DRL-Assisted Reoptimization of Network Slice Embedding on EON-Enabled Transport Networks
IEEE Transactions on Network and Service Management(2023)
摘要
5G transport networks will support dynamic services with diverse requirements through network slicing. Elastic Optical Networks (EONs) facilitate transport network slicing by flexible spectrum allocation and tuning of transmission configurations. A major challenge in supporting dynamic services is the lack of priori knowledge of future slice requests. As a consequence, slice embedding can become sub-optimal over time, leading to spectrum fragmentation and skewed utilization. This in turn can block future slice requests, impacting operator revenue. To address this issue, operators can periodically re-optimize slice embedding for reducing fragmentation. In this paper, we address this problem of re-optimizing network slice embedding on EONs for minimizing fragmentation. The problem is solved in its splittable version, which significantly increases problem complexity, but also offers more opportunities for a larger set of re-configuration actions. We employ simulated annealing for systematically exploring the large solution space. We also propose a greedy algorithm to address the practical constraint of limiting the number of re-configuration steps. Moreover, we present a novel method based on Deep Reinforcement Learning (DRL) for determining when performing re-configuration is most effective. Our extensive simulations demonstrate that the greedy algorithm yields a solution very close to that obtained using simulated annealing while requiring orders of magnitude lesser re-configuration actions. Finally, we show that by applying the greedy algorithm periodically on the network according to the DRL-based time selection algorithm, a significant improvement in the total number of accepted slice requests can be achieved with only performing a limited number of re-configuration operations.
更多查看译文
关键词
Elastic optical network,fragmentation,deep reinforcement learning (DRL),transport network
AI 理解论文
溯源树
样例
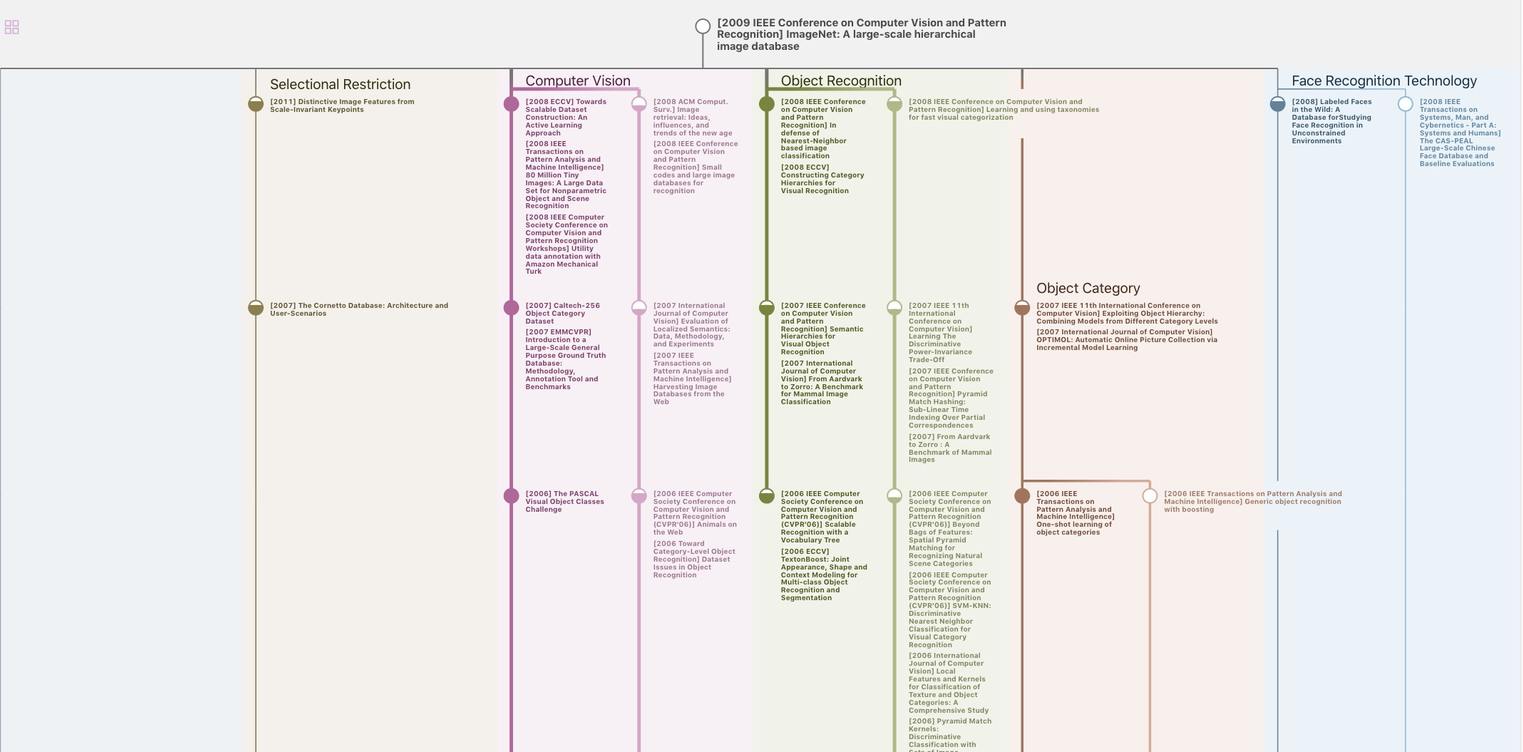
生成溯源树,研究论文发展脉络
Chat Paper
正在生成论文摘要