The Bystander Affect Detection (BAD) Dataset for Failure Detection in HRI
2023 IEEE/RSJ INTERNATIONAL CONFERENCE ON INTELLIGENT ROBOTS AND SYSTEMS (IROS)(2023)
摘要
For a robot to repair its own error, it must first know it has made a mistake. One way that people detect errors is from the implicit reactions from bystanders - their confusion, smirks, or giggles clue us in that something unexpected occurred. To enable robots to detect and act on bystander responses to task failures, we developed a novel method to elicit bystander responses to human and robot errors. Using 46 different stimulus videos featuring a variety of human and machine task failures, we collected a total of 2,452 webcam videos of human reactions from 54 participants. To test the viability of the collected data, we used the bystander reaction dataset as input to a deep-learning model, BADNet, to predict failure occurrence. We tested different data labeling methods and learned how they affect model performance, achieving precisions above 90%. We discuss strategies (manual labelling, failure-vs-control, and failure-time) used to model bystander reactions and predict failure, and how this approach can be used in real-world robotic deployments to detect errors and improve robot performance. As part of this work, we also contribute with the "Bystander Affect Detection" (BAD) dataset of bystander reactions, supporting the development of better prediction models.
更多查看译文
关键词
affective computing,robot error,human response dataset
AI 理解论文
溯源树
样例
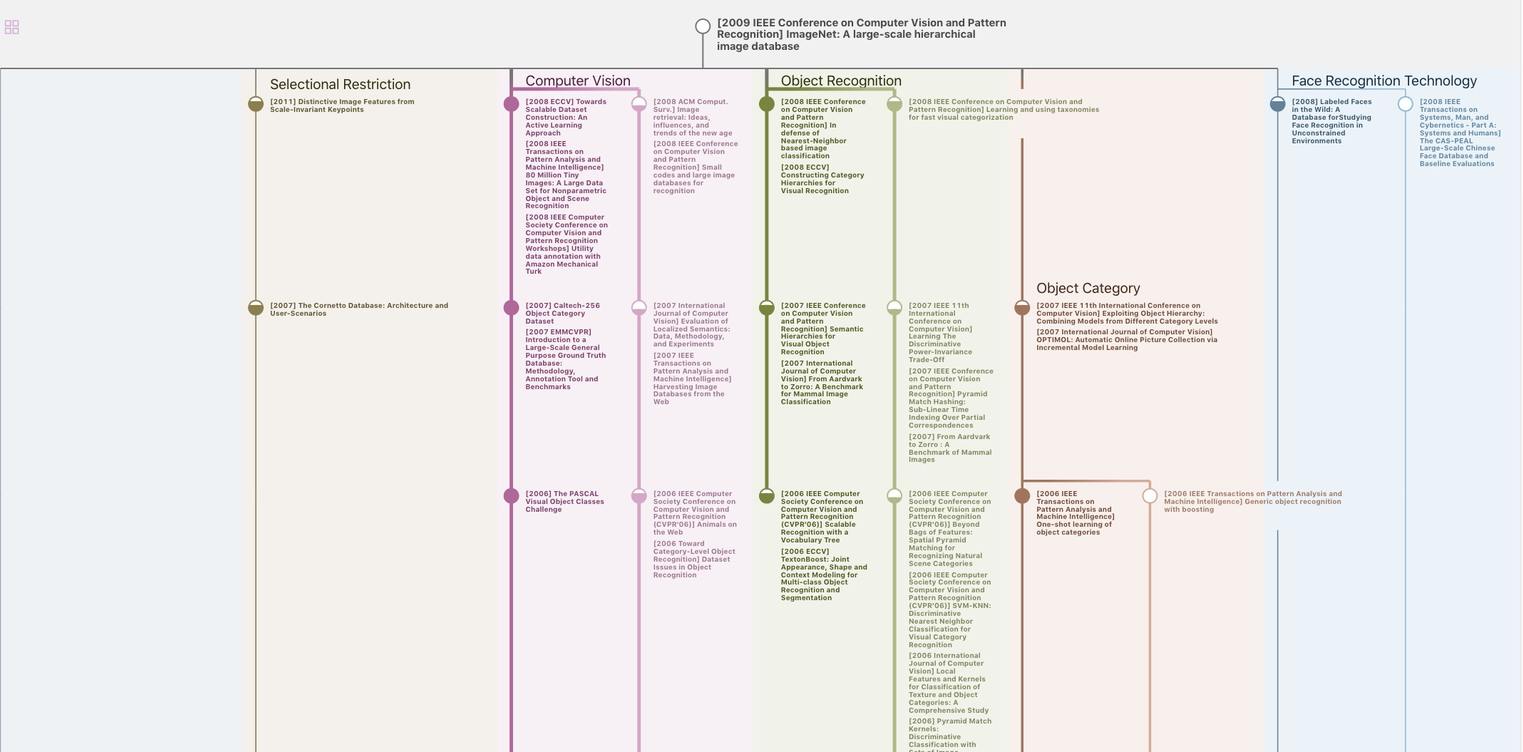
生成溯源树,研究论文发展脉络
Chat Paper
正在生成论文摘要