A pseudo-likelihood approach to community detection in weighted networks
arXiv (Cornell University)(2023)
Abstract
Community structure is common in many real networks, with nodes clustered in groups sharing the same connections patterns. While many community detection methods have been developed for networks with binary edges, few of them are applicable to networks with weighted edges, which are common in practice. We propose a pseudo-likelihood community estimation algorithm derived under the weighted stochastic block model for networks with normally distributed edge weights, extending the pseudo-likelihood algorithm for binary networks, which offers some of the best combinations of accuracy and computational efficiency. We prove that the estimates obtained by the proposed method are consistent under the assumption of homogeneous networks, a weighted analogue of the planted partition model, and show that they work well in practice for both homogeneous and heterogeneous networks. We illustrate the method on simulated networks and on a fMRI dataset, where edge weights represent connectivity between brain regions and are expected to be close to normal in distribution by construction.
MoreTranslated text
Key words
community detection,networks,pseudo-likelihood
AI Read Science
Must-Reading Tree
Example
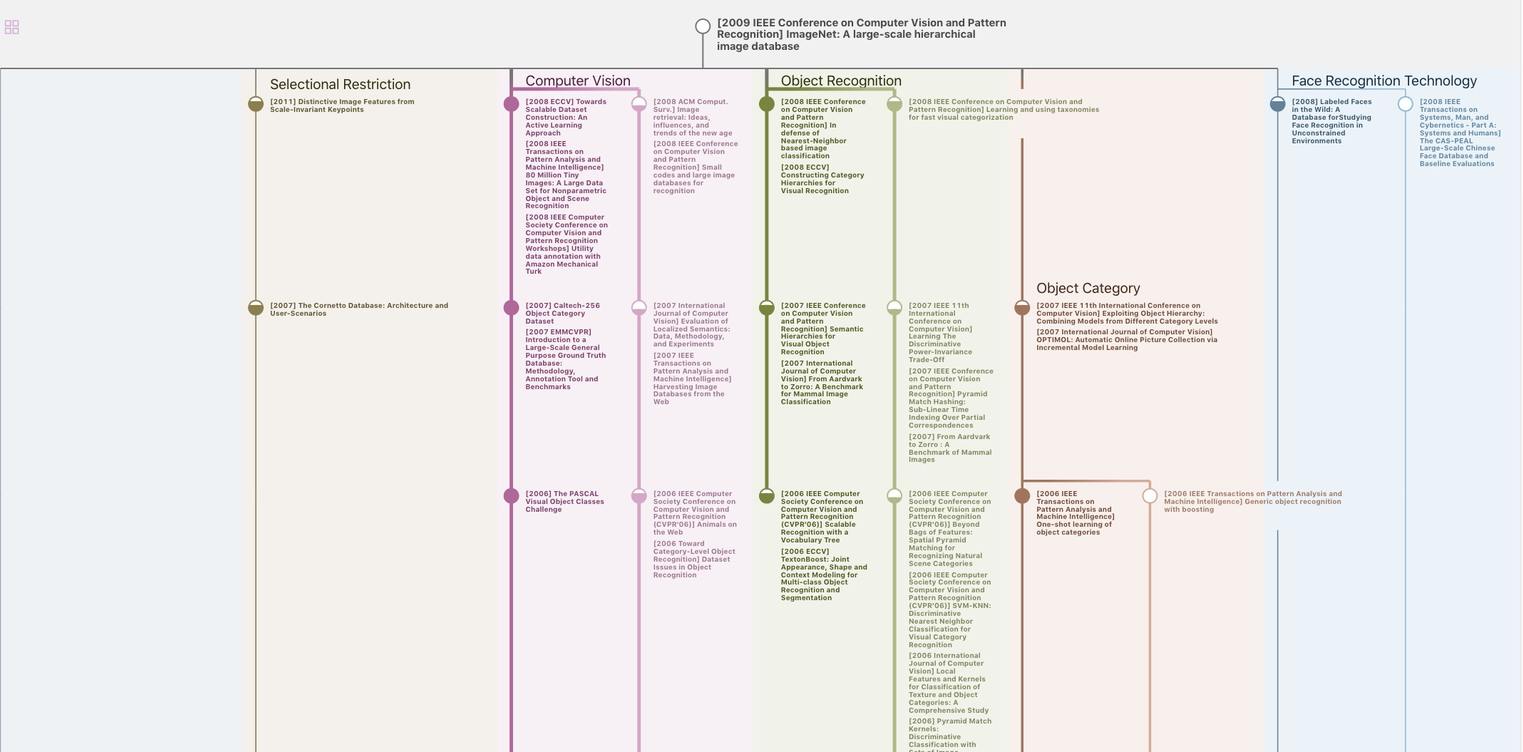
Generate MRT to find the research sequence of this paper
Chat Paper
Summary is being generated by the instructions you defined