CGMA-Net: Cross-Level Guidance and Multi-Scale Aggregation Network for Polyp Segmentation
IEEE JOURNAL OF BIOMEDICAL AND HEALTH INFORMATICS(2024)
摘要
Colonoscopy is considered the best prevention and control method for colorectal cancer, which suffers extremely high rates of mortality and morbidity. Automated polyp segmentation of colonoscopy images is of great importance since manual polyp segmentation requires a considerable time of experienced specialists. However, due to the high similarity between polyps and mucosa, accompanied by the complex morphological features of colonic polyps, the performance of automatic polyp segmentation is still unsatisfactory. Accordingly, we propose a network, namely Cross-level Guidance and Multi-scale Aggregation (CGMA-Net), to earn a performance promotion. Specifically, three modules, including Cross-level Feature Guidance (CFG), Multi-scale Aggregation Decoder (MAD), and Details Refinement (DR), are individually proposed and synergistically assembled. With CFG, we generate spatial attention maps from the higher-level features and then multiply them with the lower-level features, highlighting the region of interest and suppressing the background information. In MAD, we parallelly use multiple dilated convolutions of different sizes to capture long-range dependencies between features. For DR, an asynchronous convolution is used along with the attention mechanism to enhance both the local details and the global information. The proposed CGMA-Net is evaluated on two benchmark datasets, i.e., CVC-ClinicDB and Kvasir-SEG, whose results demonstrate that our method not only presents state-of-the-art performance but also holds relatively fewer parameters. Concretely, we achieve the Dice Similarity Coefficient (DSC) of 91.85% and 95.73% on Kvasir-SEG and CVC-ClinicDB, respectively. The assessment of model generalization is also conducted, resulting in DSC scores of 86.25% and 86.97% on the two datasets respectively.
更多查看译文
关键词
Image segmentation,Semantics,Feature extraction,Transformers,Decoding,Data mining,Bioinformatics,Polyp segmentation,cross-level feature guidance (CFG),multi-scale feature aggregation,cross interaction,details refinement (DR)
AI 理解论文
溯源树
样例
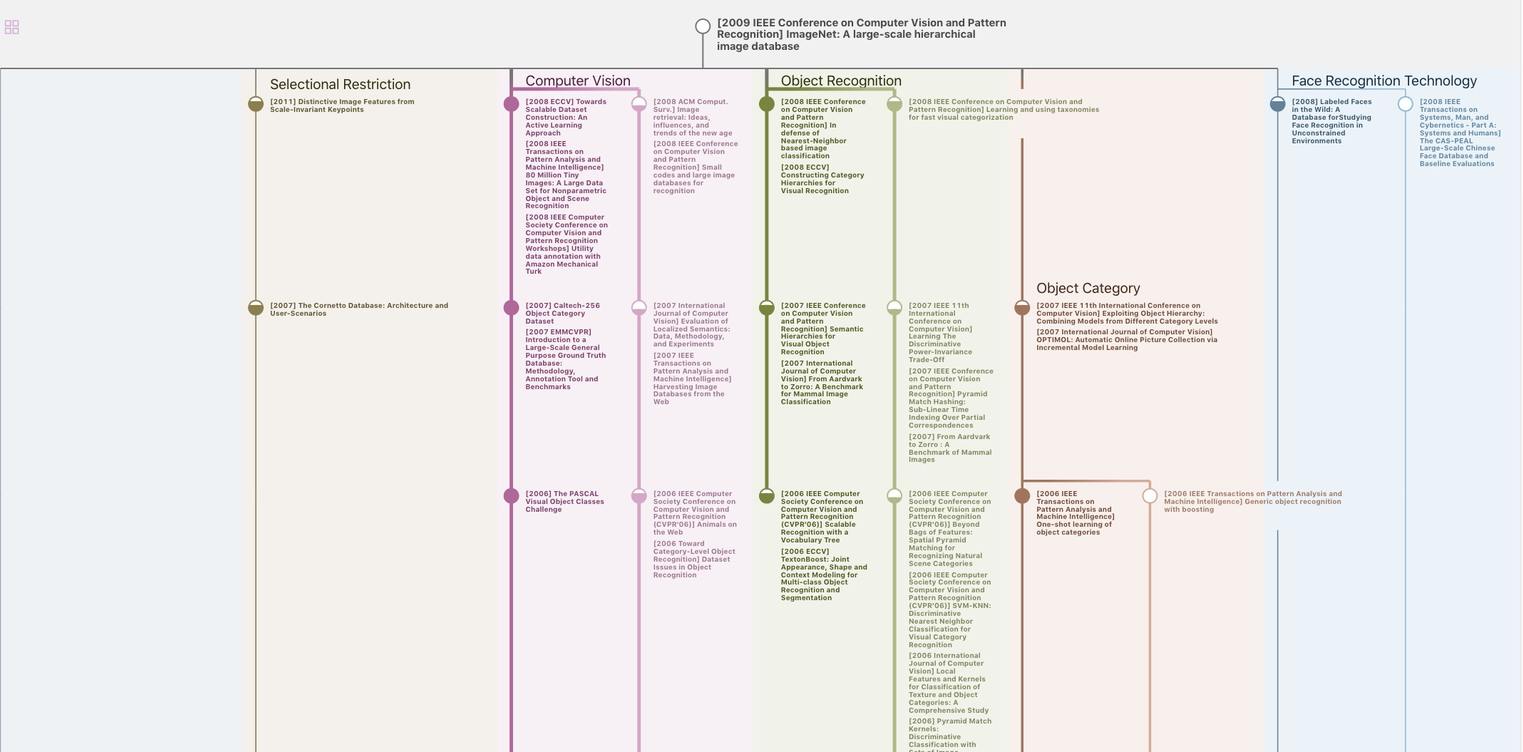
生成溯源树,研究论文发展脉络
Chat Paper
正在生成论文摘要