Generative Model Based Noise Robust Training for Unsupervised Domain Adaptation
ICLR 2023(2023)
摘要
Target domain pseudo-labelling has shown effectiveness in unsupervised domain adaptation (UDA). However, pseudo-labels of unlabeled target domain data are inevitably noisy due to the distribution shift between source and target domains. This paper proposes a Generative model-based Noise-Robust Training method (GeNRT), which eliminates domain shift while mitigating label noise. GeNRT incorporates a Distribution-based Class-wise Feature Augmentation (D-CFA) and a Generative-Discriminative classifier Consistency (GDC), both based on the class-wise target distributions modelled by generative models. D-CFA minimizes the domain gap by augmenting the source data with distribution-sampled target features, and trains a noise-robust discriminative classifier by using target domain knowledge from the generative models. GDC regards all the class-wise generative models as generative classifiers and enforces a consistency regularization between the generative and discriminative classifiers. It exploits an ensemble of target knowledge from all the generative models to train a noise-robust discriminative classifier and eventually gets theoretically linked to the Ben-David domain adaptation theorem for reducing the domain gap. Extensive experiments on Office-Home, PACS, and Digit-Five show that our GeNRT achieves comparable performance to state-of-the-art methods under single-source and multi-source UDA settings.
更多查看译文
关键词
Unsupervised Domain Adaptation,Generative Models,Feature Augmentation,Generative and Discriminative Consistency
AI 理解论文
溯源树
样例
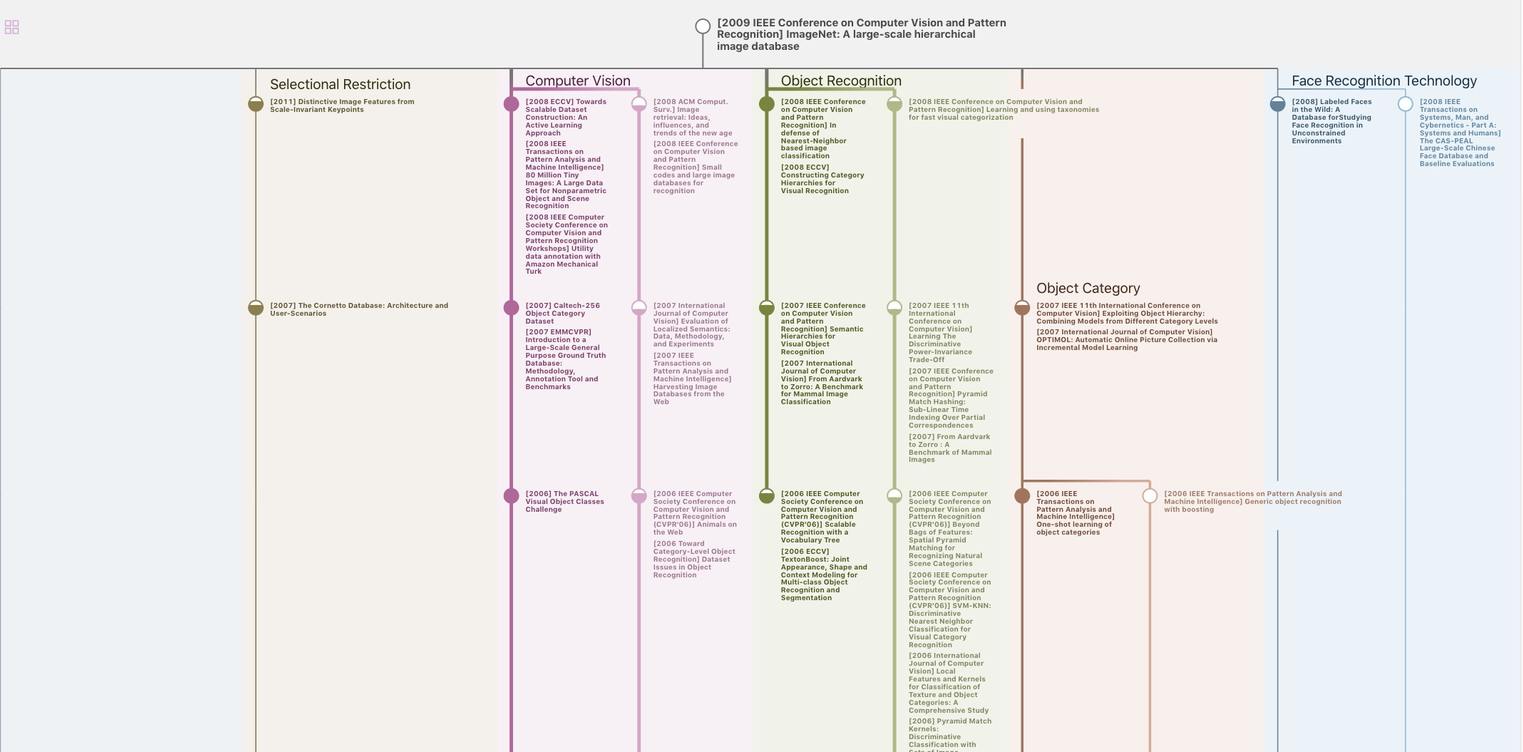
生成溯源树,研究论文发展脉络
Chat Paper
正在生成论文摘要