Performance Analysis of Various Cancers Using Genetic Data with Variance Threshold.
OCIT(2022)
摘要
Cancer is the leading risk factor for death. It is critical to develop effective methods for identifying cancer types and detecting them early. Because of the TCGA (The Cancer genome atlas), genomic data is now more widely available and used extensively. This study applies machine learning techniques to an RNA-Seq gene expression dataset to classify the many types and subtypes of cancer. These datasets typically have multiple dimensions and hundreds of columns with no labels. Because of this, creating high-performing production models requires a certain machine learning implementation. The development and application of machine learning models for the study of genomic datasets is continued in this research work. Machine learning methods are employed to examine and compare cancer categorization in our study. Decision Tree, Naive Bayes, Support Vector Machine (SVM), K-Nearest Neighbour (KNN) and Random Forest are the algorithms used. The dataset is high throughput sequencing RNA seq data which includes 22 types of cancer along with their subtypes as well as normal types of samples. To improve model accuracy, the data is preprocessed, oversampling technique SMOTE and feature selection approaches are applied. As a result, we have compared the comparative parameters like accuracy, area under the ROC Curve (AUC) score, precision, recall, F1 score and receiver operating characteristic curve (ROC) curve. The results show that SVM shows the best accuracy when feature selection and oversampling technique is applied.
更多查看译文
关键词
RNA Sequencing,The Cancer genome atlas(TCGA),Support Vector Machine,Receiver operating characteristic curve,K-Nearest Neighbor,Area under the ROC,Synthetic minority oversampling (SMOTE)
AI 理解论文
溯源树
样例
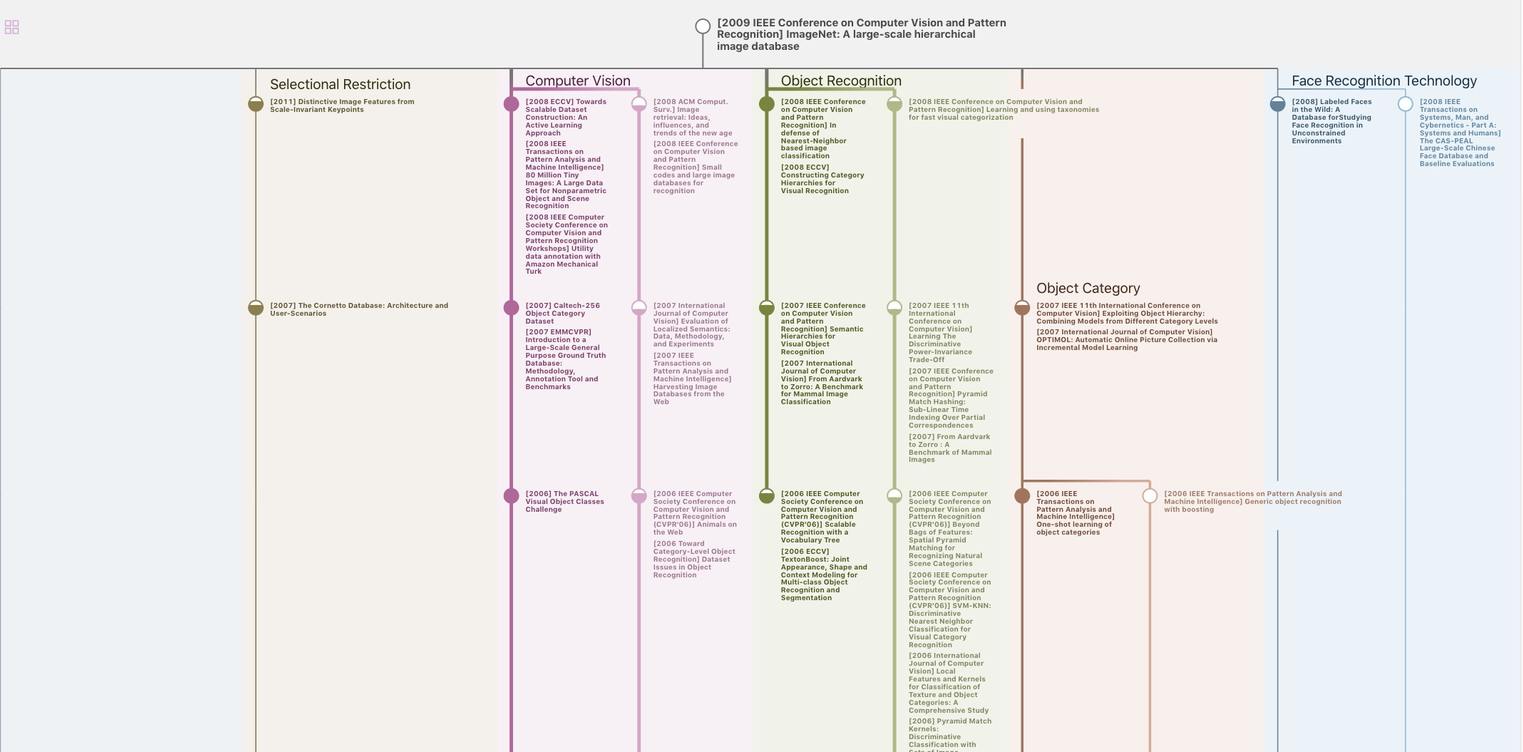
生成溯源树,研究论文发展脉络
Chat Paper
正在生成论文摘要