Invertible Kernel PCA with Random Fourier Features
arxiv(2023)
摘要
Kernel principal component analysis (kPCA) is a widely studied method to construct a low-dimensional data representation after a nonlinear transformation. The prevailing method to reconstruct the original input signal from kPCA -- an important task for denoising -- requires us to solve a supervised learning problem. In this paper, we present an alternative method where the reconstruction follows naturally from the compression step. We first approximate the kernel with random Fourier features. Then, we exploit the fact that the nonlinear transformation is invertible in a certain subdomain. Hence, the name \emph{invertible kernel PCA (ikPCA)}. We experiment with different data modalities and show that ikPCA performs similarly to kPCA with supervised reconstruction on denoising tasks, making it a strong alternative.
更多查看译文
关键词
random fourier features
AI 理解论文
溯源树
样例
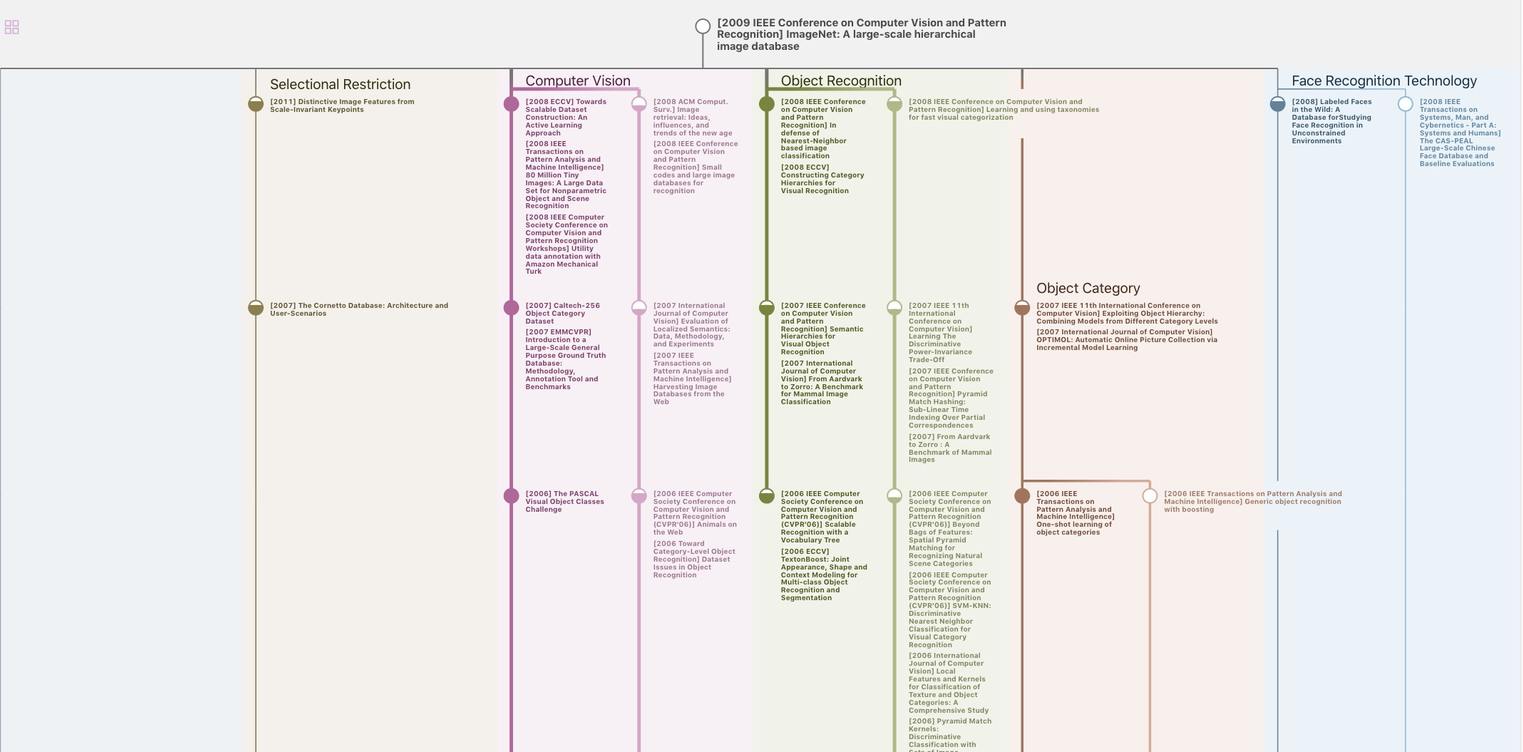
生成溯源树,研究论文发展脉络
Chat Paper
正在生成论文摘要