End to End Trained Long Term Recurrent Convolutional Network for Subject-level Alzheimer Detection
2022 56th Asilomar Conference on Signals, Systems, and Computers(2022)
摘要
Alzheimer's disease early detection and diagnosis is essential for timely treatment of dementia. In this paper, we present an end to end trained long term recurrent convolutional network (LRCN) for Alzheimer's detection from structural MRI (sMRI) images. We employ a 2D slice level CNN for the LRCN model and perform minimal preprocessing on the dataset for our experiments. The proposed model can be divided into two components namely the feature extractor and classifier. The feature extractor consists of the pre-trained network Resnet-18 as backbone followed by a custom 2D convolutional neural network. The features extracted from this network is fed to the classifier which is a recurrent neural network. The dataset used for our experiments was Alzheimer's Disease Neuroimaging Inititative (ADNI). The proposed model was trained for the classification task of Alzheimer's disease (AD) vs control or disease-free (CN) with subject level data division. In subject level data division, 3D sMRI samples were divided into train and test sets and it was ensured that any sample seen by the model during testing was not previously given to the model during training. Hence, subject level division led to an unbiased training of the proposed models. Finally, the results demonstrate promising performance of our model over existing unbiased 2D slice-level model.
更多查看译文
关键词
Alzheimer detection,Transfer Learning,Long term recurrent convolutional network,subject level division
AI 理解论文
溯源树
样例
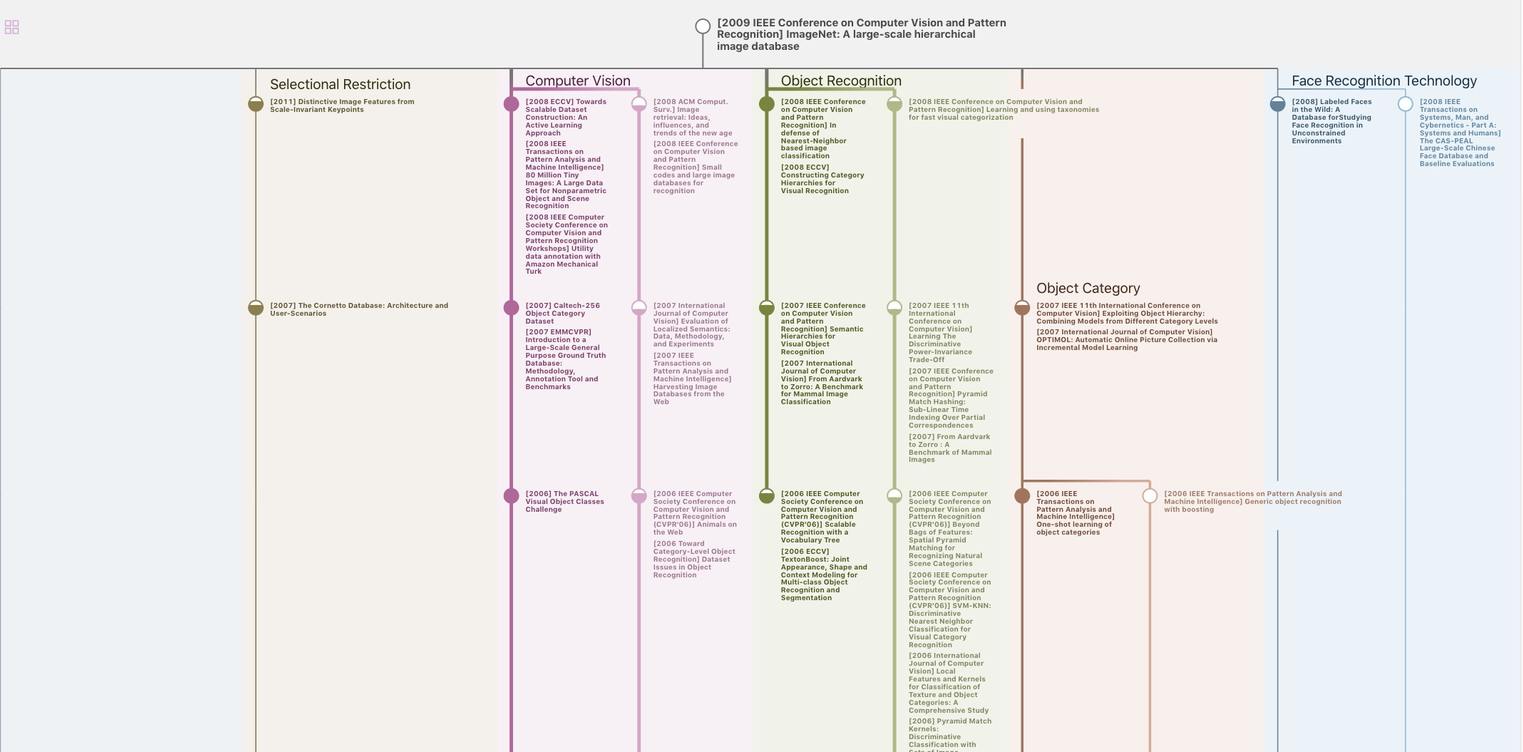
生成溯源树,研究论文发展脉络
Chat Paper
正在生成论文摘要