Pacos: Modeling Users' Interpretable and Context-Dependent Choices in Preference Reversals
arxiv(2023)
摘要
Choice problems refer to selecting the best choices from several items, and learning users' preferences in choice problems is of great significance in understanding the decision making mechanisms and providing personalized services. Existing works typically assume that people evaluate items independently. In practice, however, users' preferences depend on the market in which items are placed, which is known as context effects; and the order of users' preferences for two items may even be reversed, which is referred to preference reversals. In this work, we identify three factors contributing to context effects: users' adaptive weights, the inter-item comparison, and display positions. We propose a context-dependent preference model named Pacos as a unified framework for addressing three factors simultaneously, and consider two design methods including an additive method with high interpretability and an ANN-based method with high accuracy. We study the conditions for preference reversals to occur and provide an theoretical proof of the effectiveness of Pacos in addressing preference reversals. Experimental results show that the proposed method has better performance than prior works in predicting users' choices, and has great interpretability to help understand the cause of preference reversals.
更多查看译文
关键词
preference,context-dependent
AI 理解论文
溯源树
样例
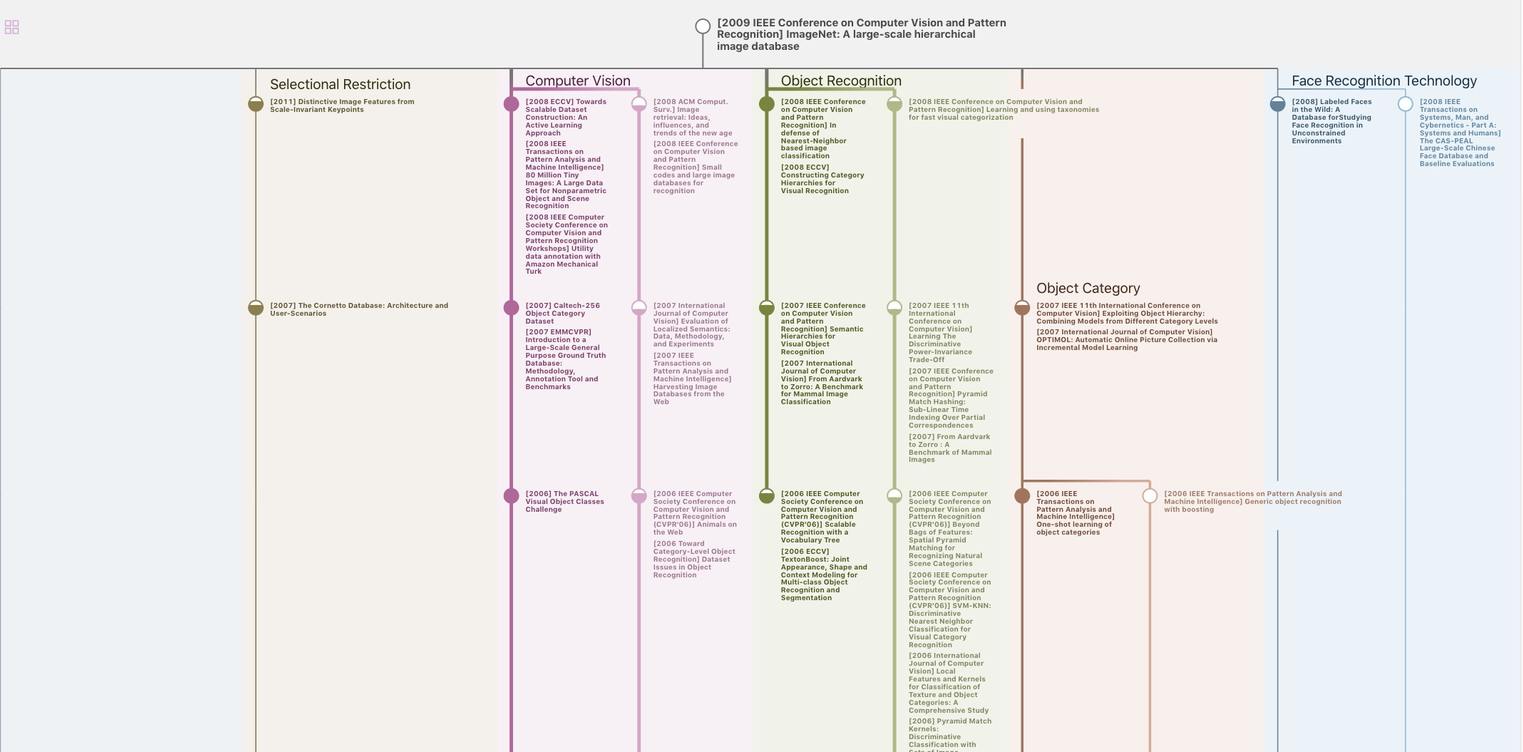
生成溯源树,研究论文发展脉络
Chat Paper
正在生成论文摘要