Machine learning using magnetic stochastic synapses
Neuromorphic Computing and Engineering(2023)
Abstract
The impressive performance of artificial neural networks has come at the cost of high energy usage and CO2 emissions. Unconventional computing architectures, with magnetic systems as a candidate, have potential as alternative energy-efficient hardware, but, still face challenges, such as stochastic behaviour, in implementation. Here, we present a methodology for exploiting the traditionally detrimental stochastic effects in magnetic domain-wall motion in nanowires. We demonstrate functional binary stochastic synapses alongside a gradient learning rule that allows their training with applicability to a range of stochastic systems. The rule, utilising the mean and variance of the neuronal output distribution, finds a trade-off between synaptic stochasticity and energy efficiency depending on the number of measurements of each synapse. For single measurements, the rule results in binary synapses with minimal stochasticity, sacrificing potential performance for robustness. For multiple measurements, synaptic distributions are broad, approximating better-performing continuous synapses. This observation allows us to choose design principles depending on the desired performance and the device's operational speed and energy cost. We verify performance on physical hardware, showing it is comparable to a standard neural network.
MoreTranslated text
Key words
neuromorphic,magnetic nanowire,binary stochastic synapses,gradient rule,spintronics
AI Read Science
Must-Reading Tree
Example
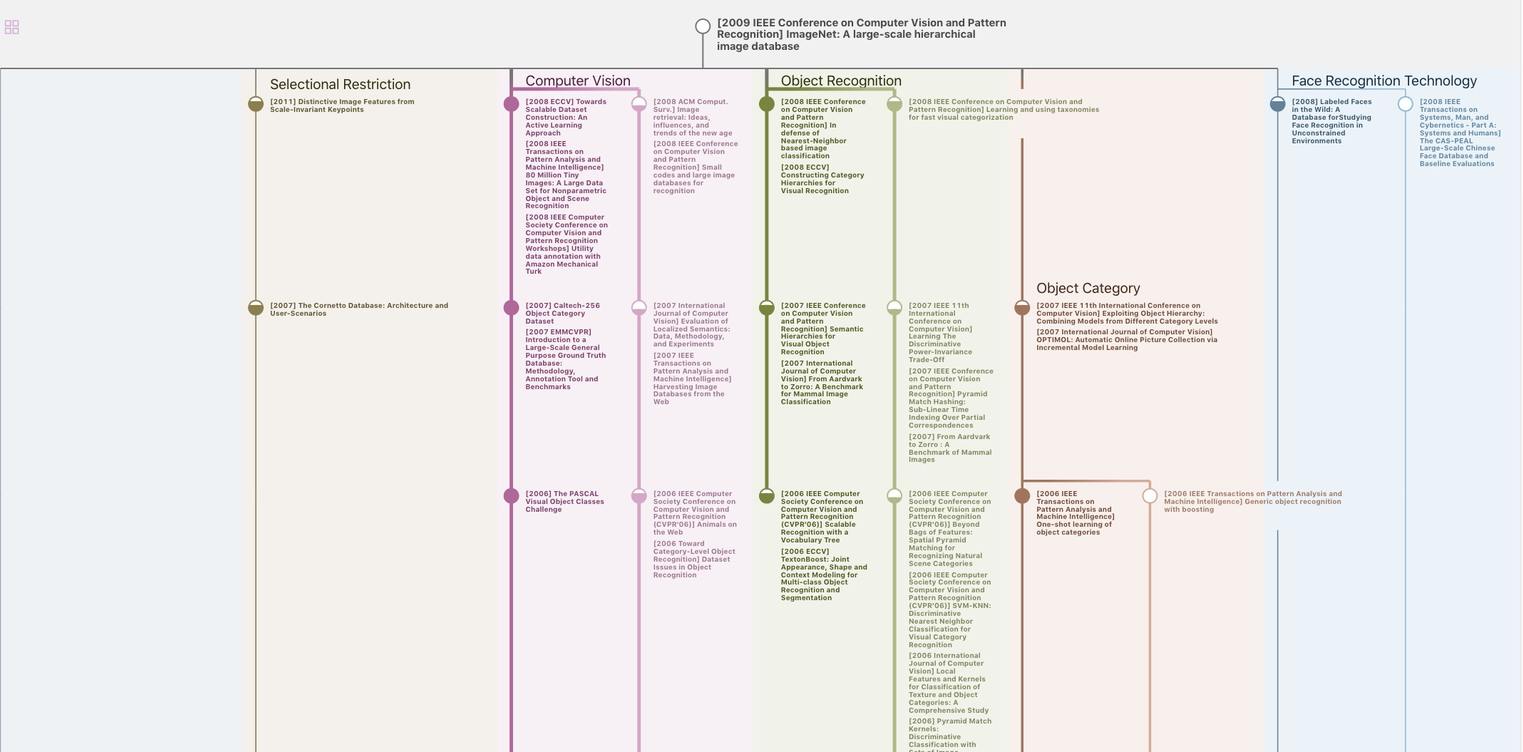
Generate MRT to find the research sequence of this paper
Chat Paper
Summary is being generated by the instructions you defined