Annotator-dependent uncertainty-aware estimation of gait relative attributes
Pattern Recognition(2023)
摘要
In this paper, we describe an uncertainty-aware estimation framework for gait relative attributes. We specifically design a two-stream network model that takes a pair of gait videos as input. It then outputs a corresponding pair of Gaussian distributions of gait absolute attribute scores and annotator-dependent gait relative attribute label distributions. Moreover, we propose a differentiable annotator-independent uncertainty layer to estimate the gait relative attribute score distribution from the absolute distributions then map it to a relative attribute label distribution using the computation of cumulative distribution functions. Furthermore, we propose another annotator-dependent uncertainty layer to estimate the un-certainty on the gait relative attribute labels in terms of a set of trainable transition matrices. Finally, we design a joint loss function on the relative attribute label distribution to learn the model parameters. Ex-periments on two gait relative attribute datasets demonstrated the effectiveness of the proposed method against baselines in quantitative and qualitative evaluations. (c) 2022 The Authors. Published by Elsevier Ltd. This is an open access article under the CC BY-NC-ND license ( http://creativecommons.org/licenses/by-nc-nd/4.0/ )
更多查看译文
关键词
Gait relative attribute,Relative label distribution,Relative score distribution,Annotator?s uncertainty,Transition matrix
AI 理解论文
溯源树
样例
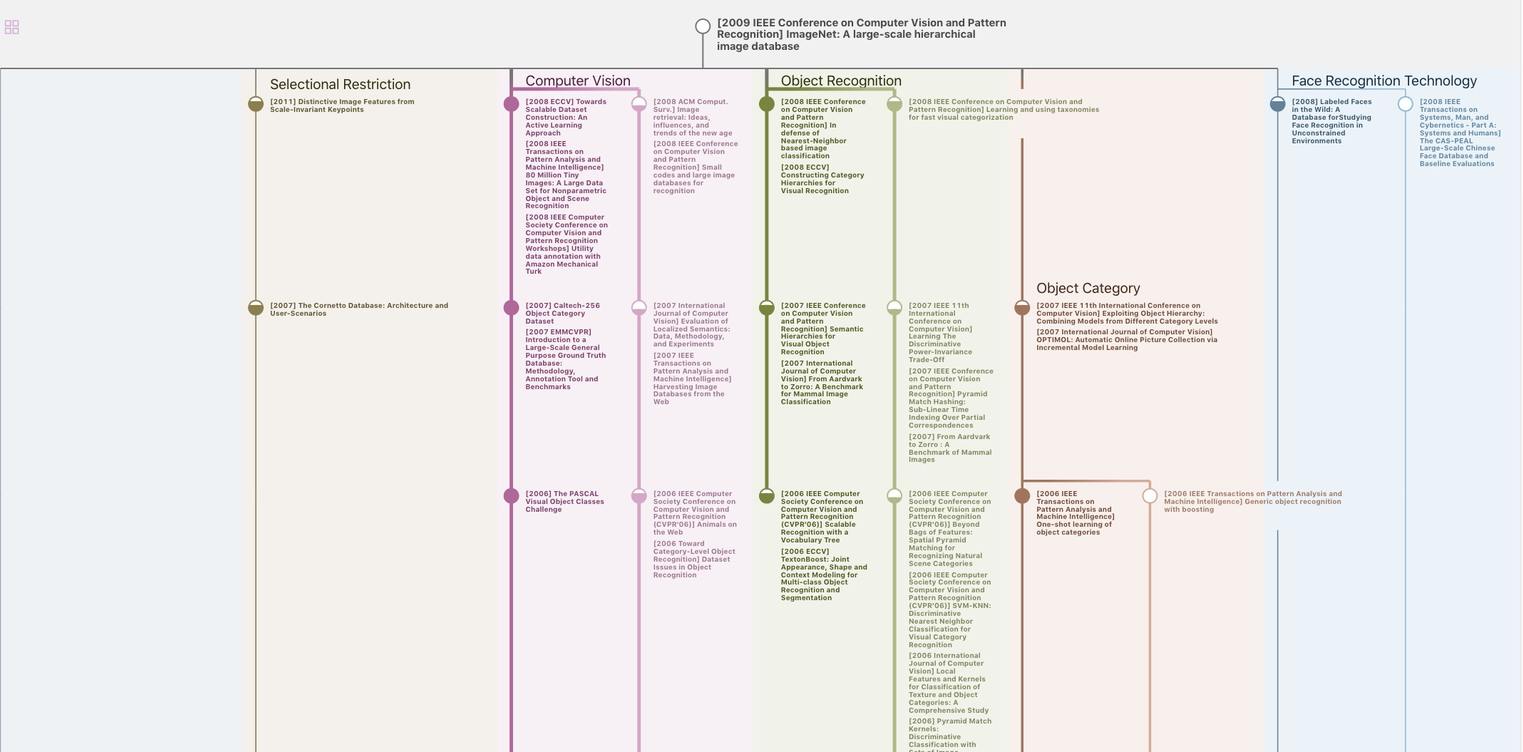
生成溯源树,研究论文发展脉络
Chat Paper
正在生成论文摘要