A methodology to identify identical single-board computers based on hardware behavior fingerprinting
Journal of Network and Computer Applications(2023)
摘要
The connectivity and resource-constrained nature of single-board devices open the door to cybersecurity concerns affecting Internet of Things (IoT) scenarios. One of the most important issues is the presence of unauthorized IoT devices that want to impersonate legitimate ones by using identical hardware and software specifications. This situation can provoke sensitive information leakages, data poisoning, or privilege escalation in IoT scenarios. Combining behavioral fingerprinting and Machine/Deep Learning (ML/DL) techniques is a promising approach to identify these malicious spoofing devices by detecting minor performance differences generated by imperfections in manufacturing. However, existing solutions are not suitable for single-board devices since they do not consider their hardware and software limitations, underestimate critical aspects such as fingerprint stability or context changes, and do not explore the potential of ML/DL techniques. To improve it, this work first identifies the essential properties for single-board device identification: uniqueness, stability, diversity, scalability, efficiency, robustness, and security. Then, a novel methodology relies on behavioral fingerprinting to identify identical single-board devices and meet the previous properties. The methodology leverages the different built-in components of the system and ML/DL techniques, comparing the device internal behavior with each other to detect variations that occurred in manufacturing processes. The methodology validation has been performed in a real environment composed of 15 identical Raspberry Pi 4 Model B and 10 Raspberry Pi 3 Model B+ devices, obtaining a 91.9% average TPR with an XGBoost model and achieving the identification for all devices by setting a 50% threshold in the evaluation process. Finally, a discussion compares the proposed solution with related work, highlighting the fingerprint properties not met, and provides important lessons learned and limitations.
更多查看译文
关键词
Device behavior fingerprinting,Device identification,Cyberattack detection,Behavioral data,Hardware fingerprinting
AI 理解论文
溯源树
样例
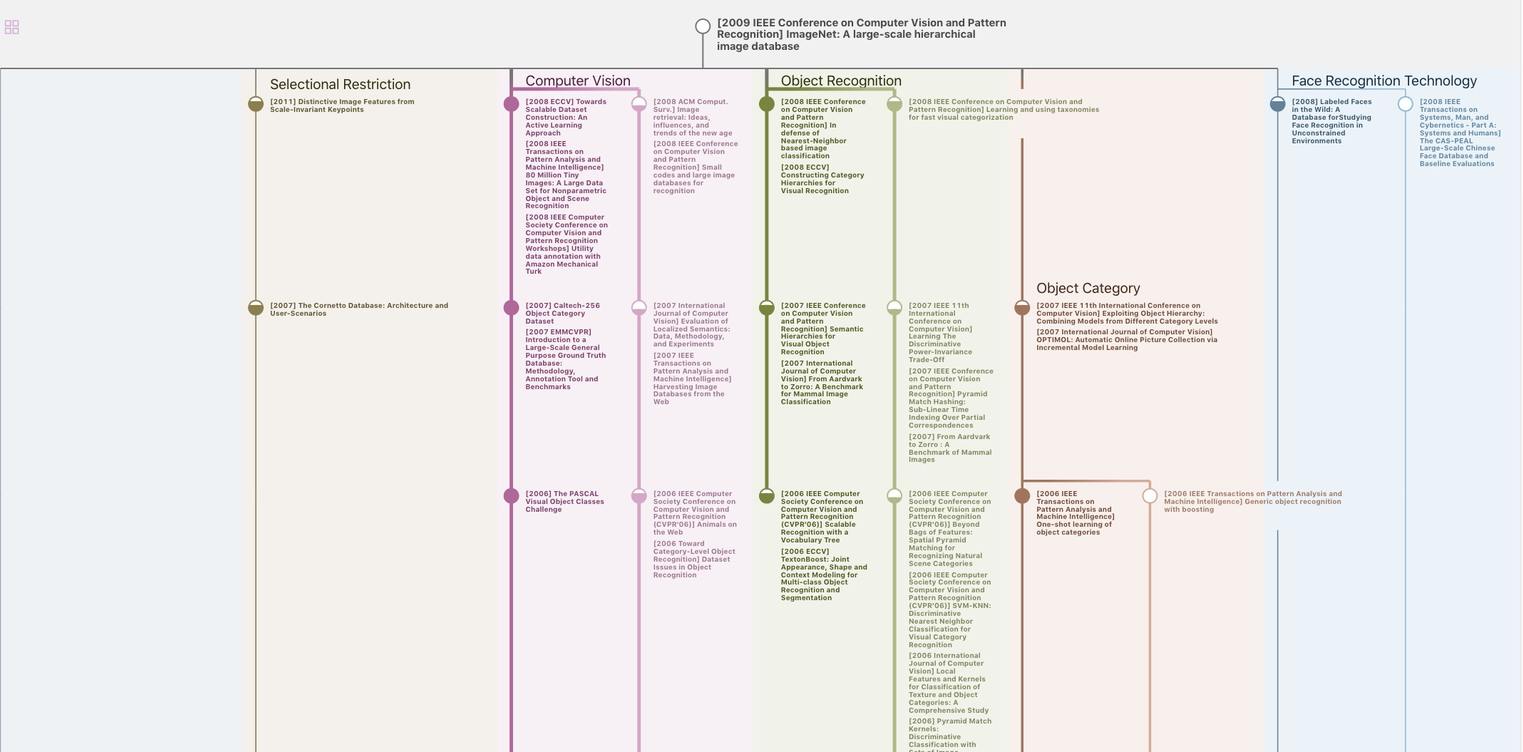
生成溯源树,研究论文发展脉络
Chat Paper
正在生成论文摘要