Spectral classification of LAMOST emission line galaxies based on machine learning methods
New Astronomy(2023)
摘要
Spectral classification of emission-line galaxies(ELGs) plays an important role to understand the formation and evolution of different galaxies. Machine learning can obtain the ability of spectrum recognition by learning the features of a large number of spectra so as to automatically classify unlabeled spectra. We apply several machine learning methods: multi-layer perception (MLP), support vector machine (SVM), K-nearest neighbor(KNN) and random forest(RF) for classifying 49,000 emission-line galaxies observed by LAMOST(Large Sky Area Multi-Object Fiber Spectroscopic Telescope). In classification process, we directly employ the spectral flux pixels around some frequently used emission lines(H beta, [OIII]. lambda lambda 4959,5007, [OI], H alpha, [NII]lambda lambda 6548,6584 and [SII]lambda lambda.6717,6731) as feature space. By comparing four algorithms, MLP classifier has the highest accuracy of 92.31%. In addition, we confirm the robustness of our MLP classifier. Finally, we provide our MLP classifier for classifying the emission-line galaxies in LAMOST new observations.
更多查看译文
关键词
Machine learning,Spectral classification,LAMOST,Data analysis
AI 理解论文
溯源树
样例
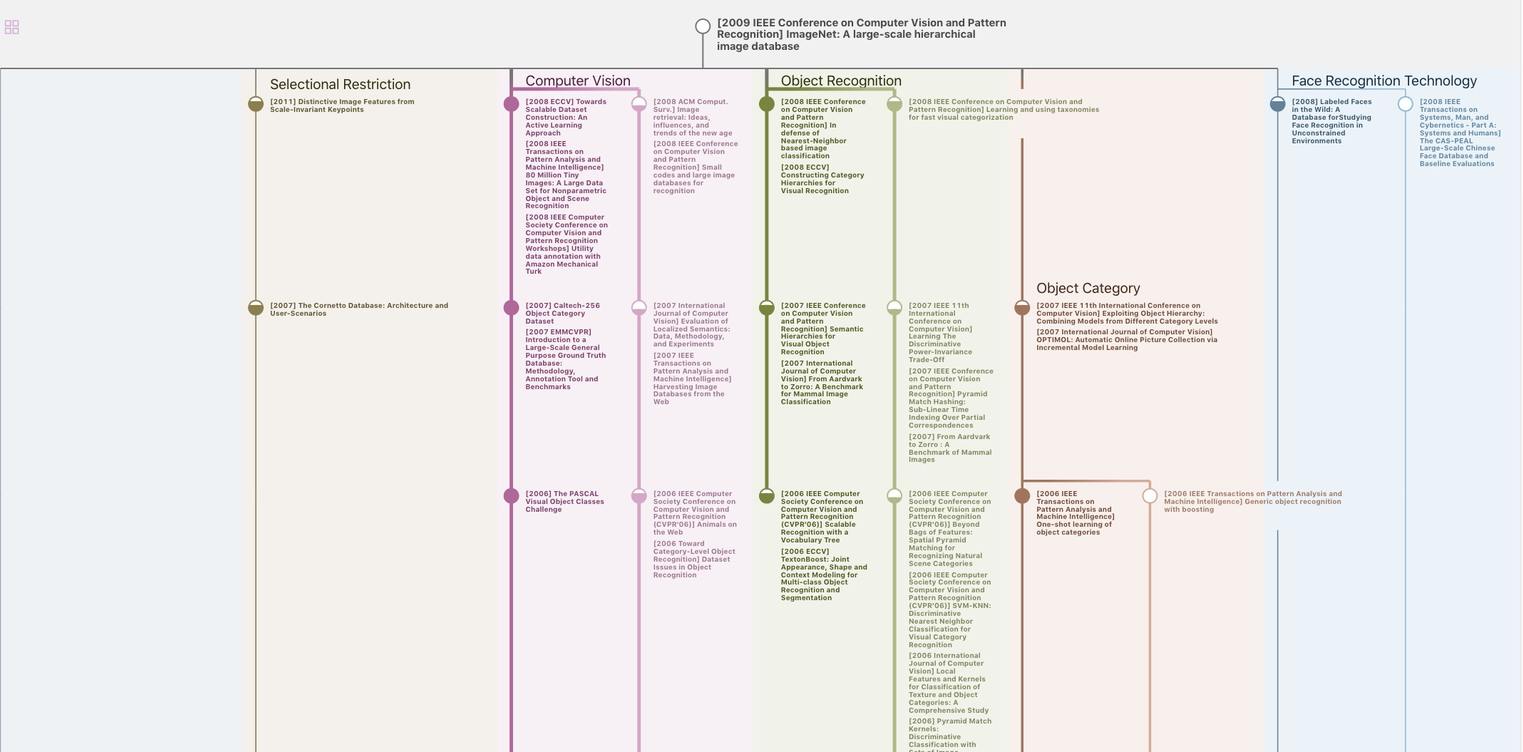
生成溯源树,研究论文发展脉络
Chat Paper
正在生成论文摘要