Solving Low-Rank Semidefinite Programs via Manifold Optimization
arxiv(2023)
摘要
We propose a manifold optimization approach to solve linear semidefinite programs (SDP) with low-rank solutions. This approach incorporates the augmented Lagrangian method and the Burer-Monteiro factorization, and features the adaptive strategies for updating the factorization size and the penalty parameter. We prove that the present algorithm can solve SDPs to global optimality, despite of the non-convexity brought by the Burer-Monteiro factorization. Along with the algorithm, we release an open-source SDP solver ManiSDP. Comprehensive numerical experiments demonstrate that ManiSDP achieves state-of-the-art in terms of efficiency, accuracy and scalability, and is faster than several advanced SDP solvers (MOSEK, SDPLR, SDPNAL+, STRIDE) by up to orders of magnitudes on a variety of linear SDPs. The largest SDP solved by ManiSDP (in about 8.5 hours with maximal KKT residue 3.5e-13) is the second-order moment relaxation of a binary quadratic program with 120 variables, which has matrix dimension 7261 and contains 17,869,161 affine constraints.
更多查看译文
关键词
manifold optimization,low-rank
AI 理解论文
溯源树
样例
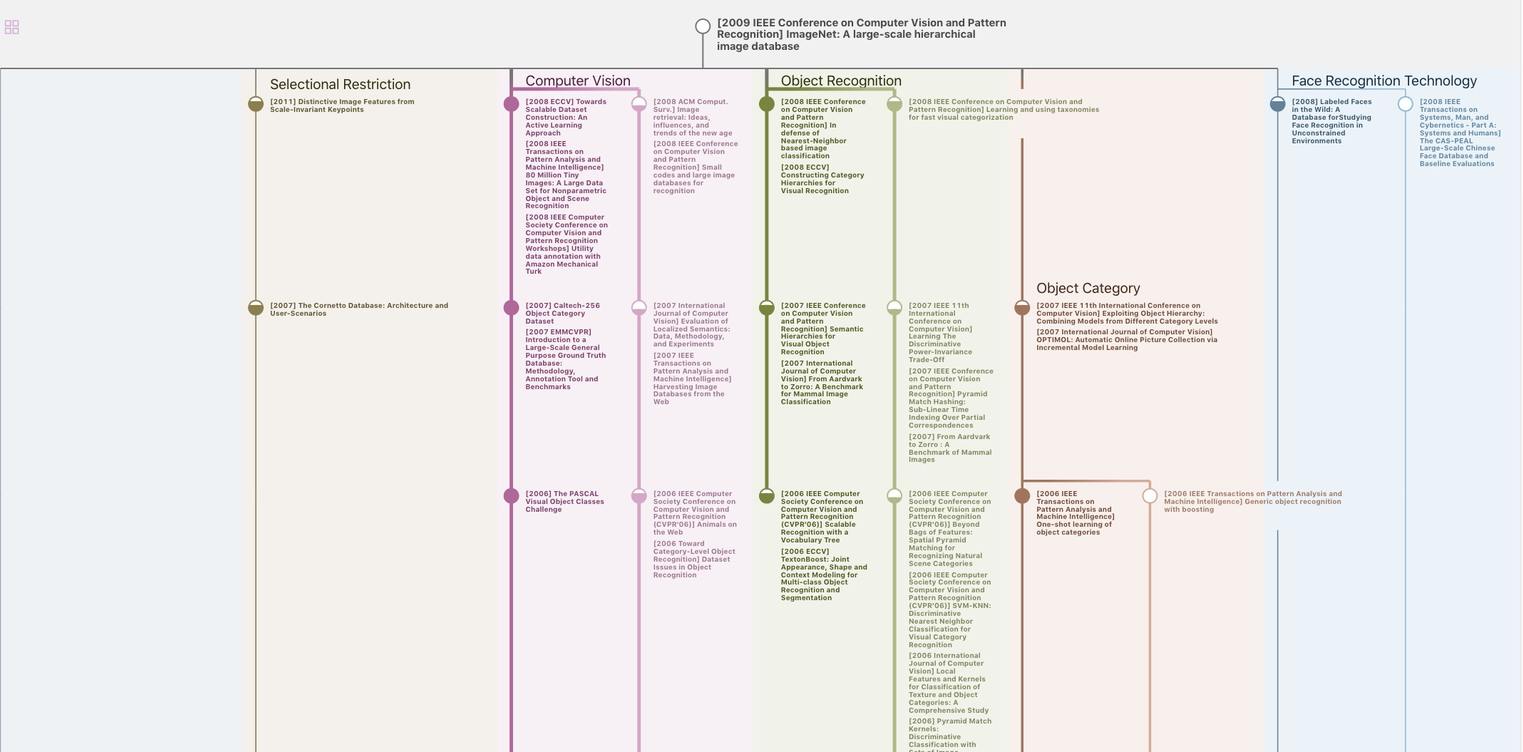
生成溯源树,研究论文发展脉络
Chat Paper
正在生成论文摘要