Estimating Racial Disparities when Race is Not Observed
Social Science Research Network(2024)
摘要
The estimation of racial disparities in various fields is often hampered bythe lack of individual-level racial information. In many cases, the lawprohibits the collection of such information to prevent direct racialdiscrimination. As a result, analysts have frequently adopted Bayesian ImprovedSurname Geocoding (BISG) and its variants, which combine individual names andaddresses with Census data to predict race. Unfortunately, the residuals ofBISG are often correlated with the outcomes of interest, generally attenuatingestimates of racial disparities. To correct this bias, we propose analternative identification strategy under the assumption that surname isconditionally independent of the outcome given (unobserved) race, residencelocation, and other observed characteristics. We introduce a new class ofmodels, Bayesian Instrumental Regression for Disparity Estimation (BIRDiE),that take BISG probabilities as inputs and produce racial disparity estimatesby using surnames as an instrumental variable for race. Our estimation methodis scalable, making it possible to analyze large-scale administrative data. Wealso show how to address potential violations of the key identificationassumptions. A validation study based on the North Carolina voter file showsthat BISG+BIRDiE reduces error by up to 84in party registration. Finally, we apply the proposed methodology to estimateracial differences in who benefits from the home mortgage interest deductionusing individual-level tax data from the U.S. Internal Revenue Service.Open-source software is available which implements the proposed methodology.
更多查看译文
AI 理解论文
溯源树
样例
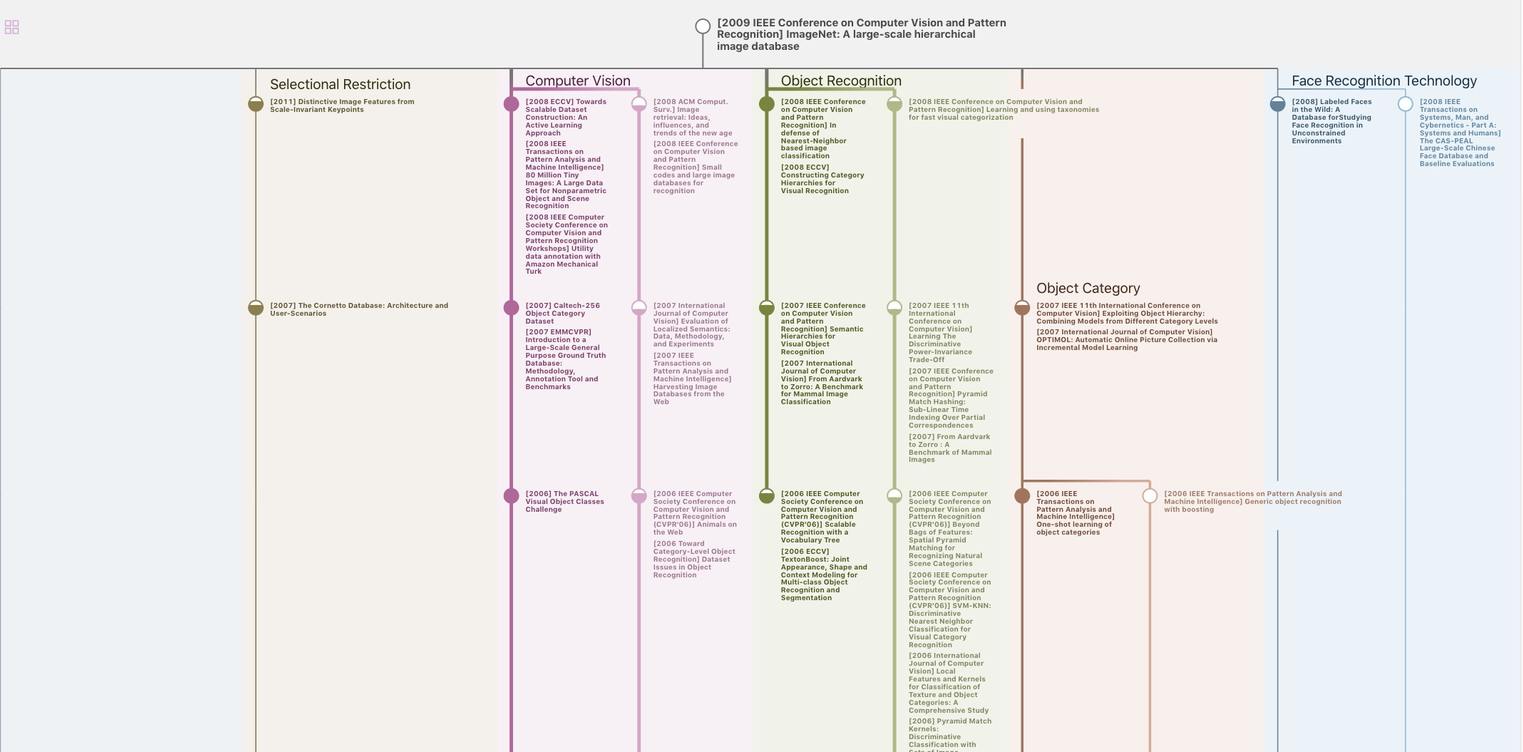
生成溯源树,研究论文发展脉络
Chat Paper
正在生成论文摘要