XGBoost-Based Simple Three-Item Model Accurately Predicts Outcomes of Acute Ischemic Stroke.
Diagnostics (Basel, Switzerland)(2023)
摘要
An all-inclusive and accurate prediction of outcomes for patients with acute ischemic stroke (AIS) is crucial for clinical decision-making. This study developed extreme gradient boosting (XGBoost)-based models using three simple factors-age, fasting glucose, and National Institutes of Health Stroke Scale (NIHSS) scores-to predict the three-month functional outcomes after AIS. We retrieved the medical records of 1848 patients diagnosed with AIS and managed at a single medical center between 2016 and 2020. We developed and validated the predictions and ranked the importance of each variable. The XGBoost model achieved notable performance, with an area under the curve of 0.8595. As predicted by the model, the patients with initial NIHSS score > 5, aged over 64 years, and fasting blood glucose > 86 mg/dL were associated with unfavorable prognoses. For patients receiving endovascular therapy, fasting glucose was the most important predictor. The NIHSS score at admission was the most significant predictor for those who received other treatments. Our proposed XGBoost model showed a reliable predictive power of AIS outcomes using readily available and simple predictors and also demonstrated the validity of the model for application in patients receiving different AIS treatments, providing clinical evidence for future optimization of AIS treatment strategies.
更多查看译文
关键词
NIHSS,XGBoost,acute ischemic stroke,glucose,machine learning,prognosis
AI 理解论文
溯源树
样例
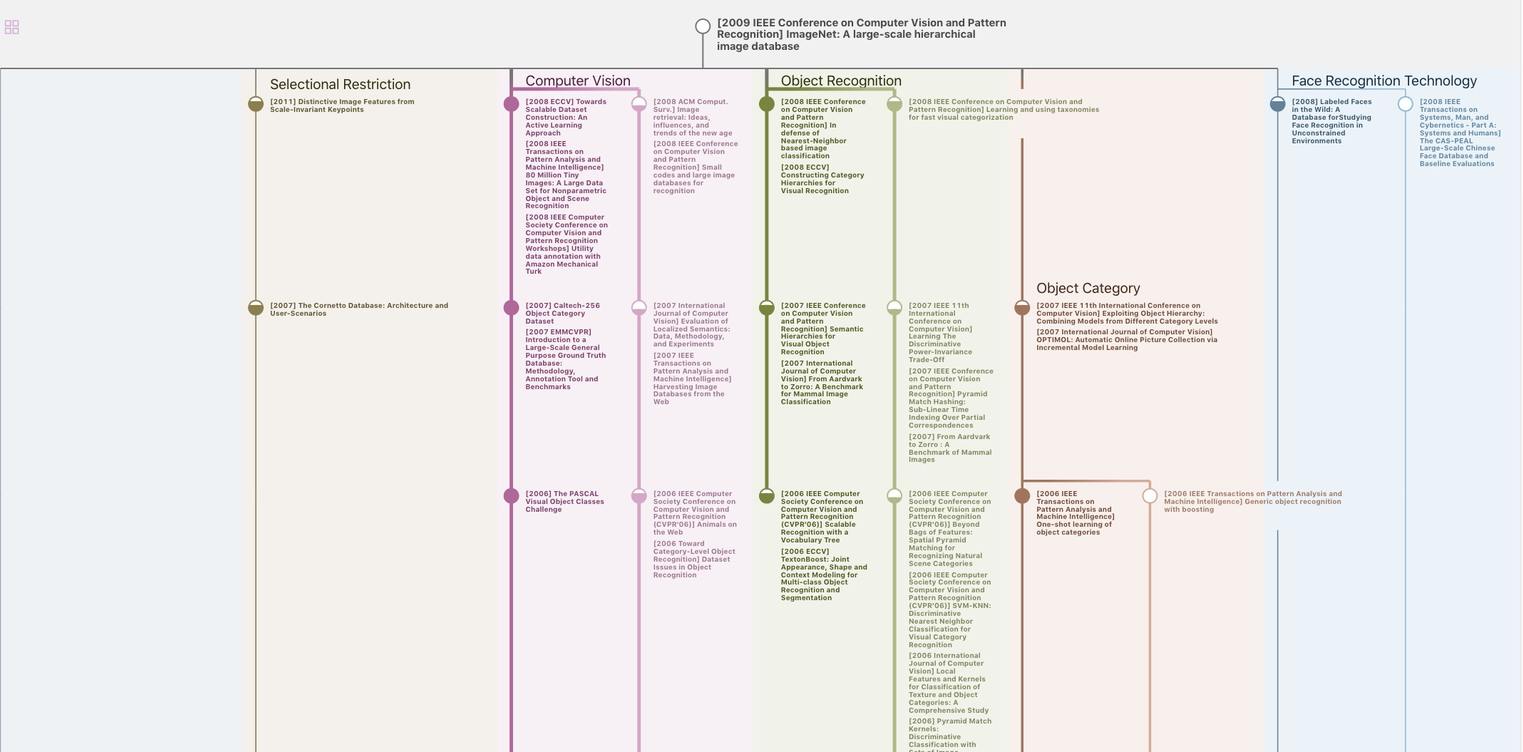
生成溯源树,研究论文发展脉络
Chat Paper
正在生成论文摘要