Smooth and Stepwise Self-Distillation for Object Detection
arxiv(2023)
摘要
Distilling the structured information captured in feature maps has contributed to improved results for object detection tasks, but requires careful selection of baseline architectures and substantial pre-training. Self-distillation addresses these limitations and has recently achieved state-of-the-art performance for object detection despite making several simplifying architectural assumptions. Building on this work, we propose Smooth and Stepwise Self-Distillation (SSSD) for object detection. Our SSSD architecture forms an implicit teacher from object labels and a feature pyramid network backbone to distill label-annotated feature maps using Jensen-Shannon distance, which is smoother than distillation losses used in prior work. We additionally add a distillation coefficient that is adaptively configured based on the learning rate. We extensively benchmark SSSD against a baseline and two state-of-the-art object detector architectures on the COCO dataset by varying the coefficients and backbone and detector networks. We demonstrate that SSSD achieves higher average precision in most experimental settings, is robust to a wide range of coefficients, and benefits from our stepwise distillation procedure.
更多查看译文
关键词
knowledge distillation,object detection,Jensen-Shannon distance,stepwise distillation
AI 理解论文
溯源树
样例
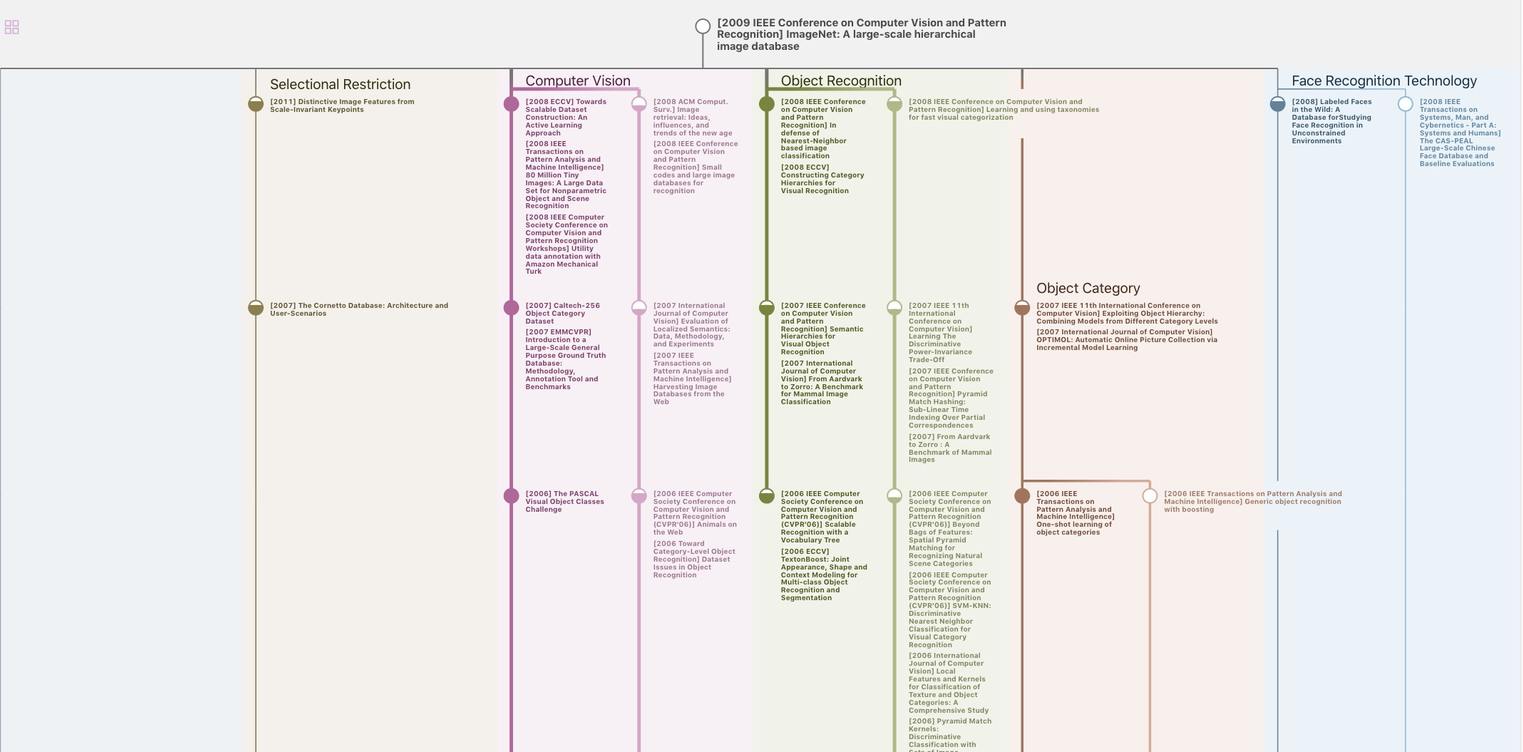
生成溯源树,研究论文发展脉络
Chat Paper
正在生成论文摘要