Polynomial Time and Private Learning of Unbounded Gaussian Mixture Models
arxiv(2023)
摘要
We study the problem of privately estimating the parameters of $d$-dimensional Gaussian Mixture Models (GMMs) with $k$ components. For this, we develop a technique to reduce the problem to its non-private counterpart. This allows us to privatize existing non-private algorithms in a blackbox manner, while incurring only a small overhead in the sample complexity and running time. As the main application of our framework, we develop an $(\varepsilon, \delta)$-differentially private algorithm to learn GMMs using the non-private algorithm of Moitra and Valiant [MV10] as a blackbox. Consequently, this gives the first sample complexity upper bound and first polynomial time algorithm for privately learning GMMs without any boundedness assumptions on the parameters.
更多查看译文
关键词
private learning,unbounded gaussian mixture
AI 理解论文
溯源树
样例
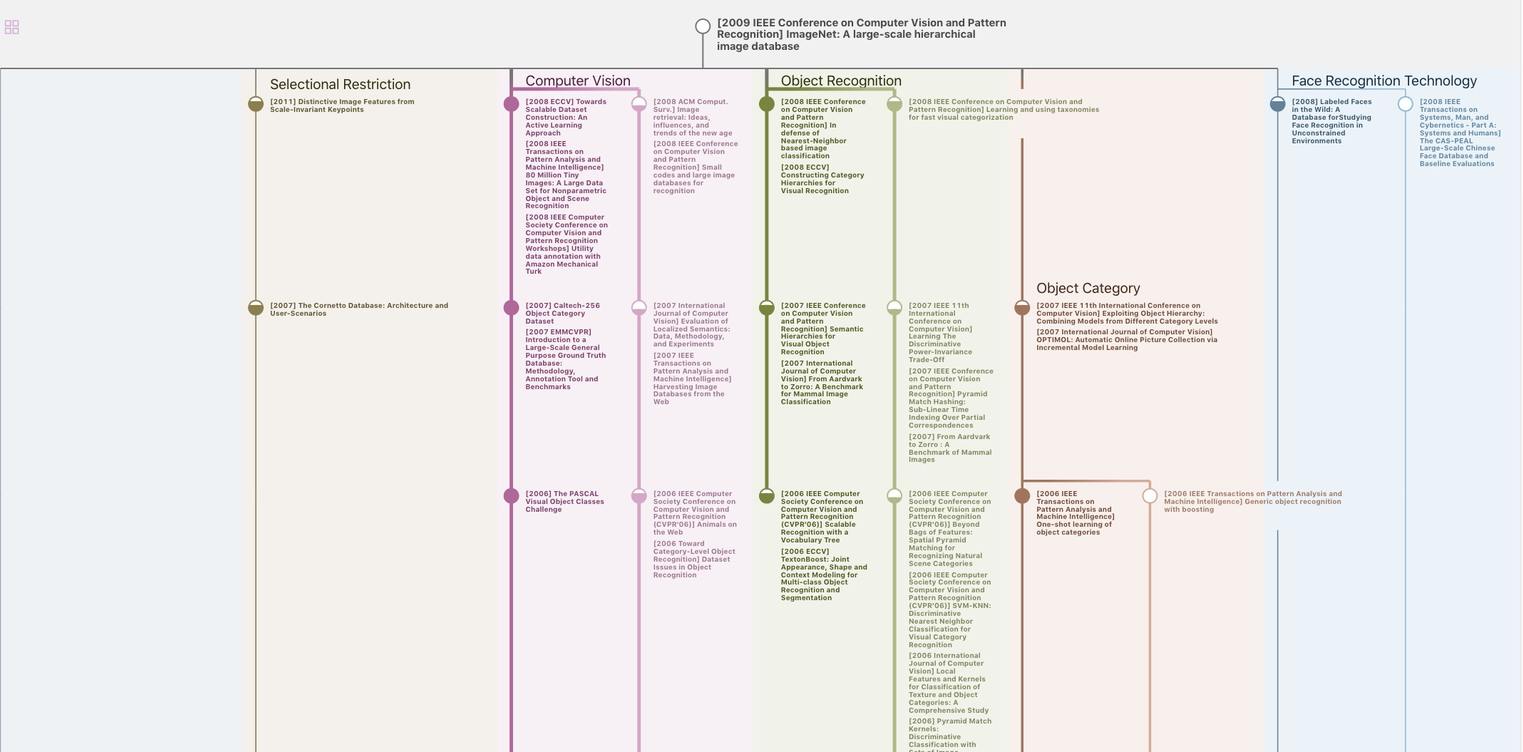
生成溯源树,研究论文发展脉络
Chat Paper
正在生成论文摘要