A Biterm Topic Model for Sparse Mutation Data.
Cancers(2023)
摘要
Mutational signature analysis promises to reveal the processes that shape cancer genomes for applications in diagnosis and therapy. However, most current methods are geared toward rich mutation data that has been extracted from whole-genome or whole-exome sequencing. Methods that process sparse mutation data typically found in practice are only in the earliest stages of development. In particular, we previously developed the Mix model that clusters samples to handle data sparsity. However, the Mix model had two hyper-parameters, including the number of signatures and the number of clusters, that were very costly to learn. Therefore, we devised a new method that was several orders-of-magnitude more efficient for handling sparse data, was based on mutation co-occurrences, and imitated word co-occurrence analyses of Twitter texts. We showed that the model produced significantly improved hyper-parameter estimates that led to higher likelihoods of discovering overlooked data and had better correspondence with known signatures.
更多查看译文
关键词
biterm topic model,mutational signature,panel sequencing data
AI 理解论文
溯源树
样例
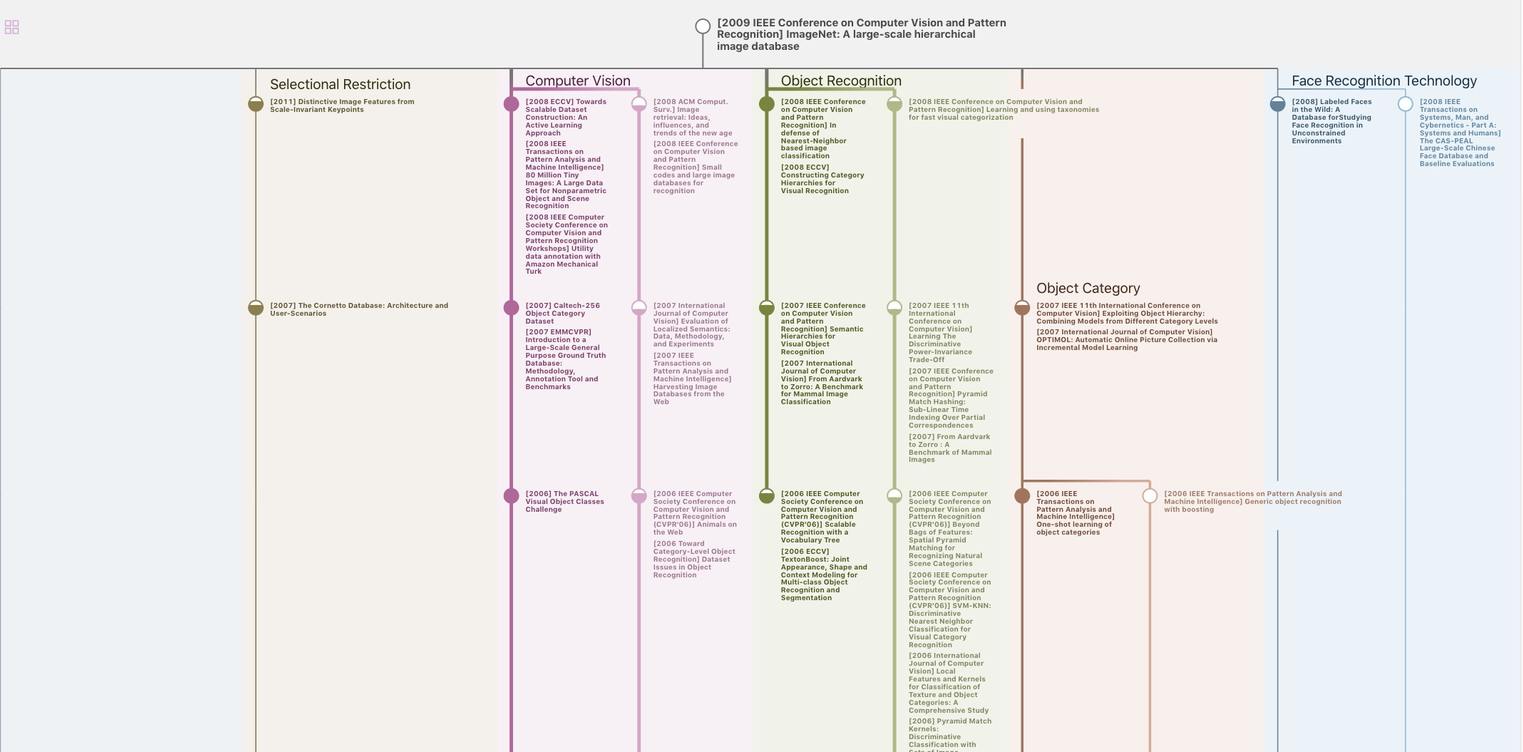
生成溯源树,研究论文发展脉络
Chat Paper
正在生成论文摘要