Graph Neural Network Autoencoders for Efficient Quantum Circuit Optimisation
arxiv(2023)
摘要
Reinforcement learning (RL) is a promising method for quantum circuit optimisation. However, the state space that has to be explored by an RL agent is extremely large when considering all the possibilities in which a quantum circuit can be transformed through local rewrite operations. This state space explosion slows down the learning of RL-based optimisation strategies. We present for the first time how to use graph neural network (GNN) autoencoders for the optimisation of quantum circuits. We construct directed acyclic graphs from the quantum circuits, encode the graphs and use the encodings to represent RL states. We illustrate our proof of concept implementation on Bernstein-Vazirani circuits and, from preliminary results, we conclude that our autoencoder approach: a) maintains the optimality of the original RL method; b) reduces by 20 \% the size of the table that encodes the learned optimisation strategy. Our method is the first realistic first step towards very large scale RL quantum circuit optimisation.
更多查看译文
关键词
efficient quantum circuit
AI 理解论文
溯源树
样例
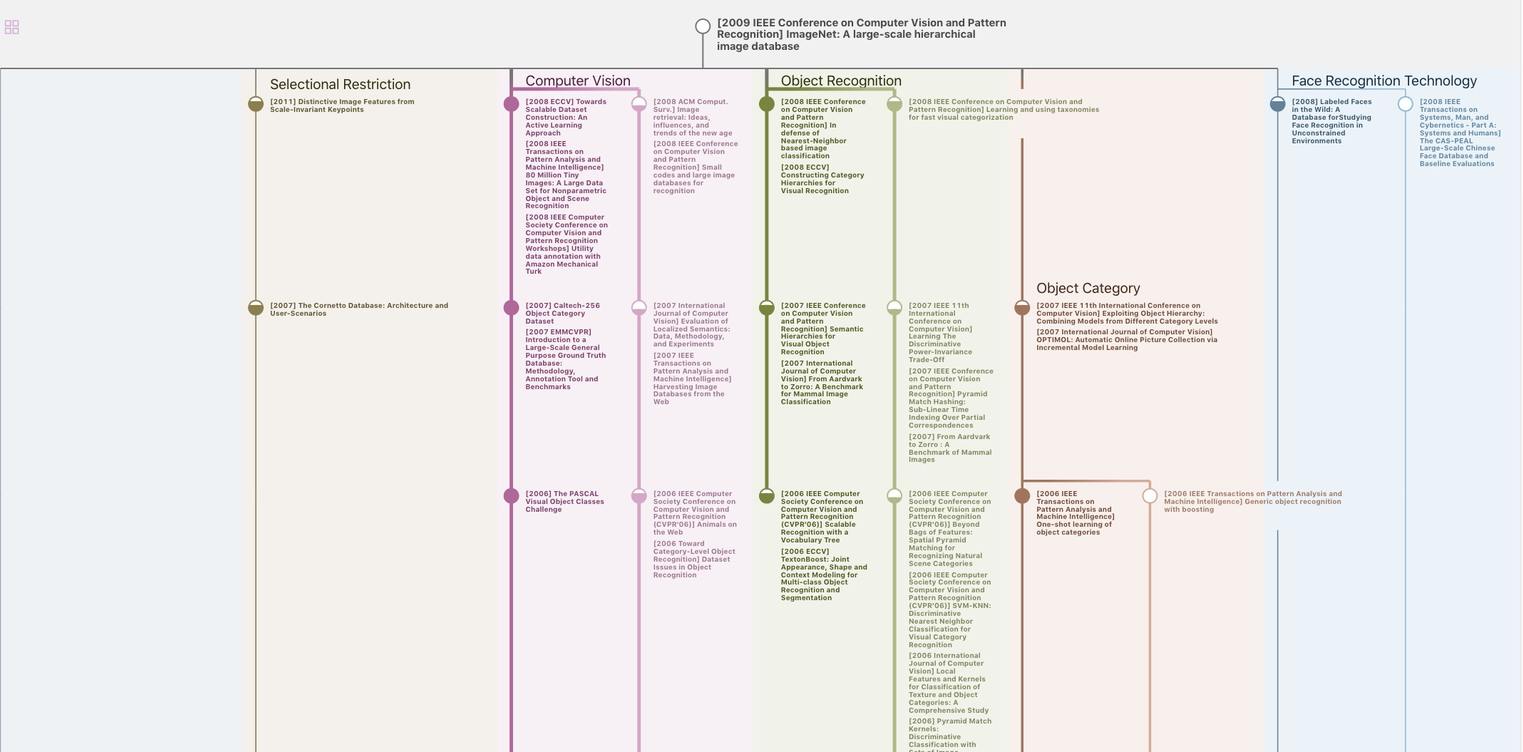
生成溯源树,研究论文发展脉络
Chat Paper
正在生成论文摘要