A Bio-Inspired Spiking Neural Network with Few-Shot Class-Incremental Learning for Gas Recognition
Italian National Conference on Sensors(2023)
摘要
The sensitivity and selectivity profiles of gas sensors are always changed by sensor drifting, sensor aging, and the surroundings (e.g., temperature and humidity changes), which lead to a serious decline in gas recognition accuracy or even invalidation. To address this issue, the practical solution is to retrain the network to maintain performance, leveraging its rapid, incremental online learning capacity. In this paper, we develop a bio-inspired spiking neural network (SNN) to recognize nine types of flammable and toxic gases, which supports few-shot class-incremental learning, and can be retrained quickly with a new gas at a low accuracy cost. Compared with gas recognition approaches such as support vector machine (SVM), k-nearest neighbor (KNN), principal component analysis (PCA) +SVM, PCA+KNN, and artificial neural network (ANN), our network achieves the highest accuracy of 98.75% in five-fold cross-validation for identifying nine types of gases, each with five different concentrations. In particular, the proposed network has a 5.09% higher accuracy than that of other gas recognition algorithms, which validates its robustness and effectiveness for real-life fire scenarios.
更多查看译文
关键词
spiking neural network,few-shot learning,incremental learning,gas recognition
AI 理解论文
溯源树
样例
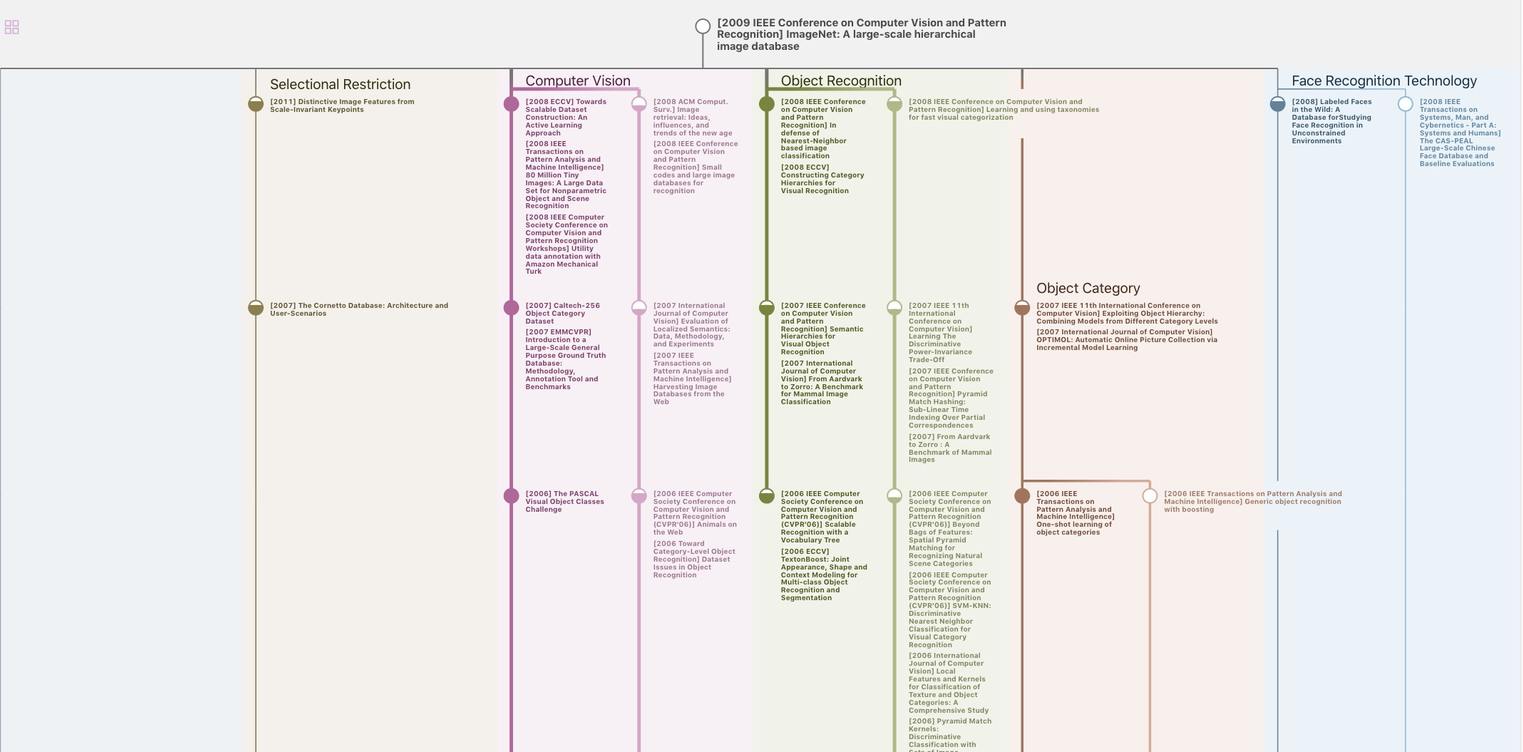
生成溯源树,研究论文发展脉络
Chat Paper
正在生成论文摘要