Expressiveness and Structure Preservation in Learning Port-Hamiltonian Systems
arxiv(2023)
摘要
A well-specified parametrization for single-input/single-output (SISO) linear port-Hamiltonian systems amenable to structure-preserving supervised learning is provided. The construction is based on controllable and observable normal form Hamiltonian representations for those systems, which reveal fundamental relationships between classical notions in control theory and crucial properties in the machine learning context, like structure-preservation and expressive power. The results in the paper suggest parametrizations of the estimation problem associated with these systems that amount, at least in the canonical case, to unique identification and prove that the parameter complexity necessary for the replication of the dynamics is only O(n) and not O(n^2), as suggested by the standard parametrization of these systems.
更多查看译文
关键词
structure preservation,learning,systems,port-hamiltonian
AI 理解论文
溯源树
样例
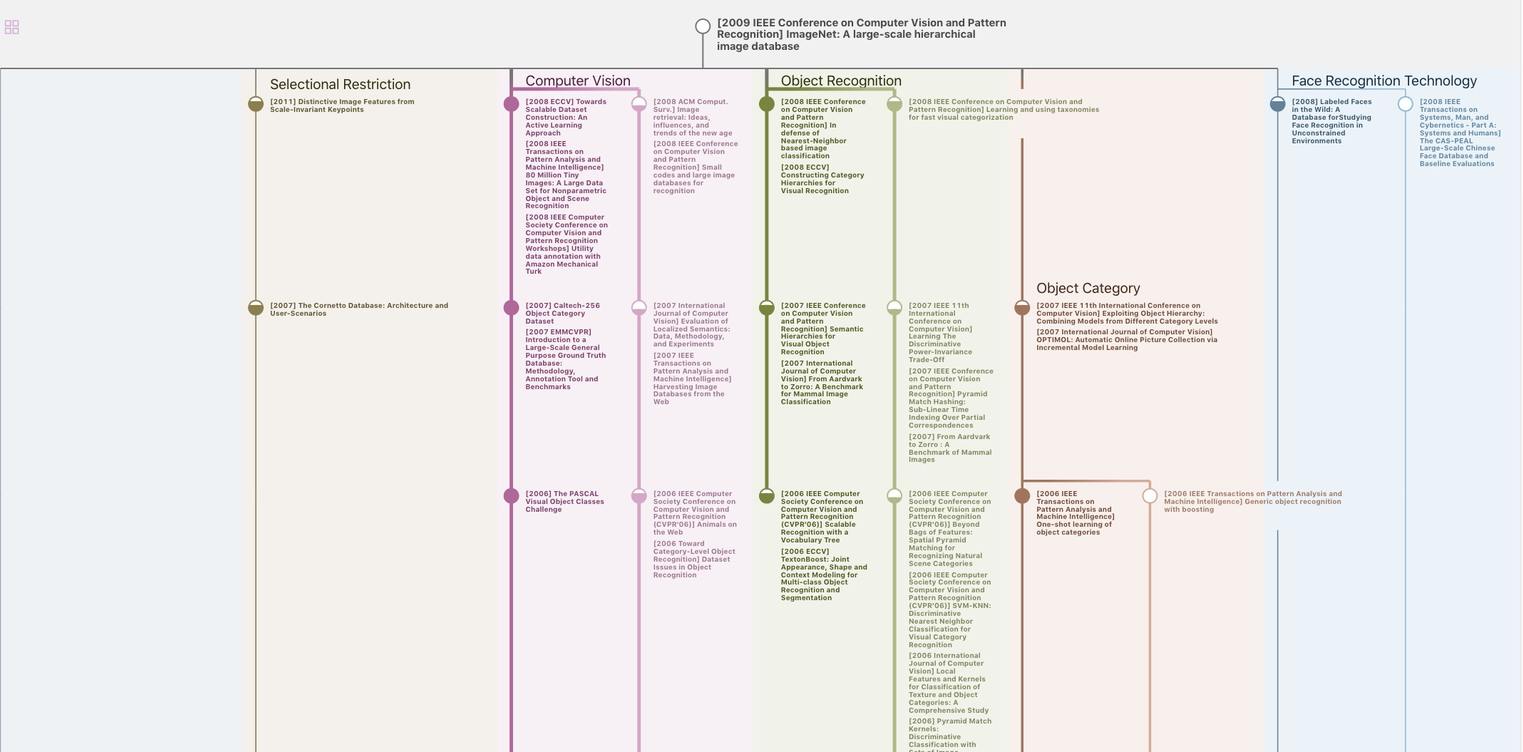
生成溯源树,研究论文发展脉络
Chat Paper
正在生成论文摘要