Variational Quantum Eigensolver for Classification in Credit Sales Risk
arxiv(2023)
摘要
In this work, a classification task is realized by the Variational Quantum Eigensolver (VQE) approach. A decision situation concerns issuing commodities in the process of credit sales -- at some point a client's history may be analyzed by debt department's employee to check if the goods issuing should not be stopped due to the risk of fraud (bivalent classification). As in the classical machine learning, the data should be normalized. We describe the two-step process of data normalization to write each observation as a quantum state. The VQE approach allows training the parameters of a quantum circuit (so-called ansatz) to output pattern-states for each class. Observations are categorized to classes with the use of the SWAP-test. The obtained solution is compact and requires only logarithmically increasing number of qubits (due to the exponential capacity of quantum registers) -- we also present alternative classical solutions which, in fact, are quite complex. All calculations, plots, and comparisons were implemented and conduced in Python language environment. Source codes of the simulations for individual examples of quantum classification can be found in the source code repository. The version of a simulator directly used in the article is in the Zenodo version of the source code.
更多查看译文
关键词
variational quantum eigensolver,classification
AI 理解论文
溯源树
样例
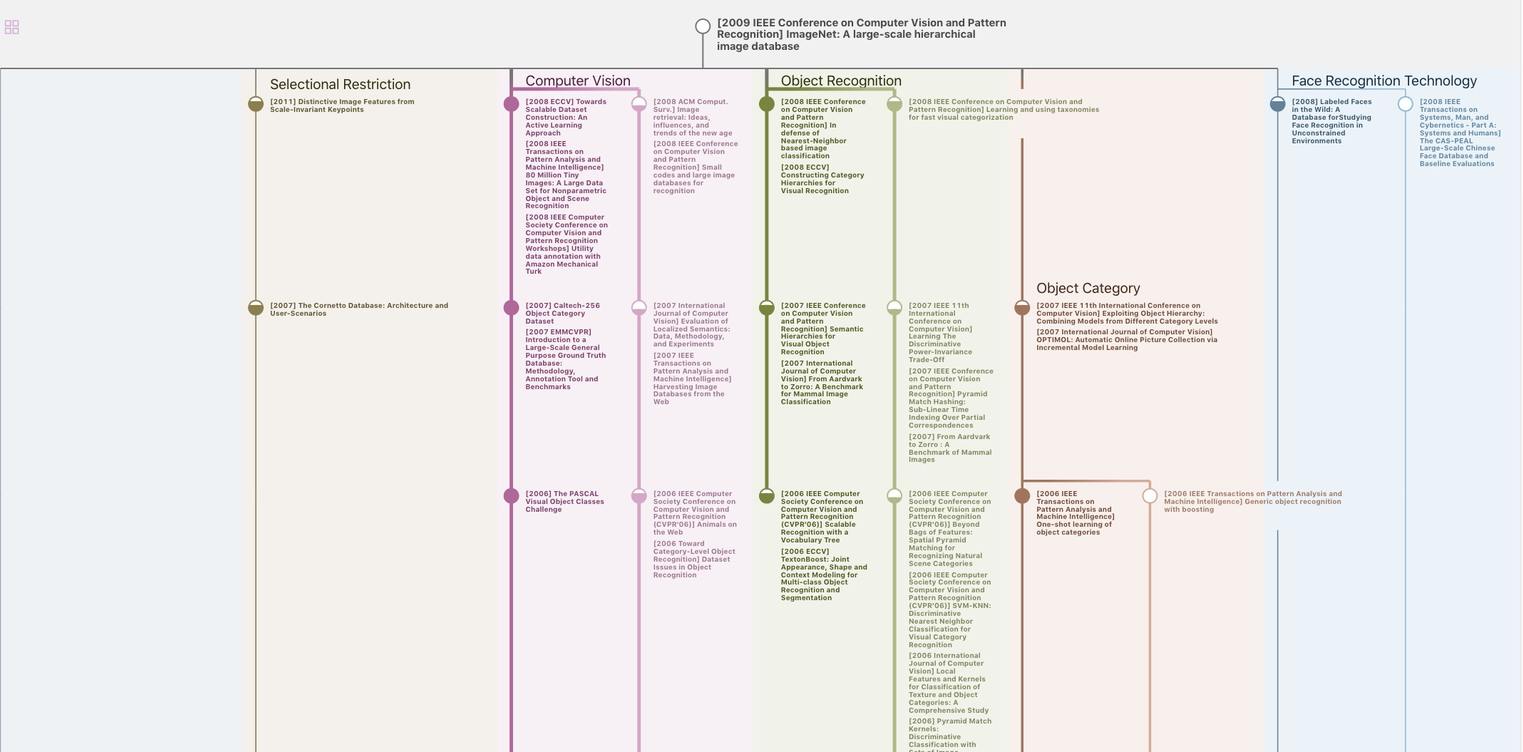
生成溯源树,研究论文发展脉络
Chat Paper
正在生成论文摘要