Deep-learning-based ring artifact correction for tomographic reconstruction.
Journal of synchrotron radiation(2023)
摘要
X-ray tomography has been widely used in various research fields thanks to its capability of observing 3D structures with high resolution non-destructively. However, due to the nonlinearity and inconsistency of detector pixels, ring artifacts usually appear in tomographic reconstruction, which may compromise image quality and cause nonuniform bias. This study proposes a new ring artifact correction method based on the residual neural network (ResNet) for X-ray tomography. The artifact correction network uses complementary information of each wavelet coefficient and a residual mechanism of the residual block to obtain high-precision artifacts through low operation costs. In addition, a regularization term is used to accurately extract stripe artifacts in sinograms, so that the network can better preserve image details while accurately separating artifacts. When applied to simulation and experimental data, the proposed method shows a good suppression of ring artifacts. To solve the problem of insufficient training data, ResNet is trained through the transfer learning strategy, which brings advantages of robustness, versatility and low computing cost.
更多查看译文
关键词
X-ray tomography,deep learning,residual neural network,ring artifact correction
AI 理解论文
溯源树
样例
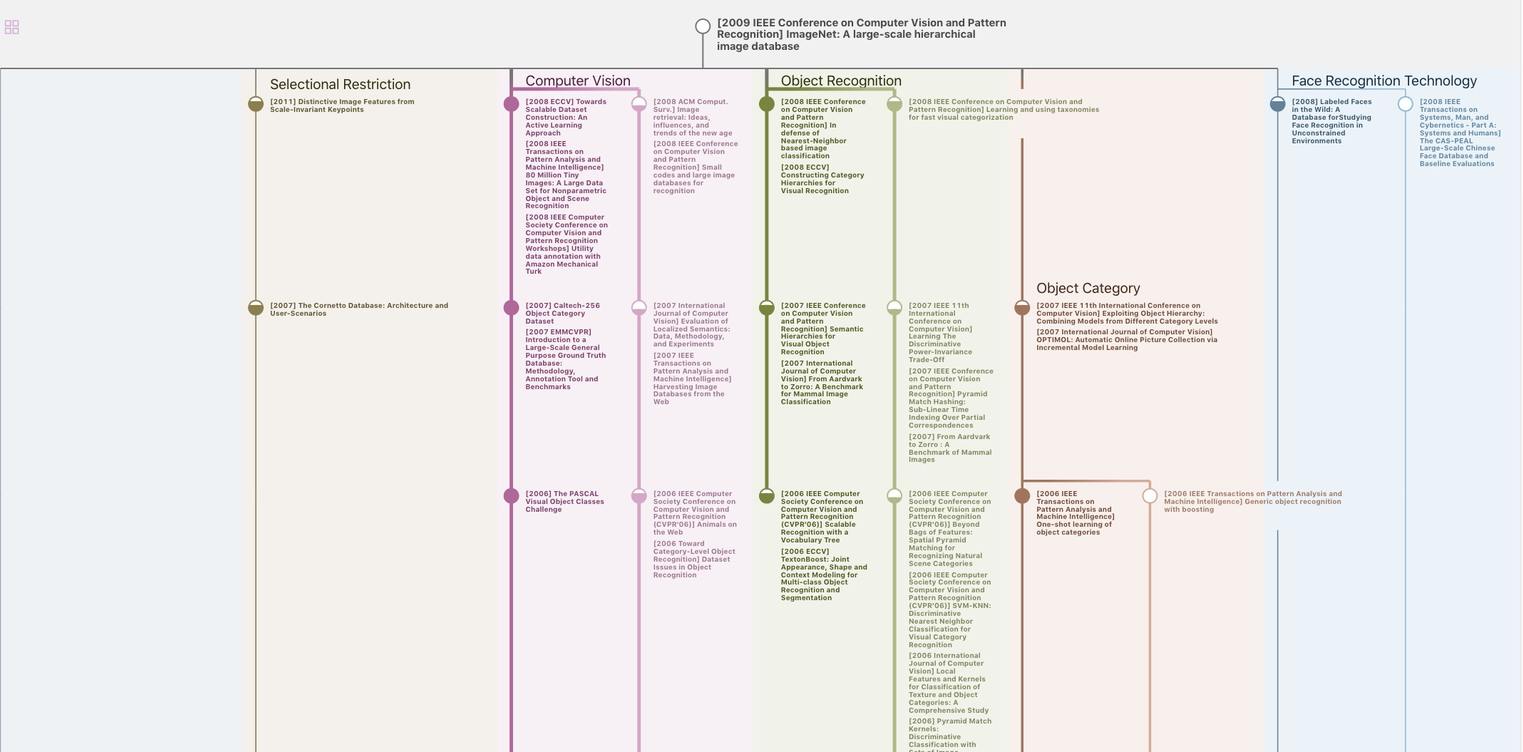
生成溯源树,研究论文发展脉络
Chat Paper
正在生成论文摘要