Semi-parametric inference based on adaptively collected data
arxiv(2023)
摘要
Many standard estimators, when applied to adaptively collected data, fail to be asymptotically normal, thereby complicating the construction of confidence intervals. We address this challenge in a semi-parametric context: estimating the parameter vector of a generalized linear regression model contaminated by a non-parametric nuisance component. We construct suitably weighted estimating equations that account for adaptivity in data collection, and provide conditions under which the associated estimates are asymptotically normal. Our results characterize the degree of "explorability" required for asymptotic normality to hold. For the simpler problem of estimating a linear functional, we provide similar guarantees under much weaker assumptions. We illustrate our general theory with concrete consequences for various problems, including standard linear bandits and sparse generalized bandits, and compare with other methods via simulation studies.
更多查看译文
关键词
data,semi-parametric
AI 理解论文
溯源树
样例
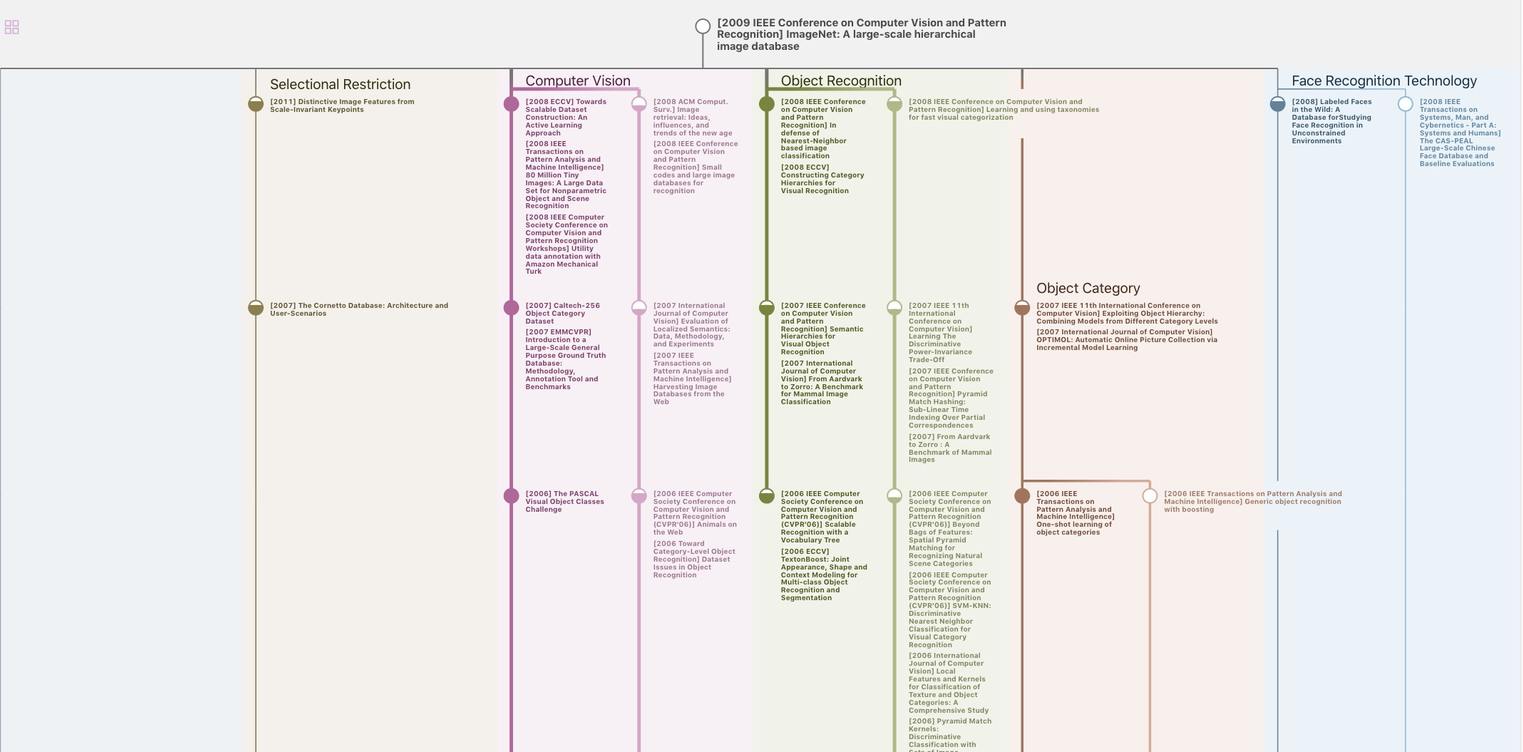
生成溯源树,研究论文发展脉络
Chat Paper
正在生成论文摘要