Classification of Patient Recovery From COVID-19 Symptoms Using Consumer Wearables and Machine Learning
IEEE Journal of Biomedical and Health Informatics(2023)
摘要
Current remote monitoring of COVID-19 patients relies on manual symptom reporting, which is highly dependent on patient compliance. In this research, we present a machine learning (ML)-based remote monitoring method to estimate patient recovery from COVID-19 symptoms using automatically collected wearable device data, instead of relying on manually collected symptom data. We deploy our remote monitoring system, namely eCOVID, in two COVID-19 telemedicine clinics. Our system utilizes a Garmin wearable and symptom tracker mobile app for data collection. The data consists of vitals, lifestyle, and symptom information which is fused into an online report for clinicians to review. Symptom data collected via our mobile app is used to label the recovery status of each patient daily. We propose a ML-based binary patient recovery classifier which uses wearable data to estimate whether a patient has recovered from COVID-19 symptoms. We evaluate our method using leave-one-subject-out (LOSO) cross-validation, and find that Random Forest (RF) is the top performing model. Our method achieves an F1-score of 0.88 when applying our RF-based model personalization technique using weighted bootstrap aggregation. Our results demonstrate that ML-assisted remote monitoring using automatically collected wearable data can supplement or be used in place of manual daily symptom tracking which relies on patient compliance.
更多查看译文
关键词
Machine learning,wearables,remote patient monitoring,COVID-19
AI 理解论文
溯源树
样例
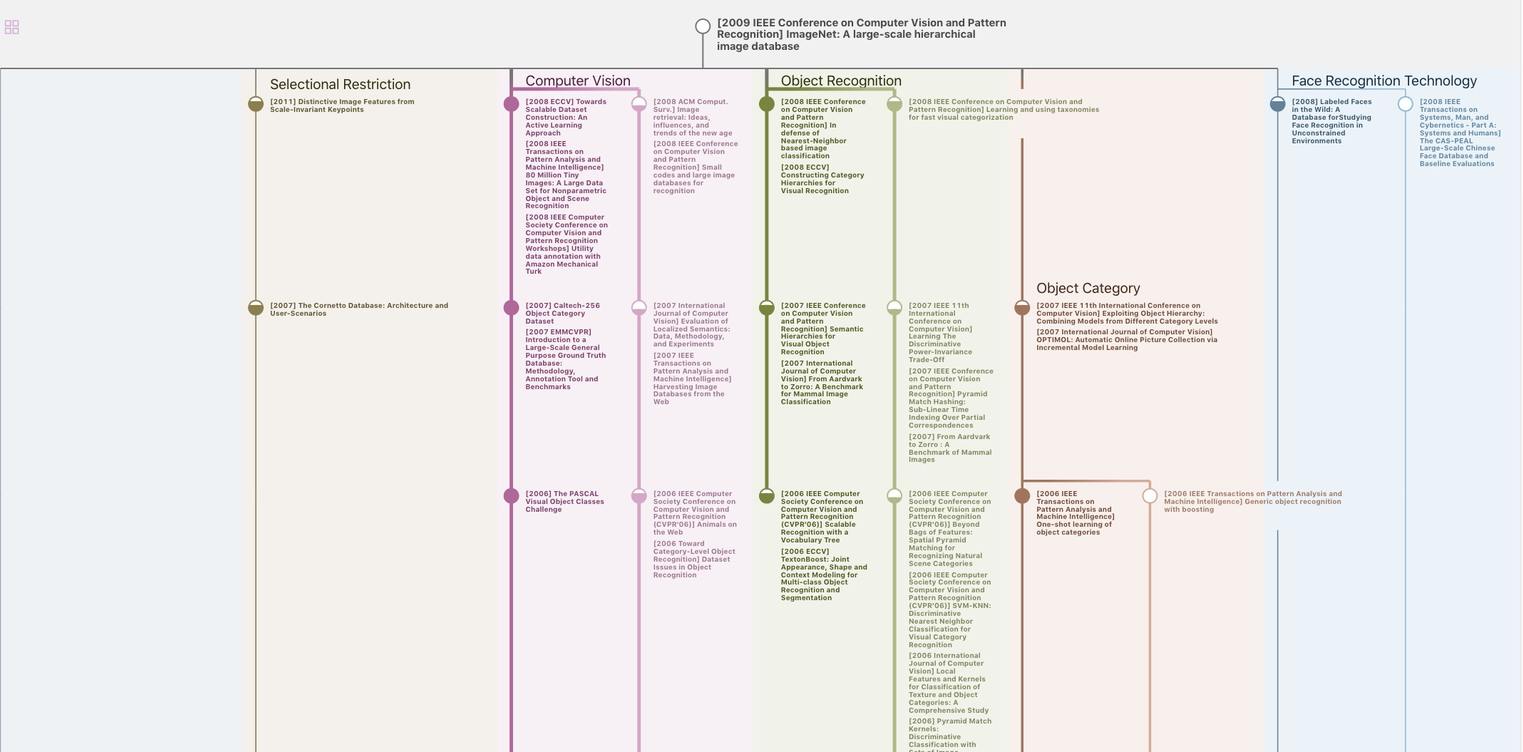
生成溯源树,研究论文发展脉络
Chat Paper
正在生成论文摘要