Deep Reinforcement Learning for Beam Angle Optimization of Intensity-Modulated Radiation Therapy
arxiv(2023)
摘要
Objective: Intensity-modulated radiation therapy (IMRT) beam angle optimization (BAO) is a challenging combinatorial optimization problem that is NP-hard. In this study, we aim to develop a personalized BAO algorithm for IMRT that improves the quality of the final treatment. Methods: To improve the quality of IMRT treatment planning, we propose a deep reinforcement learning (DRL)-based approach for IMRT BAO. We consider the task as a sequential decision-making problem and formulate it as a Markov Decision Process. To facilitate the training process, a 3D-Unet is designed to predict the dose distribution for the different number of beam angles, ranging from 1 to 9, to simulate the IMRT environment. By leveraging the simulation model, double deep-Q network (DDQN) and proximal policy optimization (PPO) are used to train agents to select the personalized beam angle sequentially within a few seconds. Results: The treatment plans with beam angles selected by DRL outperform those with clinically used evenly distributed beam angles. For DDQN, the overall average improvement of the CIs is 0.027, 0.032, and 0.03 for 5, 7, and 9 beam angles respectively. For PPO, the overall average improvement of CIs is 0.045, 0.051, and 0.025 for 5, 7, and 9 beam angles respectively. Conclusion: The proposed DRL-based beam angle selection strategy can generate personalized beam angles within a few seconds, and the resulting treatment plan is superior to that obtained using evenly distributed angles. Significance: A fast and automated personalized beam angle selection approach is been proposed for IMRT BAO.
更多查看译文
AI 理解论文
溯源树
样例
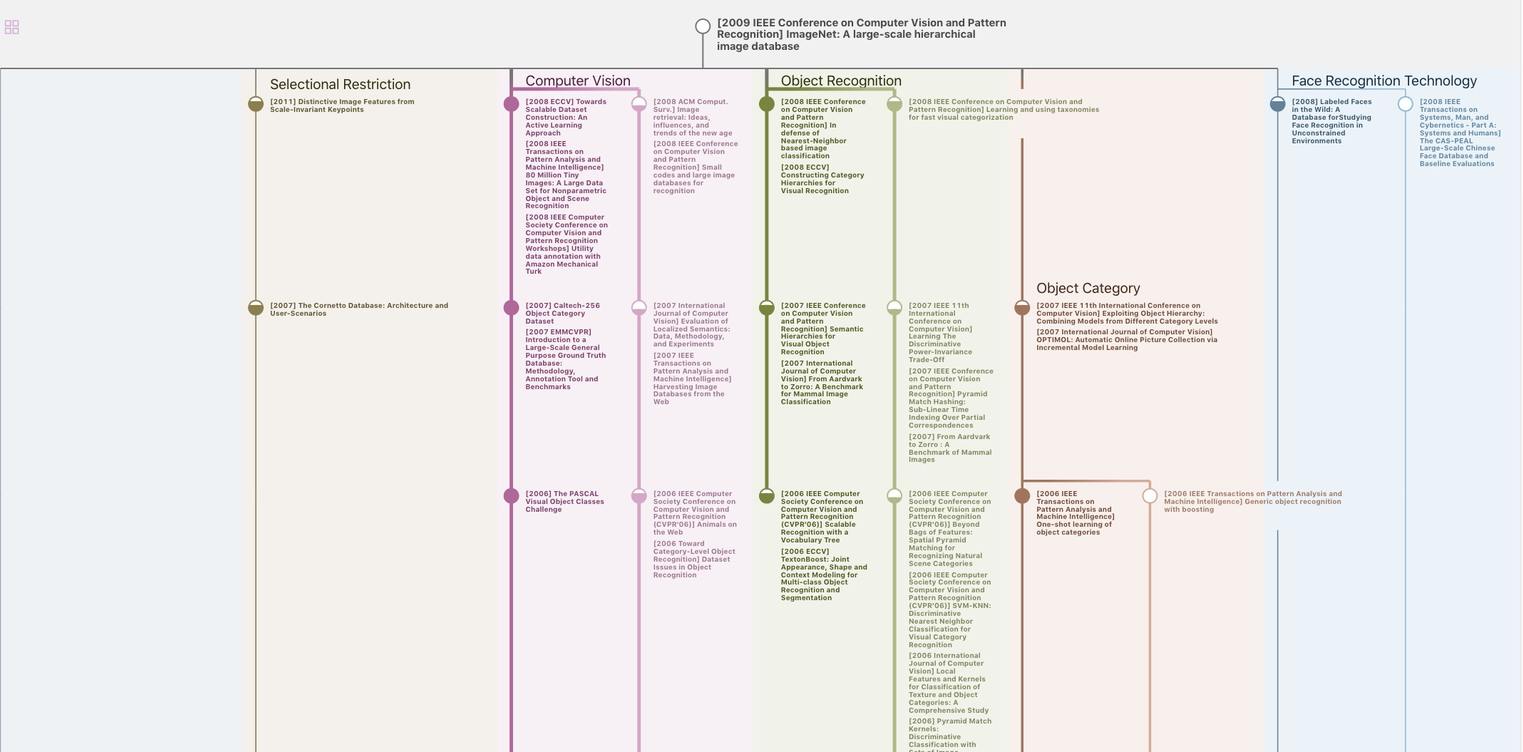
生成溯源树,研究论文发展脉络
Chat Paper
正在生成论文摘要