Clustering large 3D volumes: A sampling-based approach
arxiv(2023)
摘要
In many applications of X-ray computed tomography, an unsupervised segmentation of the reconstructed 3D volumes forms an important step in the image processing chain for further investigation of the digitized object. Therefore, the goal is to train a clustering algorithm on the volume, which produces a voxelwise classification by assigning a cluster index to each voxel. However, clustering methods, e.g., K-Means, typically have an asymptotic polynomial runtime with respect to the dataset size, and thus, these techniques are rarely applicable to large volumes. In this work, we introduce a novel clustering technique based on random sampling, which allows for the voxelwise classification of arbitrarily large volumes. The presented method conducts efficient linear passes over the data to extract a representative random sample of a fixed size on which the classifier can be trained. Then, a final linear pass performs the segmentation and assigns a cluster index to each individual voxel. Quantitative and qualitative evaluations show that excellent results can be achieved even with a very small sample size. Consequently, the unsupervised segmentation by means of clustering becomes feasible for arbitrarily large volumes.
更多查看译文
关键词
large 3d volumes,clustering,sampling-based
AI 理解论文
溯源树
样例
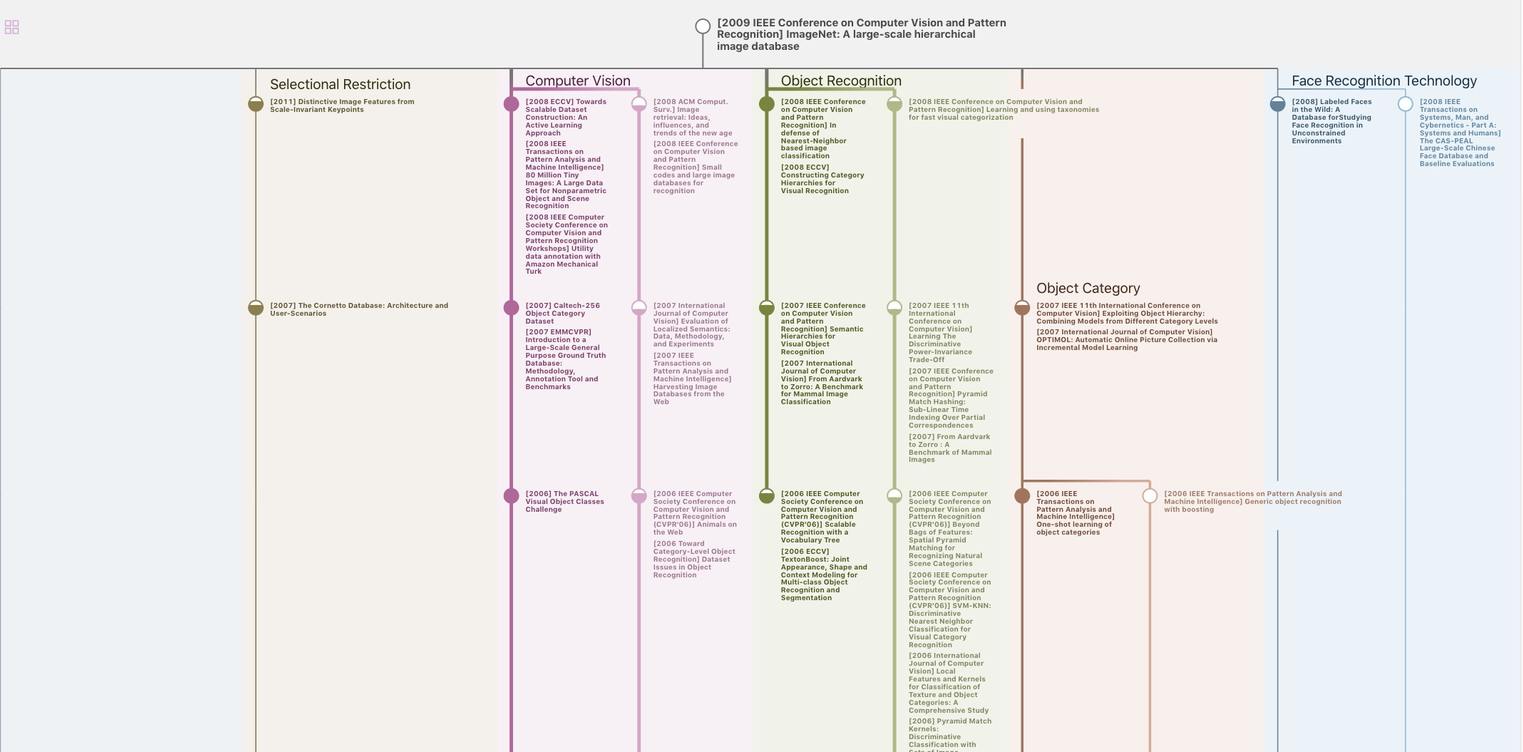
生成溯源树,研究论文发展脉络
Chat Paper
正在生成论文摘要