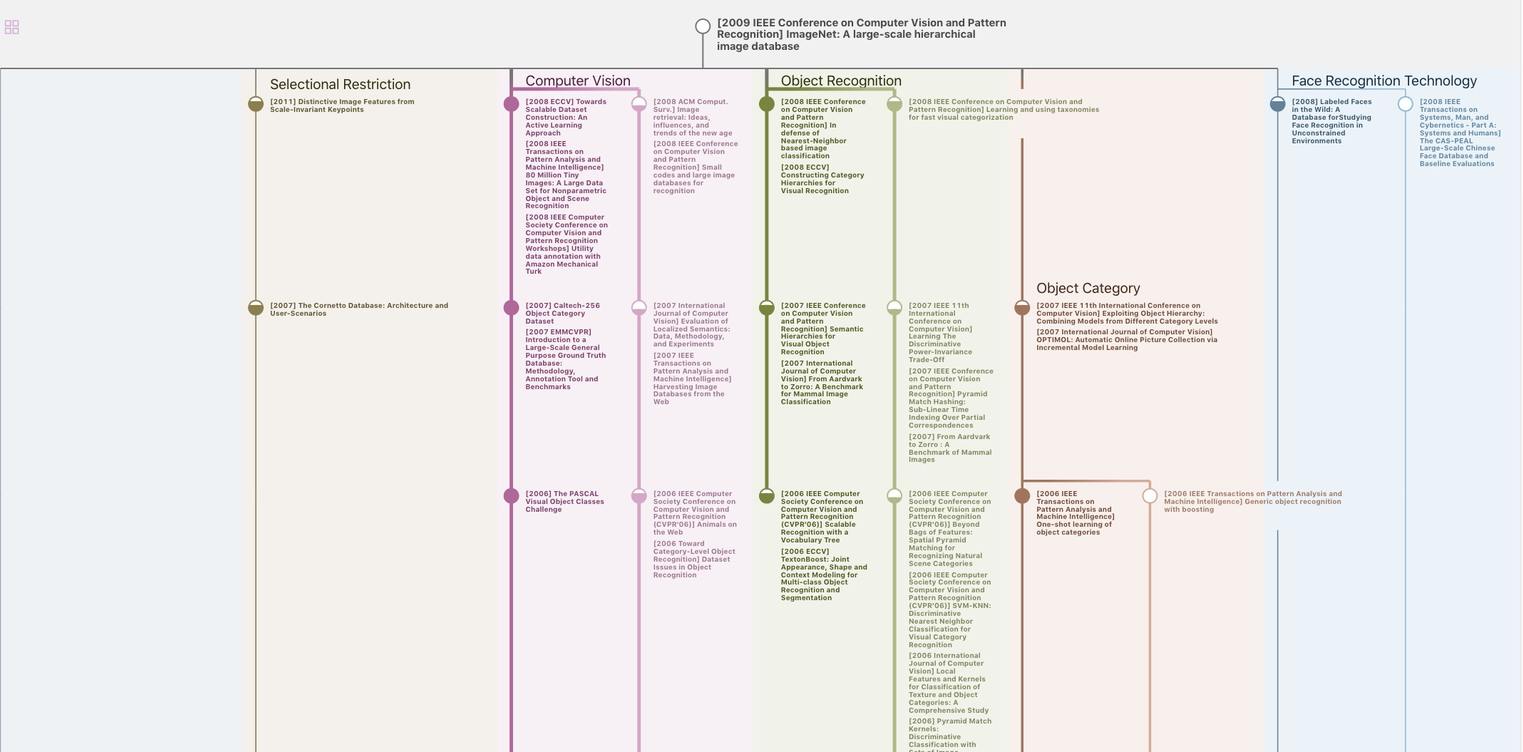
生成溯源树,研究论文发展脉络
Chat Paper
正在生成论文摘要