Rapid urban flood risk mapping for data-scarce environments using social sensing and region-stable deep neural network
Journal of Hydrology(2023)
摘要
Urban flooding is one of the most widespread natural hazards in modern cities. Risk mapping provides critical information for flood risk management to reduce life and economic loss. As a widely used approach to support flood risk mapping, physical-based modeling suffers from model accuracy and computation complexity. Empirical methods rely on availability of rich disaster data and are not normally transferable for fast flood prediction to different cities. Both methods require high-quality hazard data and disaster information for reliable prediction, which are not always available. This paper presents an alternative near real-time flood risk mapping method for data scarce environments developed using social sensing and region-stable deep neural network (RS-DNN). By extracting disaster information in near real-time using social sensing techniques and considering risk distribution factors rather than flood influencing factors, this new method enables flood risk mapping and analysis cross a large domain in minutes, with all input data openly available. The proposed method can be adapted to different disaster process and different case study cities through timely social sensing.
更多查看译文
关键词
Data scarcity,Flood risk mapping,Region-stable DNN,Risk distribution factors,Social sensing
AI 理解论文
溯源树
样例
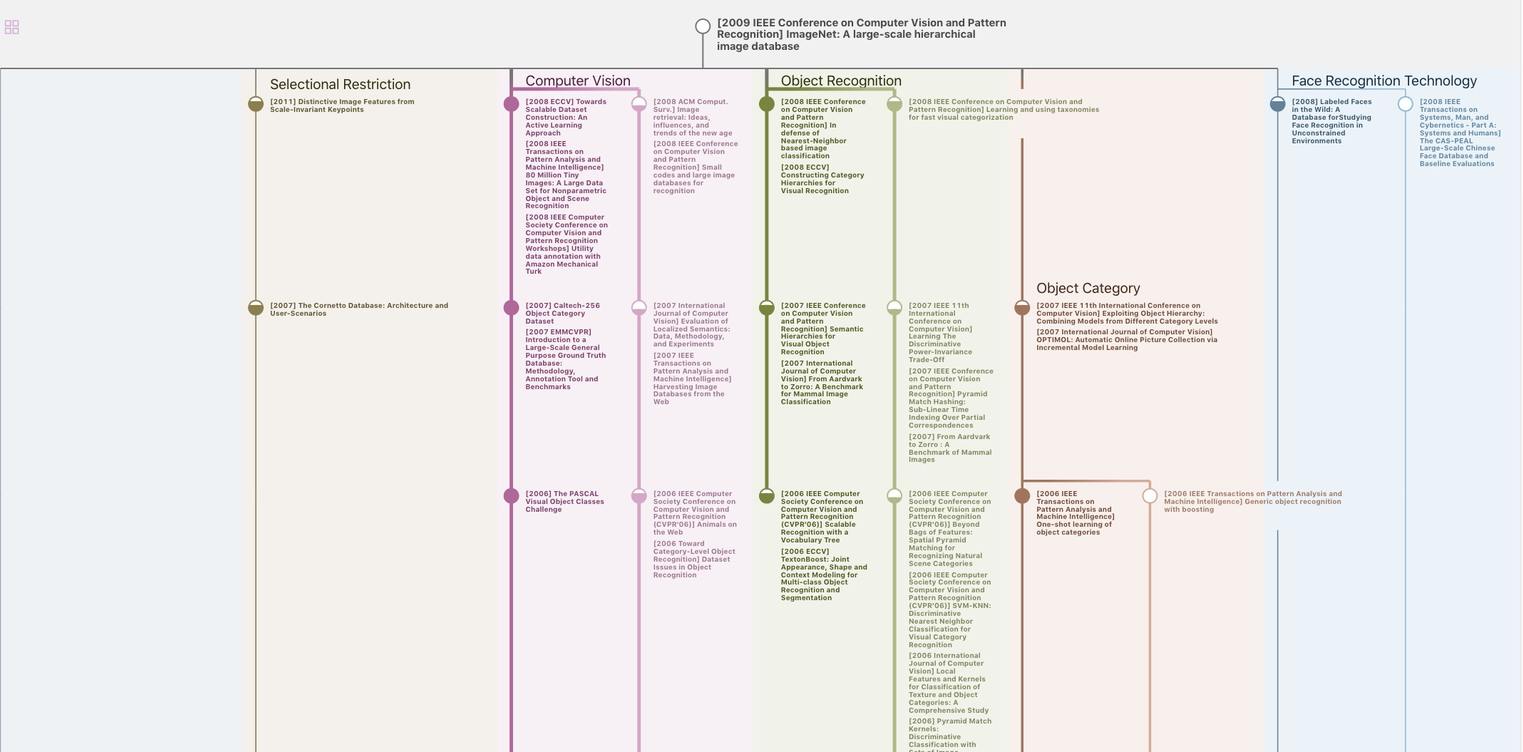
生成溯源树,研究论文发展脉络
Chat Paper
正在生成论文摘要