Model-Agnostic Meta-Learning for Multilingual Hate Speech Detection
IEEE TRANSACTIONS ON COMPUTATIONAL SOCIAL SYSTEMS(2024)
摘要
Hate speech in social media is a growing phenomenon, and detecting such toxic content has recently gained significant traction in the research community. Existing studies have explored fine-tuning language models (LMs) to perform hate speech detection, and these solutions have yielded significant performance. However, most of these studies are limited to detecting hate speech only in English, neglecting the bulk of hateful content that is generated in other languages, particularly in low-resource languages. Developing a classifier that captures hate speech and nuances in a low-resource language with limited data is extremely challenging. To fill the research gap, we propose HateMAML, a model-agnostic meta-learning-based framework that effectively performs hate speech detection in low-resource languages. HateMAML utilizes a self-supervision strategy to overcome the limitation of data scarcity and produces better LM initialization for fast adaptation to an unseen target language (i.e., cross-lingual transfer) or other hate speech datasets (i.e., domain generalization). Extensive experiments are conducted on five datasets across eight different low-resource languages. The results show that HateMAML outperforms the state-of-the-art baselines by more than 3% in the cross-domain multilingual transfer setting. We also conduct ablation studies to analyze the characteristics of HateMAML.
更多查看译文
关键词
Cross-lingual transfer,hate speech detection,meta-learning
AI 理解论文
溯源树
样例
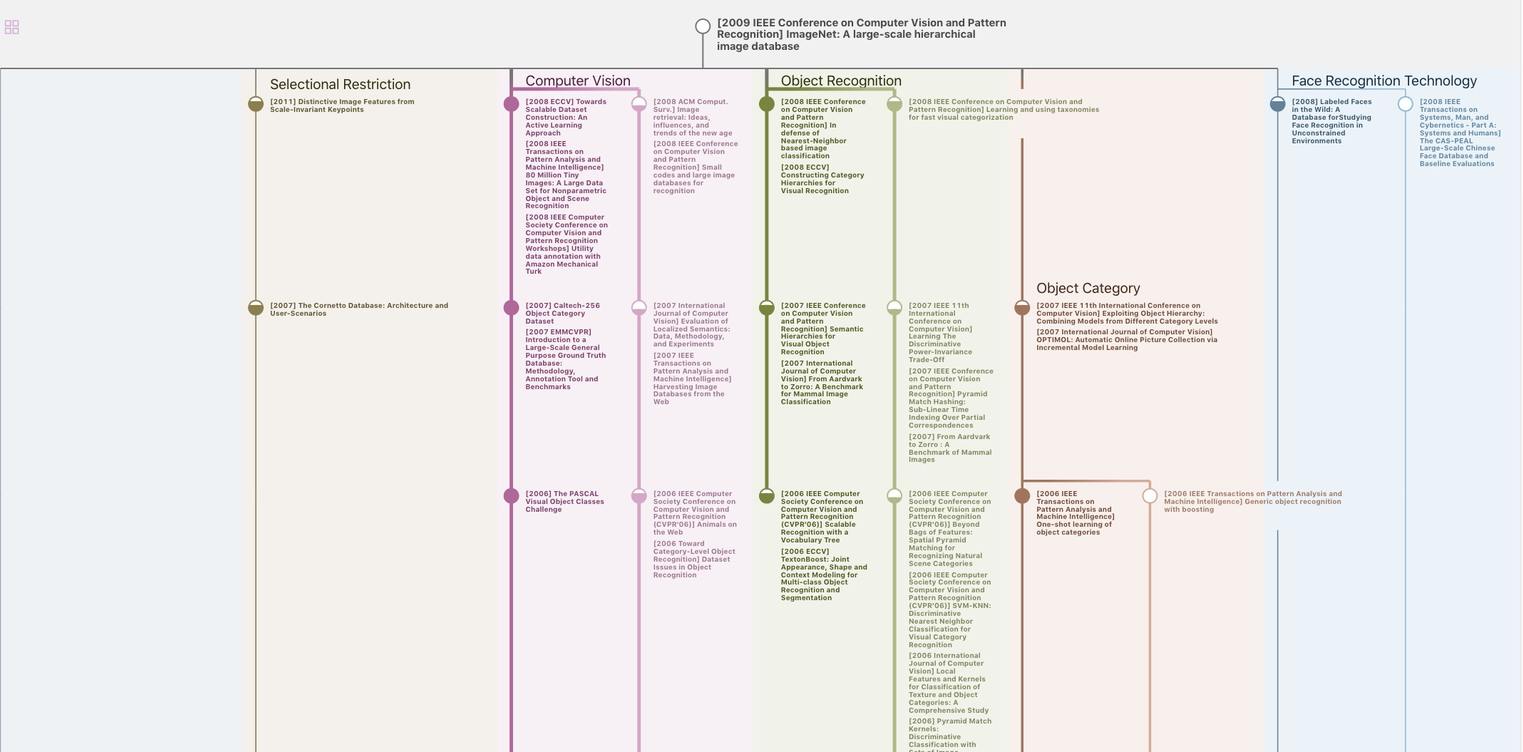
生成溯源树,研究论文发展脉络
Chat Paper
正在生成论文摘要