DULDA: Dual-Domain Unsupervised Learned Descent Algorithm for PET Image Reconstruction
arxiv(2023)
摘要
Deep learning based PET image reconstruction methods have achieved promising results recently. However, most of these methods follow a supervised learning paradigm, which rely heavily on the availability of high-quality training labels. In particular, the long scanning time required and high radiation exposure associated with PET scans make obtaining these labels impractical. In this paper, we propose a dual-domain unsupervised PET image reconstruction method based on learned descent algorithm, which reconstructs high-quality PET images from sinograms without the need for image labels. Specifically, we unroll the proximal gradient method with a learnable l(2,1) norm for PET image reconstruction problem. The training is unsupervised, using measurement domain loss based on deep image prior as well as image domain loss based on rotation equivariance property. The experimental results demonstrate the superior performance of proposed method compared with maximum-likelihood expectation-maximization (MLEM), total-variation regularized EM (EM-TV) and deep image prior based method (DIP).
更多查看译文
关键词
Image reconstruction,Positron emission tomography (PET),Unsupervised learning,Model based deep learning,Dual-domain
AI 理解论文
溯源树
样例
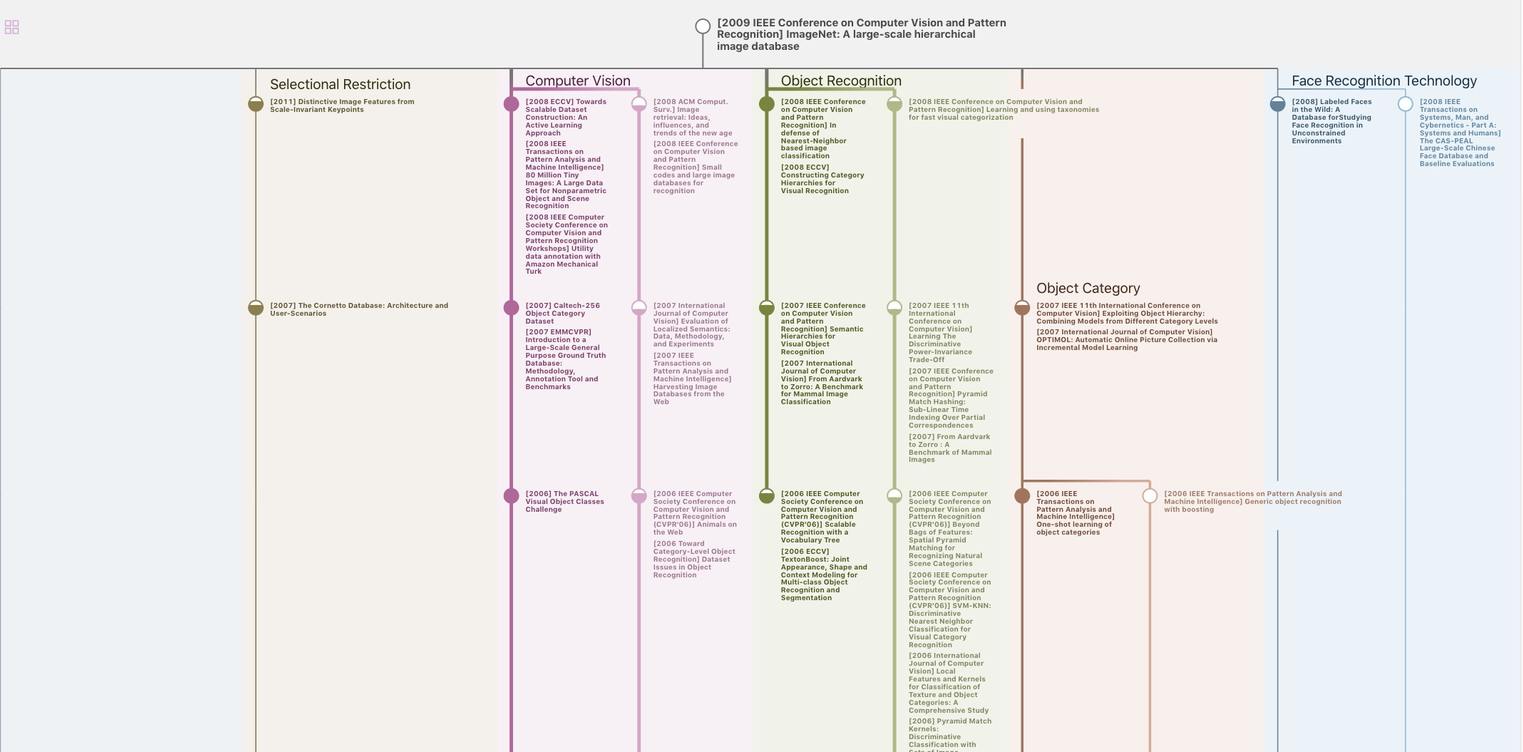
生成溯源树,研究论文发展脉络
Chat Paper
正在生成论文摘要