Can Membership Inferencing be Refuted?
arxiv(2023)
摘要
Membership inference (MI) attack is currently the most popular test for measuring privacy leakage in machine learning models. Given a machine learning model, a data point and some auxiliary information, the goal of an MI attack is to determine whether the data point was used to train the model. In this work, we study the reliability of membership inference attacks in practice. Specifically, we show that a model owner can plausibly refute the result of a membership inference test on a data point $x$ by constructing a proof of repudiation that proves that the model was trained without $x$. We design efficient algorithms to construct proofs of repudiation for all data points of the training dataset. Our empirical evaluation demonstrates the practical feasibility of our algorithm by constructing proofs of repudiation for popular machine learning models on MNIST and CIFAR-10. Consequently, our results call for a re-evaluation of the implications of membership inference attacks in practice.
更多查看译文
关键词
membership inferencing
AI 理解论文
溯源树
样例
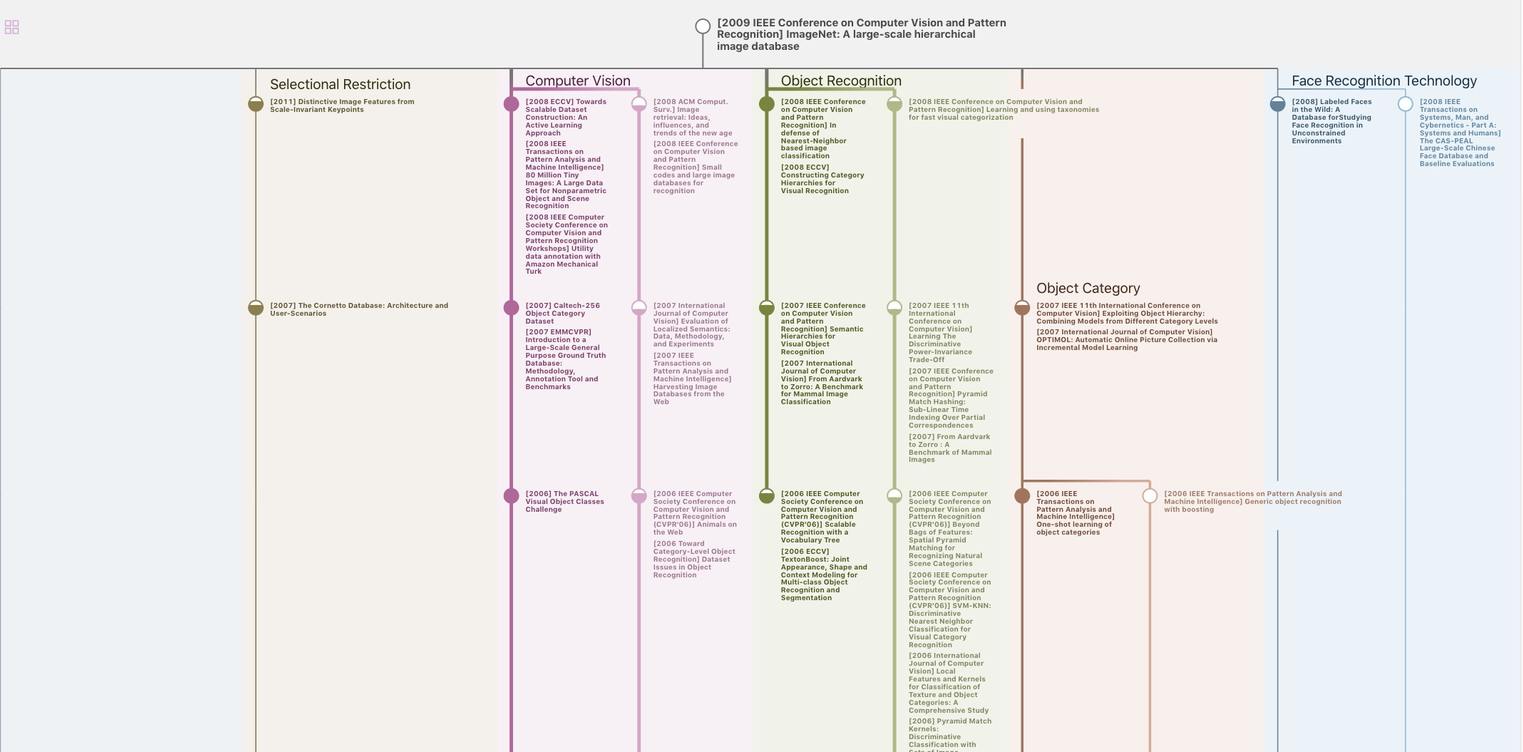
生成溯源树,研究论文发展脉络
Chat Paper
正在生成论文摘要